On the Privacy of Social Networks with Personal Privacy Choices.
ISIT(2023)
摘要
We consider the problem of designing privacy mechanisms for users whose data is correlated based on a Markov random field over a graph, with social networks being a concrete application. Each user, modeled as a node in a graph, has its own personal data and can choose their privacy settings to be: ON or OFF, indicating whether the node requires privacy or not. We suppose that the users’ data is to be shared with a third party, such as electoral or ad campaigns, while respecting the different personal privacy choices of the users.The notion of privacy we use is a variation of differential privacy called dependent differential privacy that can handle the correlated nature of the data. The goal is to preserve the required privacy by releasing a noisy version of each user’s data while minimizing the expected error. We focus on the class of one-hop mechanisms in which each node’s released data depends on its own and its neighbors’ data. This class of mechanisms leads to scalable algorithms that are easily parallelizable. We present One-Hop Algorithm and show that it outputs the privatized data while respecting the privacy settings of each user in the presence of correlation. To give more insight, we consider two examples, star and complete graphs, and compare the privacy-utility tradeoffs of different versions of our algorithm.
更多查看译文
关键词
dependent differential privacy,error minimization,graph node,Markov random field,One-Hop Algorithm,personal data,privacy mechanisms,social networks
AI 理解论文
溯源树
样例
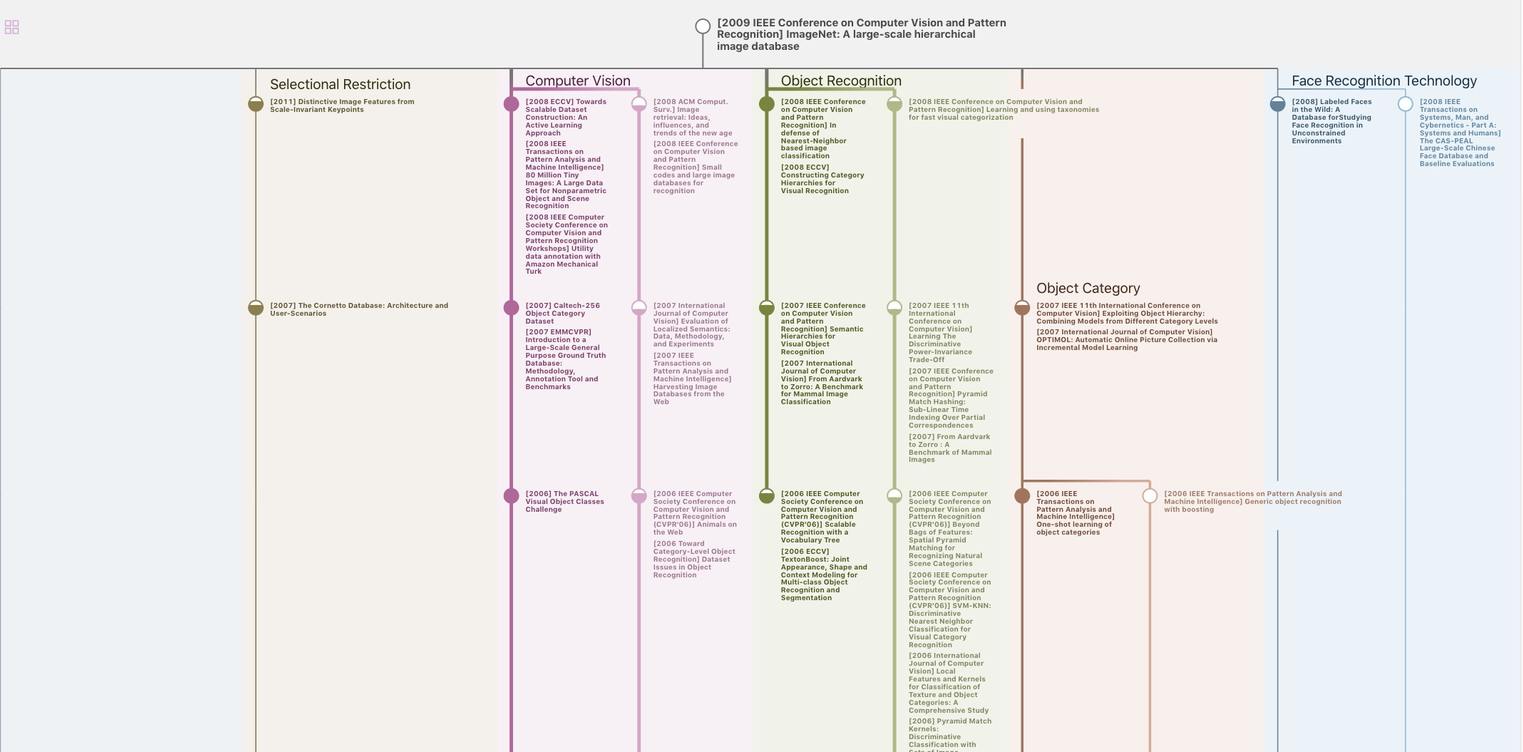
生成溯源树,研究论文发展脉络
Chat Paper
正在生成论文摘要