Classification Error in Semi-Supervised Fuzzy C-Means.
EUSFLAT/AGOP(2023)
摘要
Semi-Supervised Fuzzy C-Means (SSFCMeans) model enables inclusion of additional knowledge about the true class of a part of the training data. With this partial supervision, there comes a new possibility to use this model as a classifier. The main goal should be thus to minimize the classification error, just as in the fully supervised setting. However, the typical problems with minimizing the training error, test error, and avoiding the phenomenon of overfitting must be carefully considered with respect to the characteristics of the SSFCMeans model. In this work, we fill the identified research gap and analyze the way of handling partial supervision in Semi-Supervised Fuzzy C-Means and its impact on the aforementioned issues. We investigate this relationship experimentally using artificially simulated data. We show that the training error for the training phase is directly related to the scaling factor $$\alpha $$ and is deterministically assured to be equal to 0 in some cases. We further illustrate our main findings for real-life partially labeled data collected from smartphones of patients with bipolar disorder in a problem of predicting the phase of the disease.
更多查看译文
关键词
classification,error,semi-supervised,c-means
AI 理解论文
溯源树
样例
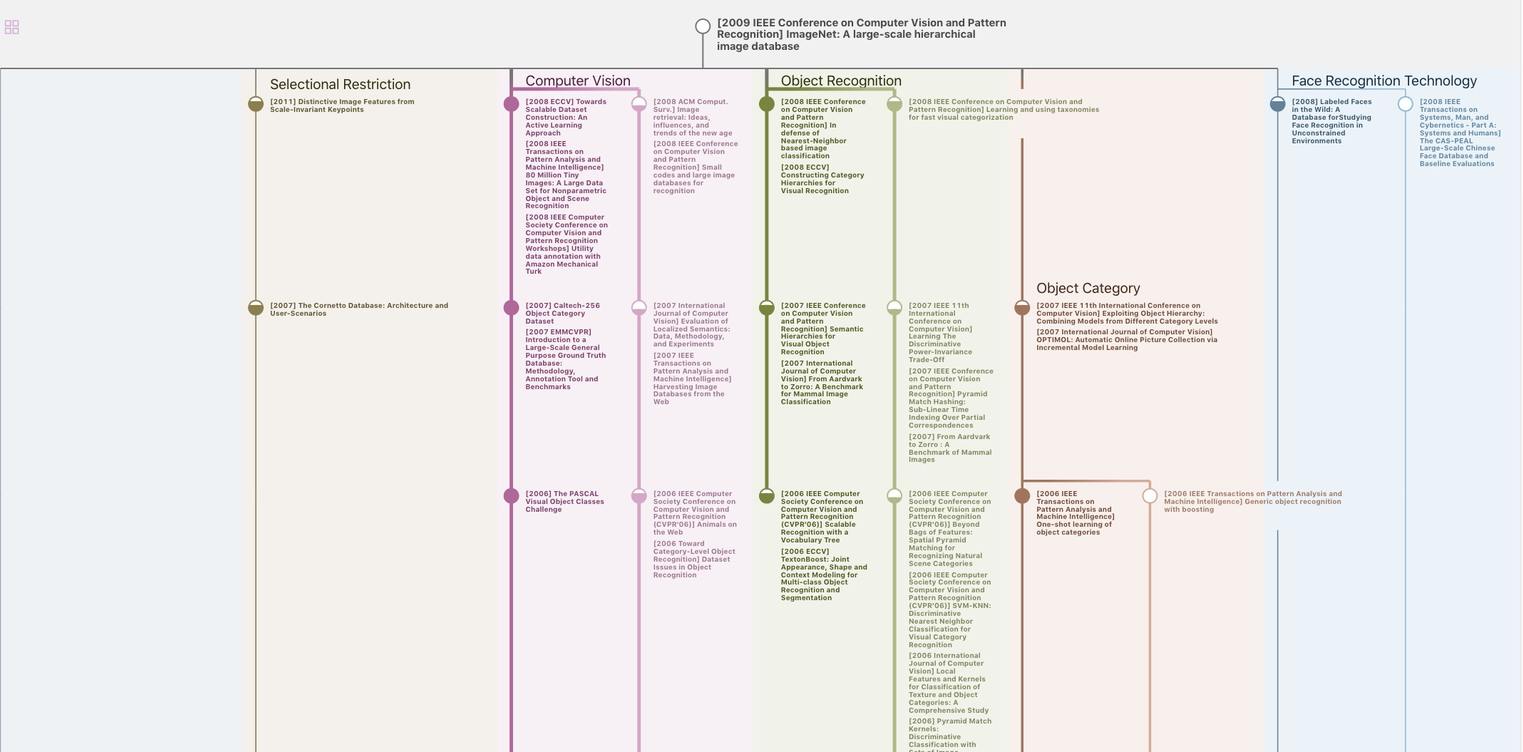
生成溯源树,研究论文发展脉络
Chat Paper
正在生成论文摘要