DNN-Based Calibration Factor Estimation for Effective SINR Mapping in CQI Selection of 5G NR.
BMSB(2023)
摘要
This paper proposes a deep neural network (DNN)-based calibration factor estimation for an effective signal-to-interference-plus-noise ratio (SINR) mapping in a channel quality indicator (CQI) selection of fifth-generation new radio (5G NR). In 5G NR, a receiver can report CQI to a transmitter, and adaptive modulation and coding (AMC) is performed in the transmitter. The CQI can be selected using an effective SINR mapping (ESM) scheme in the receiver. The ESM scheme can compress the received SINRs into an effective SINR. Further, a calibration factor is required to improve the accuracy of ESM. The optimal value of the calibration factor may differ according to the channel environment between the transmitter and receiver. In this paper, the proposed scheme can estimate the optimal calibration factor using the DNN model. Simulation results show that the proposed DNN-based scheme outperforms the conventional scheme using the predefined calibration factor.
更多查看译文
关键词
Calibration factor,channel quality indicator,deep neural network,effective signal-to-interference-plus-noise ratio
AI 理解论文
溯源树
样例
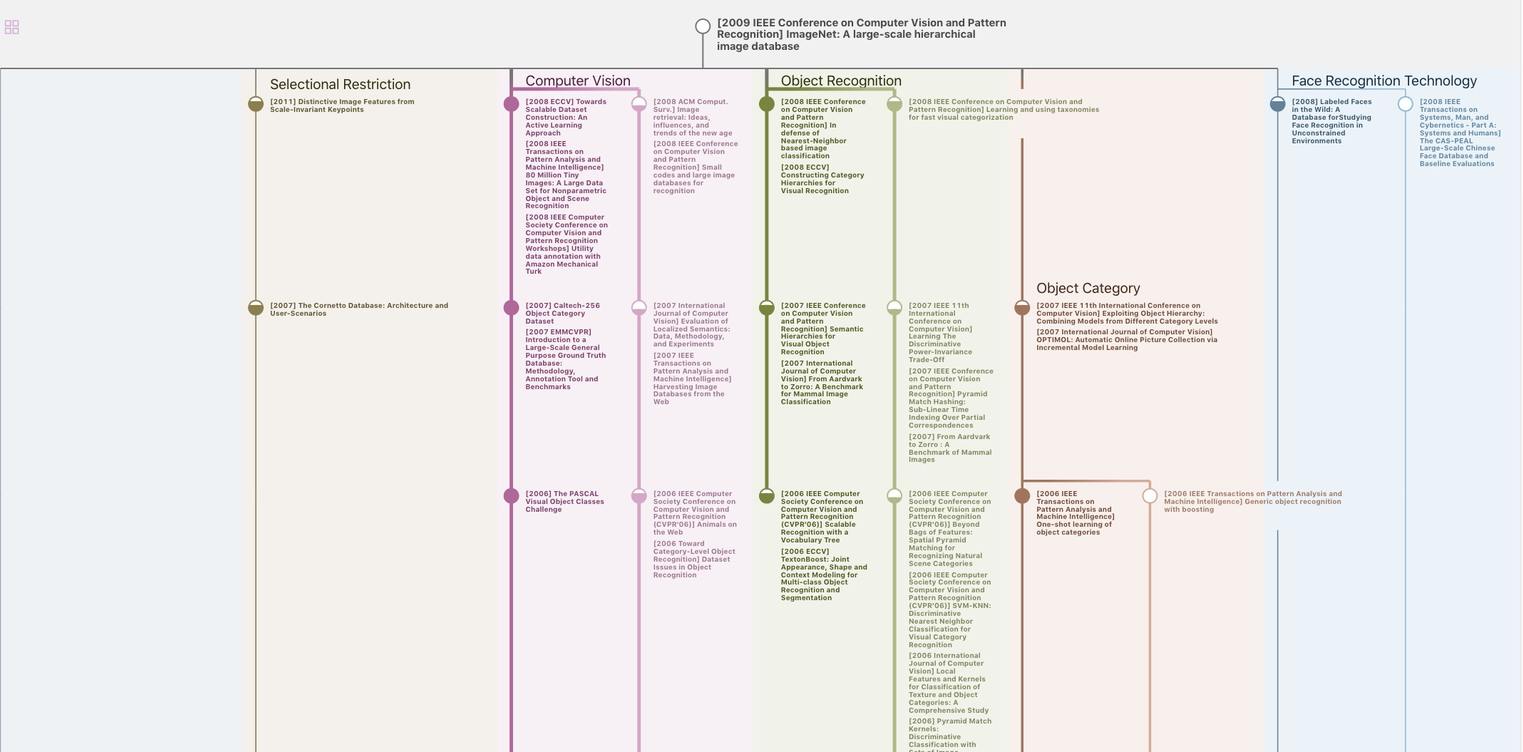
生成溯源树,研究论文发展脉络
Chat Paper
正在生成论文摘要