Multi-Agent Deep Reinforcement Learning for Task Offloading in Vehicle Edge Computing.
BMSB(2023)
摘要
With the rapid update of the Internet of Things (IoT) in this recent period, Various equipment applications that seem to be latency savvy have emerged. Offloading data using traditional methods requires waiting until the device is in range of the MEC server for transmission. This significantly increases timing overhead and often fails to meet the latency demands of certain implementations. The high construction cost makes it impractical to deploy several MEC servers to provide complete road coverage. As a model for vehicle edge computing (VEC) task offloading utilizing a Deep Reinforcement Learning (DRL) based technique, this paper suggests a multi-vehicle aided MEC system. The capacity of vehicles' computer resources is constrained, which may limit their ability to complete duties on time. The work can be sent to the roadside units (RSU) VEC server, which has more powerful processing capabilities. We present the Actor-Critic based DRL method to advance the model's training efficiency in order to improve convergence efficiency and obtain superior system performance. Simulation findings demonstrate that our proposed Actor-Critic based DRL strategy may significantly outperform the traditional DQN technique in terms of performance, convergence speed, and total operating expenses.
更多查看译文
关键词
Vehicle Edge Computing (VEC),Deep Reinforcement Learning (DRL),Task Offloading,Actor-Critic,Internet of vehicle (IoV)
AI 理解论文
溯源树
样例
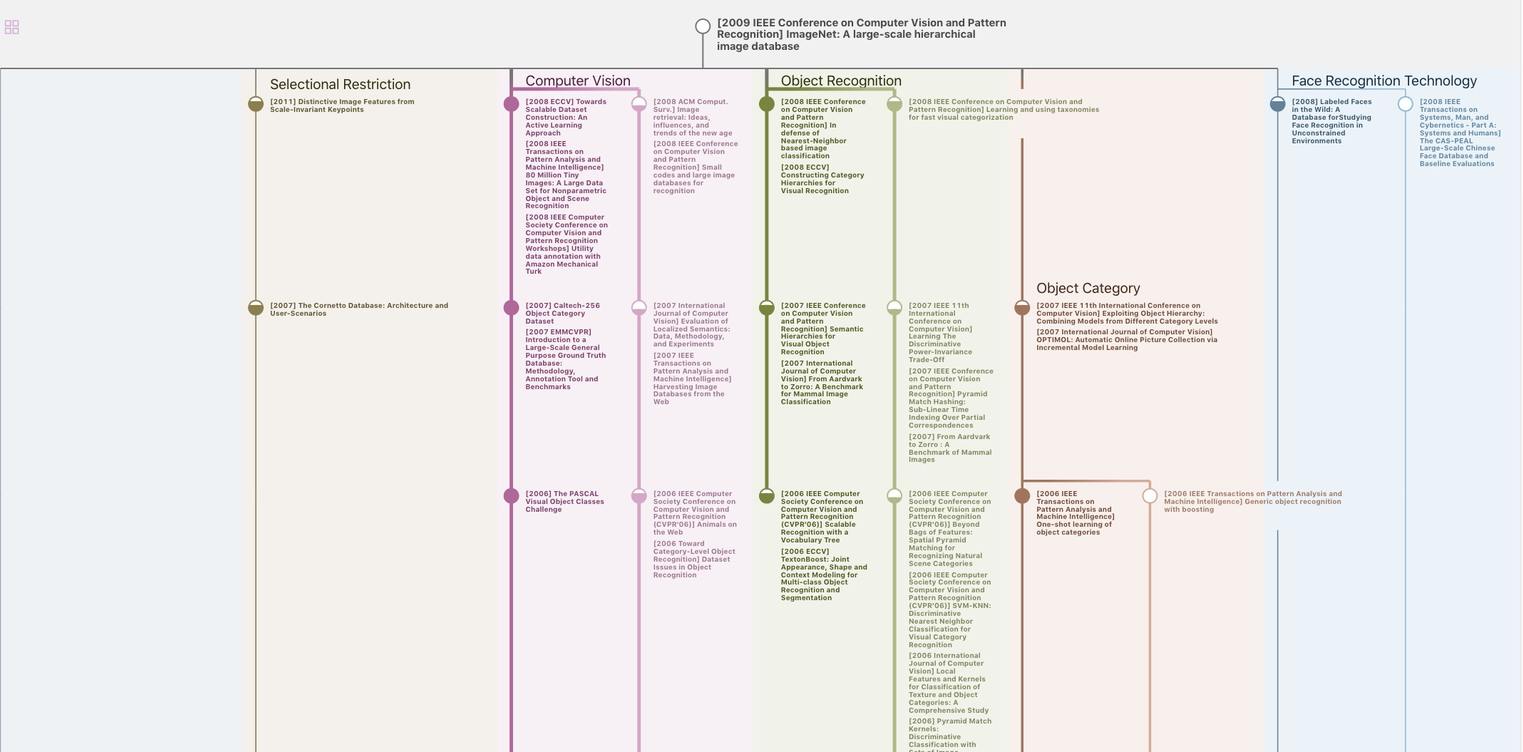
生成溯源树,研究论文发展脉络
Chat Paper
正在生成论文摘要