Resource Allocation Using Deep Reinforcement Learning in GEO Multibeam Satellite System
BMSB(2023)
摘要
Future Internet of Things (IoT) is becoming more and more common in multi-beam satellite communications. However, due to the constrained system resource, the next generation of Geostationary Earth Orbit (GEO) multibeam satellite systems will focus on achieving flexible joint power and bandwidth allocation. Therefore, a GEO multi-beam satellite resource optimization scheme is proposed in this paper. We first model the optimization problem as a Markov decision process (MDP) due to the continuity and variation of the system resource. Then we propose a deep reinforcement learning (DRL) algorithm based on Asynchronous Advantage Actor-critic (A3C) to jointly allocate power and bandwidth whose goal is to satisfy the beam traffic demand. The algorithm aims to increase the system throughput and user fairness. The simulation results demonstrate that our proposed algorithm achieves significant advantages over the existing algorithms.
更多查看译文
关键词
Multibeam GEO Satellite,power allocation,bandwidth allocation,full frequency reuse,deep reinforcement learning
AI 理解论文
溯源树
样例
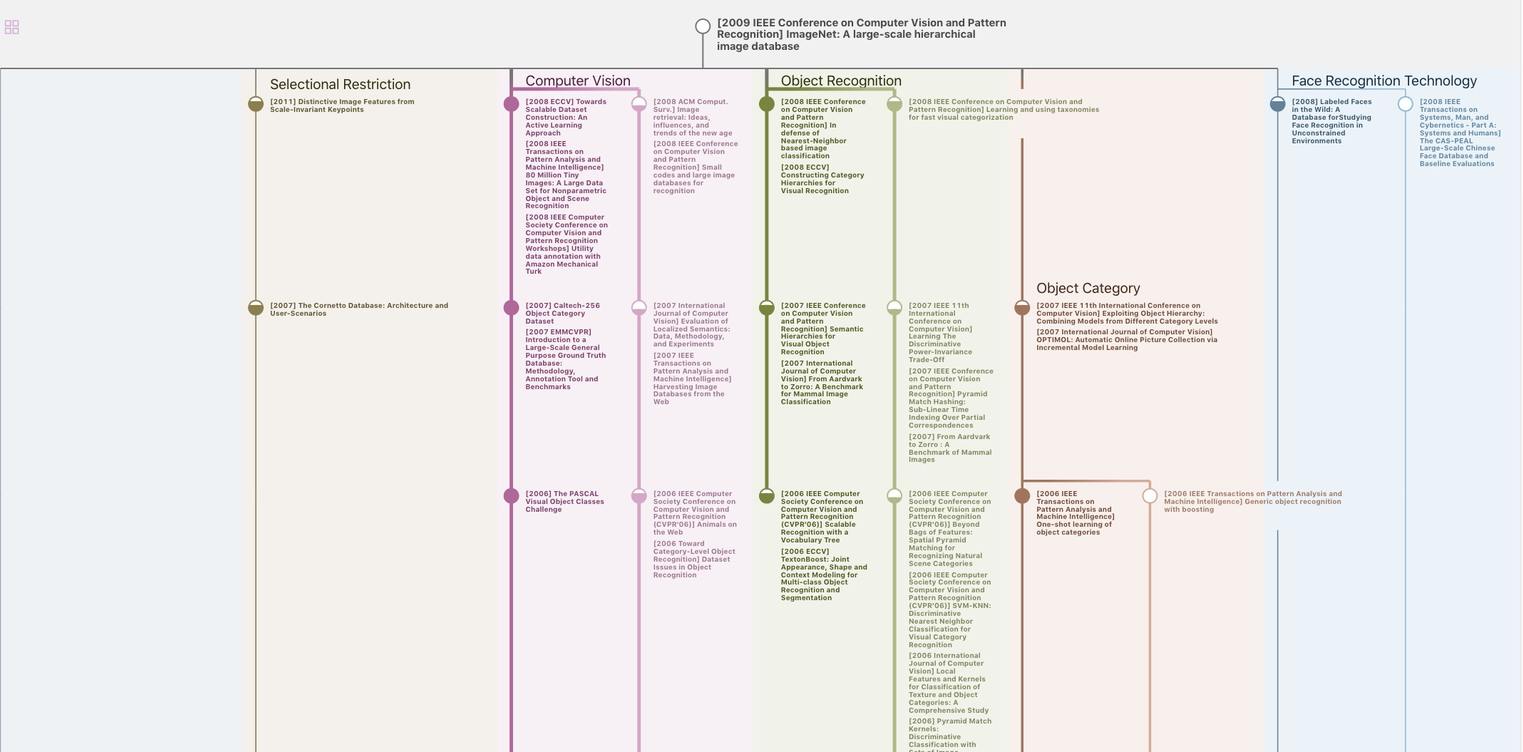
生成溯源树,研究论文发展脉络
Chat Paper
正在生成论文摘要