Domain Knowledge-Based Neural Network Architecture for End-to-End Multiuser Precoding in Massive MIMO System
VTC2023-Spring(2023)
摘要
This paper investigates an end-to-end multiuser precoding in massive multiple-input multiple-output (MIMO) system. We propose a novel neural network architecture which exploits an appropriate human expert knowledge in wireless communication domain. The employed domain knowledge addresses the inter-user interference. In particular, the concept of a leakage is adopted to decouple the sum-rate maximization problem by transforming the input to the decoder neural network (NN) at base station (BS). In contrast with the existing NN architecture, the proposed architecture is proven to be scalable to the number of user equipments (UEs) as the common NN model (parameter set) can be employed for the different numbers of UEs. Numerical results demonstrate that the proposed scalable decoder NN architecture can learn the distribution of the interuser interference effectively.
更多查看译文
关键词
Massive multiple-input multiple-output (MIMO),multiuser precoding,deep learning,limited feedback,neural network (NN),frequency-division duplex (FDD),domain knowledge,leakage,inter-user interference,scalable,downlink
AI 理解论文
溯源树
样例
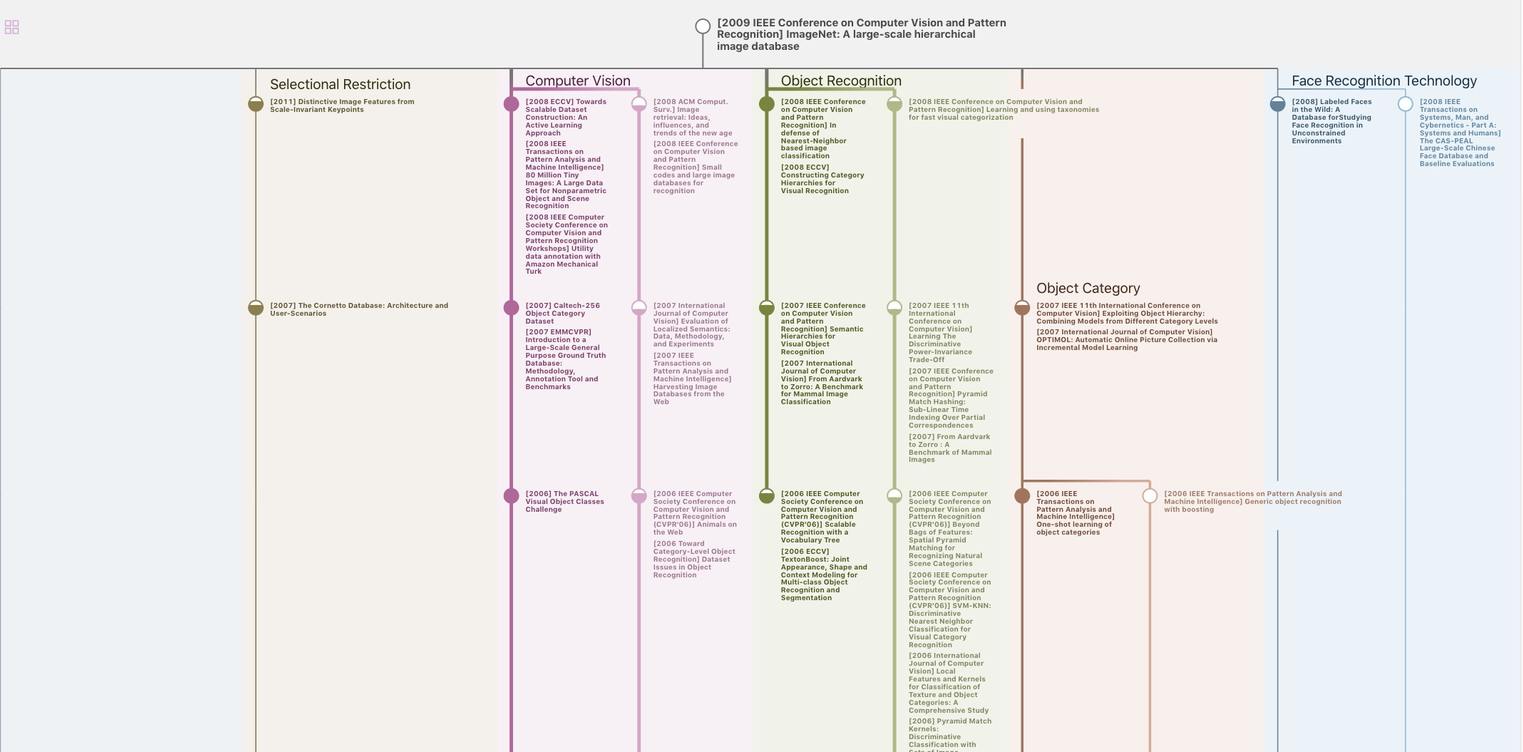
生成溯源树,研究论文发展脉络
Chat Paper
正在生成论文摘要