Automatic Gaussian Bandwidth Selection for Kernel Principal Component Analysis.
KSEM (1)(2023)
摘要
Kernel principal component analysis (KPCA) has been proved to be useful in many applications as a dimension reduction technique. Compared with PCA, KPCA better exploits the complicated spatial structure of high-dimensional features. Because of the high computational cost of exact KPCA, however, research has focused on the fast computation of KPCA, through techniques such as matrix sketching, the Nyström method, and random Fourier features. There has been little discussion of the selection of kernel bandwidth, which is critical in practice. In this paper, we propose a new data-driven bandwidth selection method, called the criterion of the maximum sum of eigenvalues (CMSE) method and a scalable variation (SCMSE) to handle big data. Both feature high time efficiency and achieve performance better than or comparable to that of the existing methods. We conduct both simulation study and real-world data analyses to support our conclusions.
更多查看译文
关键词
automatic gaussian bandwidth selection,kernel principal component analysis
AI 理解论文
溯源树
样例
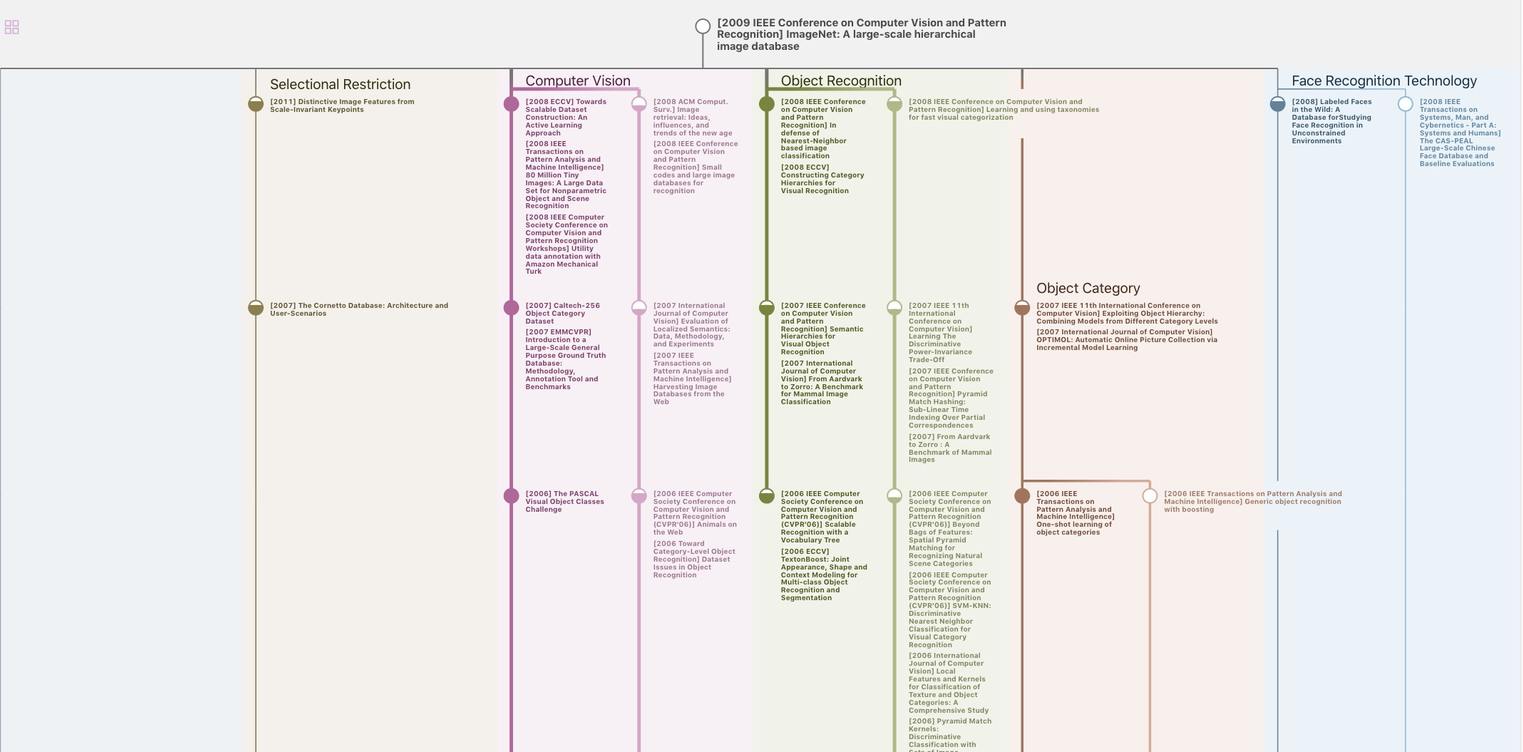
生成溯源树,研究论文发展脉络
Chat Paper
正在生成论文摘要