A policy gradient approach for optimization of smooth risk measures.
UAI(2023)
摘要
We propose policy gradient algorithms for solving a risk-sensitive reinforcement learning (RL) problem in on-policy as well as off-policy settings. We consider episodic Markov decision processes, and model the risk using the broad class of smooth risk measures of the cumulative discounted reward. We propose two template policy gradient algorithms that optimize a smooth risk measure in on-policy and off-policy RL settings, respectively. We derive non-asymptotic bounds that quantify the rate of convergence of our proposed algorithms to a stationary point of the smooth risk measure. As special cases, we establish that our algorithms apply to optimization of mean-variance and distortion risk measures, respectively.
更多查看译文
关键词
policy gradient approach,risk,optimization
AI 理解论文
溯源树
样例
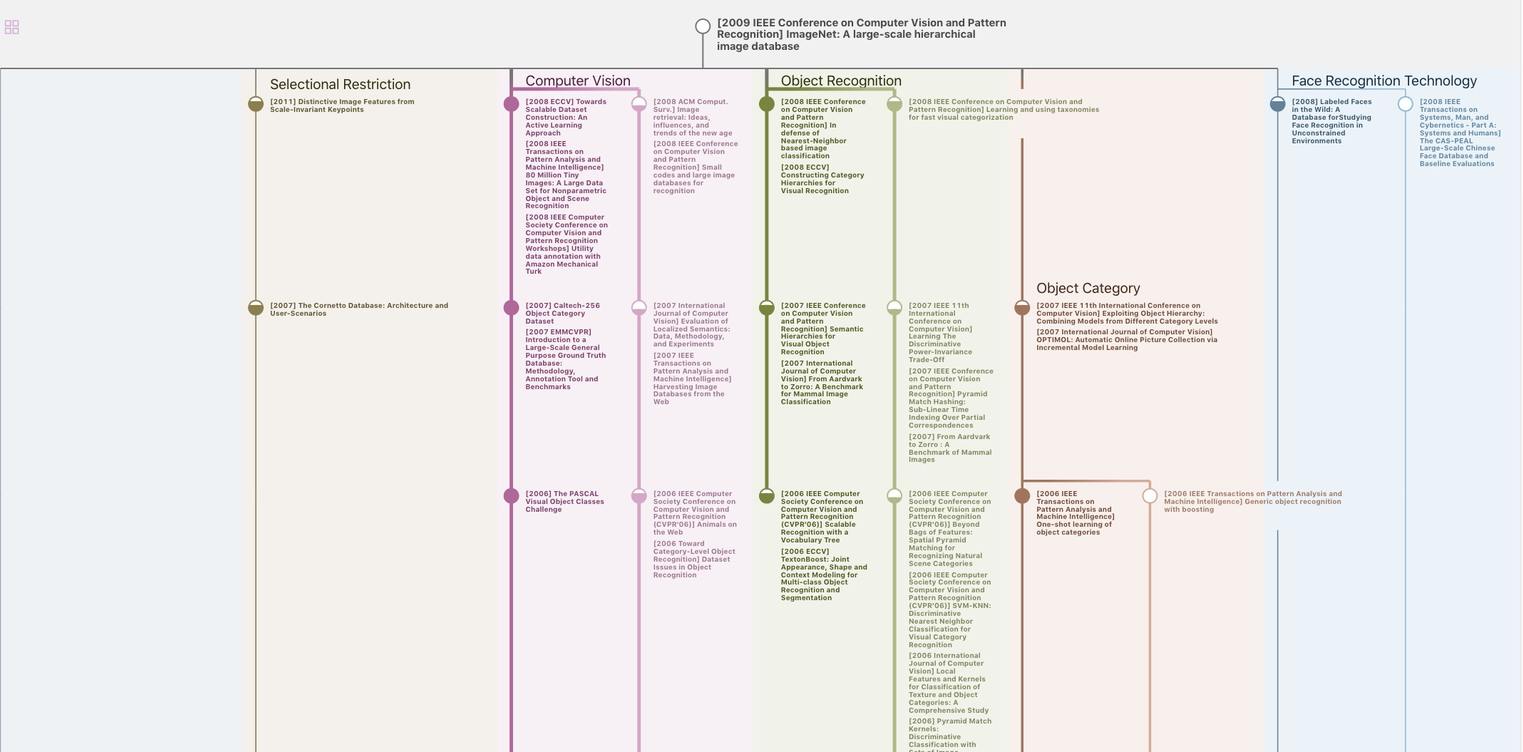
生成溯源树,研究论文发展脉络
Chat Paper
正在生成论文摘要