Stochastic Graph Neural Network-Based Value Decomposition for Multi-Agent Reinforcement Learning in Urban Traffic Control
2023 IEEE 97TH VEHICULAR TECHNOLOGY CONFERENCE, VTC2023-SPRING(2023)
摘要
Multi-Agent Reinforcement Learning (MARL) has reached astonishing achievements in various fields such as the traffic control of vehicles in a wireless connected environment. In MARL, how to effectively decompose a global feedback into the relative contributions of individual agents belongs to one of the most fundamental problems. However, the volatility of the environment (e.g., the vehicle movement and wireless disturbance) could significantly shape the time-varying topological relationships among agents, thus making the Value Decomposition (VD) challenging. Therefore, in order to cope with this annoying volatility, it becomes imperative to design a dynamic VD framework. Hence, in this paper, we propose a novel Stochastic VMIX (SVMIX) methodology by embedding the dynamic topological features into the VD and incorporating the corresponding components into a multi-agent actor-critic architecture. In particular, the Stochastic Graph Neural Network (SGNN) is leveraged to effectively extract underlying dynamics embedded in topological features and improve the flexibility of VD against the environment volatility. Finally, the superiority of SVMIX is verified through extensive simulations.
更多查看译文
关键词
Urban traffic control,multi-agent reinforcement learning,value decomposition,stochastic graph neural network
AI 理解论文
溯源树
样例
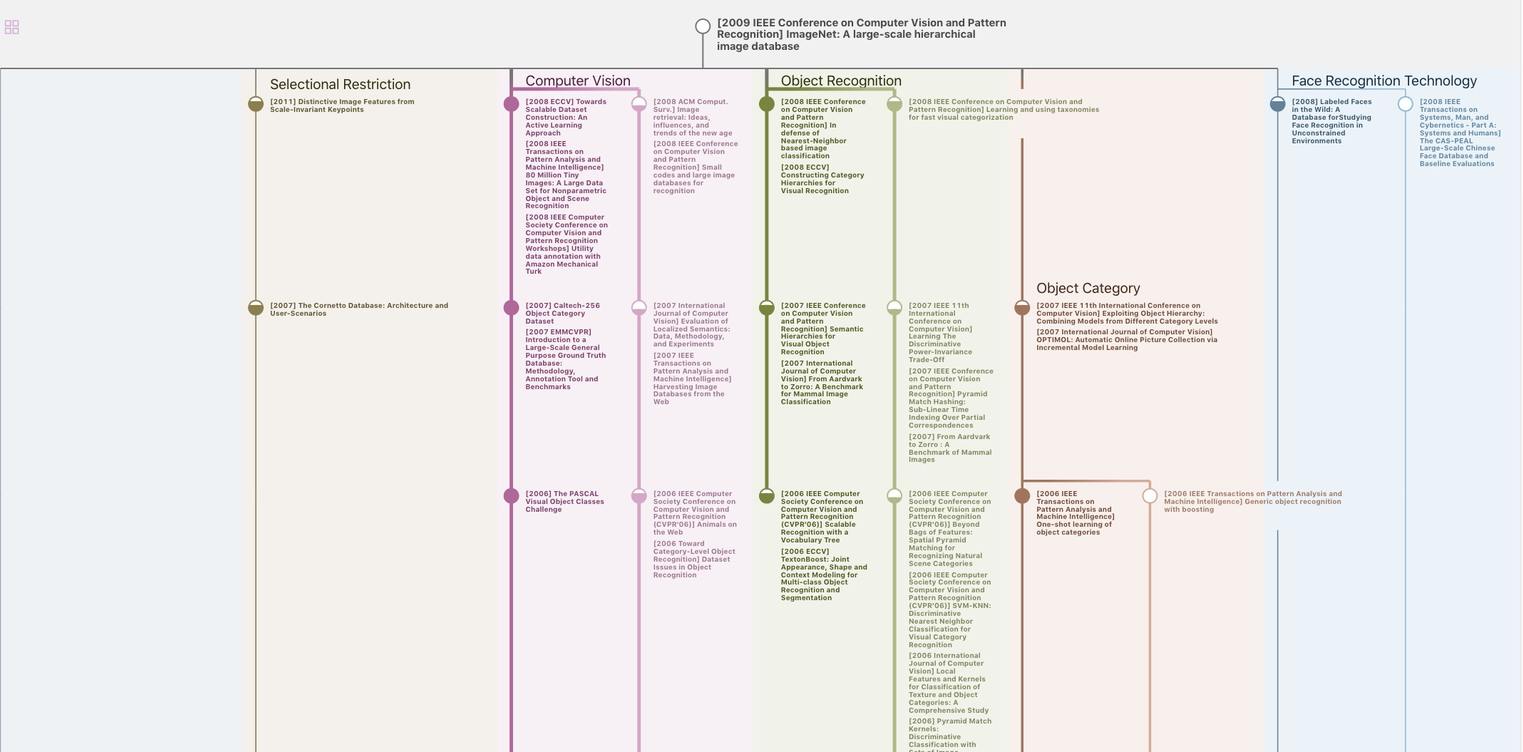
生成溯源树,研究论文发展脉络
Chat Paper
正在生成论文摘要