Convolutional Neural Networks for Gas Turbine Exhaust Gas Temperature and Power Predictions.
ICPHM(2023)
摘要
In this work, a data-driven and deep learning-based predictive modeling framework has been developed for generating accurate prediction models intended for gas turbine engine performance analysis. This paper focuses on the application of Convolutional Neural Networks (CNNs) along with tabular data to image conversion techniques to predict exhaust gas temperature (EGT) and power outputs of Gas Turbine Engines (GTE). Using one such tabular data to image conversion method called Image Generator for Tabular Data (IGTD), several CNN model architectures were explored, and their predictive capabilities were compared. The effectiveness of the proposed predictive modeling framework which combines CNNs and the IGTD algorithm has been demonstrated for EGT and power prediction using GTE operational data collected over a period of three years. The CNN models using images converted from tabular data exhibit superior predictive capabilities for both EGT and power, with a more significant improvement observed for EGT prediction. To the best of our knowledge, this is the first attempt to apply IGTD based CNNs for developing GTE models for EGT and power prediction.
更多查看译文
关键词
Gas turbine engine,convolutional neural networks,deep learning,regression models,image generator for tabular data
AI 理解论文
溯源树
样例
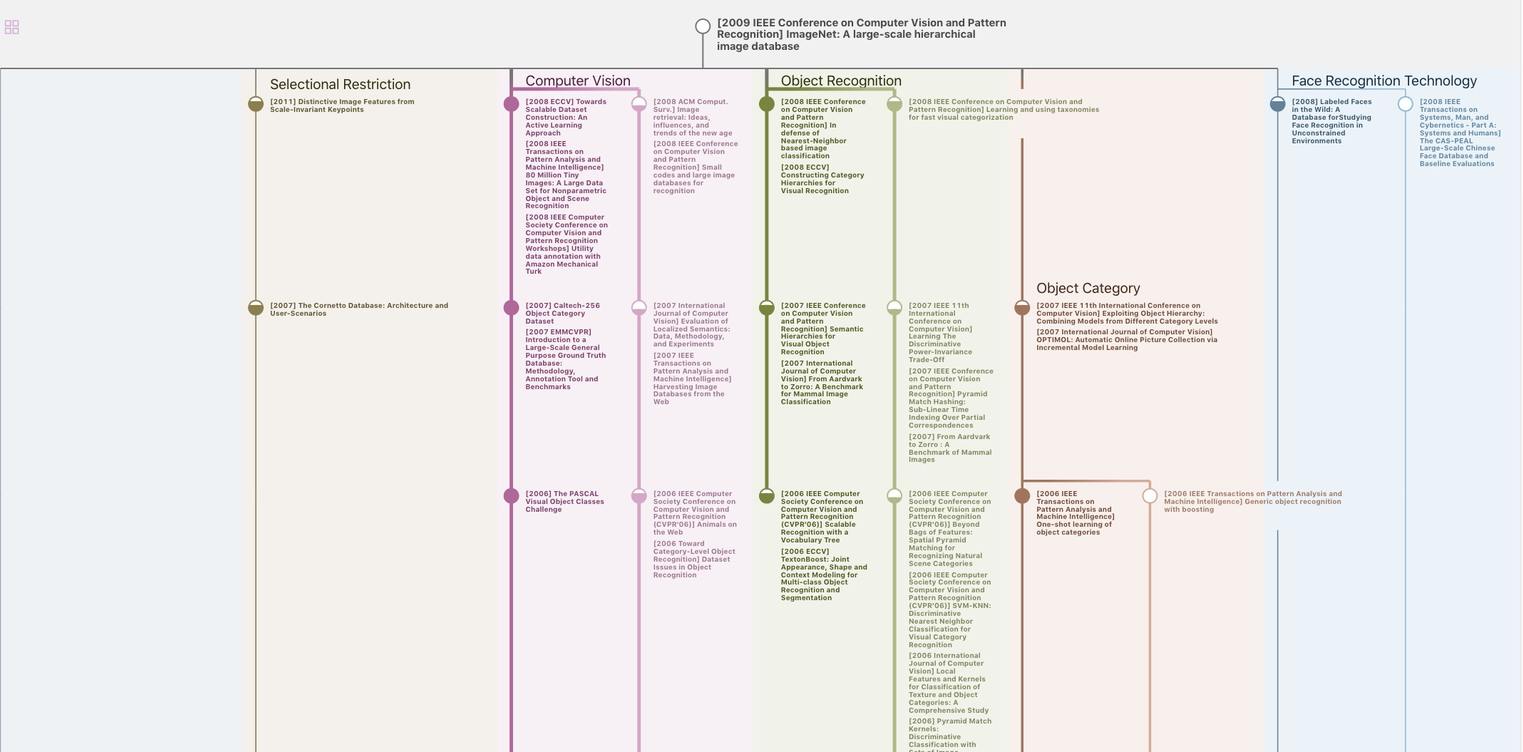
生成溯源树,研究论文发展脉络
Chat Paper
正在生成论文摘要