Hierarchical Spatio-Temporal Neural Network with Displacement Based Refinement for Monocular Head Pose Prediction
2023 18TH INTERNATIONAL CONFERENCE ON MACHINE VISION AND APPLICATIONS, MVA(2023)
摘要
Head pose prediction aims to forecast future head pose given observed sequence, which plays an increasingly important role in human computer interaction, virtual reality, and driver monitoring. However, since there are many moving possibilities, current head pose works, mainly focusing on estimation, fail to provide sufficient temporal information to meet the high demands for accurate predictions. This paper proposes (A) a Spatio-Temporal Encoder (STE), (B) a displacement based offset generating module, and (C) a time step feature aggregation module. The STE extracts spatial information via Transformer and temporal information according to the time order of frames. The displacement based offset generating module utilizes displacement information through a frequency domain process between adjacent frames to generate an offset to refine the prediction result. Furthermore, the time step feature aggregation module integrates time step features based on the information density and hierarchically extracts past motion information as prior knowledge to capture the motion recurrence. Extensive experiments have shown that the proposed network outperforms related methods, achieving a Mean Absolute Error (MAE) of 4.5865 degrees on simple background sequences and 7.1325 degrees on complex background sequences.
更多查看译文
AI 理解论文
溯源树
样例
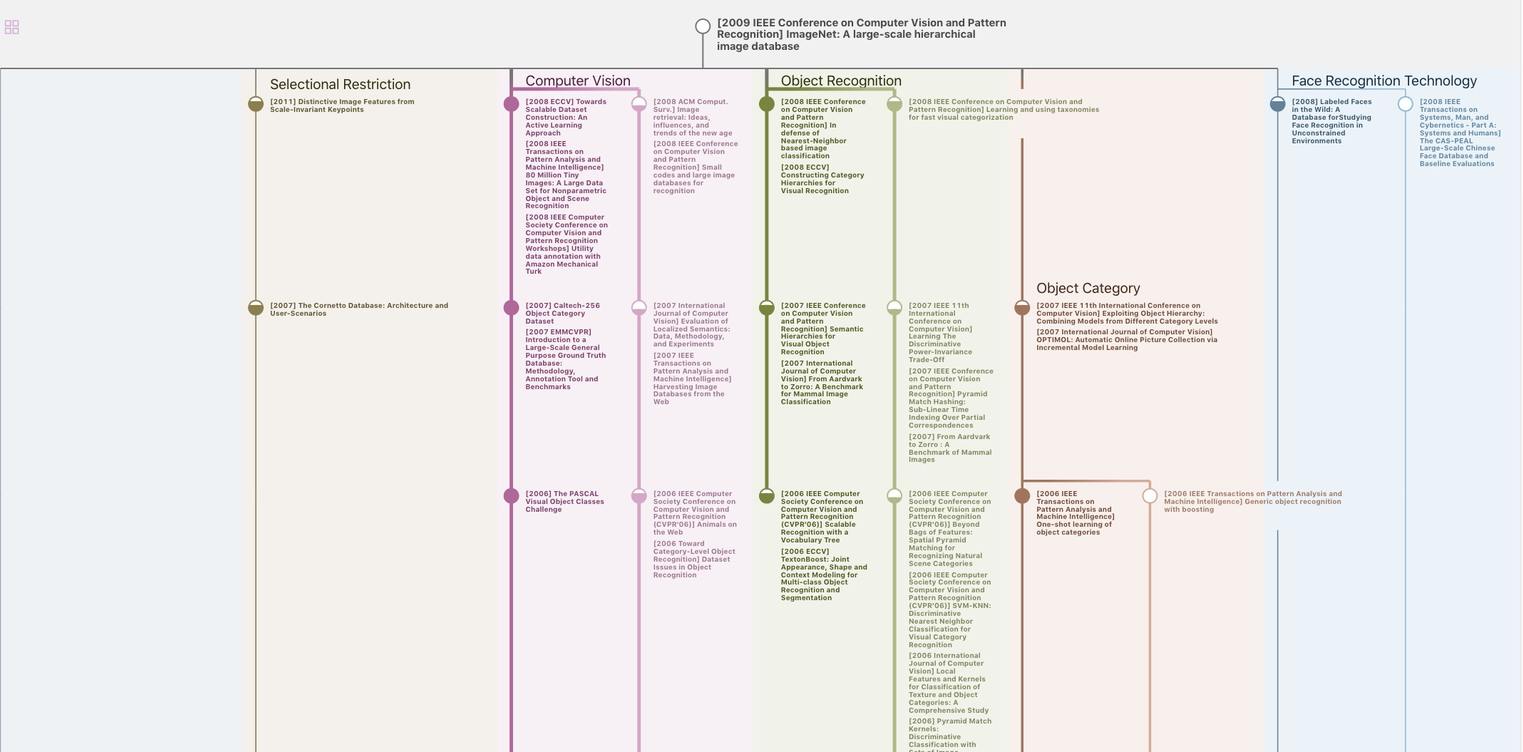
生成溯源树,研究论文发展脉络
Chat Paper
正在生成论文摘要