An Information Theoretic Approach for Collaborative Distributed Parameter Estimation.
ISIT(2023)
摘要
In many federated learning scenarios, the distributed nodes represent and exchange information in the form of functions or statistics of data, and the computation and communication are often restricted by the dimensionality of the functions. In this paper, we explore the collaborative distributed parameter estimation under such constraints. Specifically, we assume that each node can observe a sequence of i.i.d. sampled data and communicate some statistics of the observed data with dimensionality constraints. We characterize the Cramer-Rao lower bound (CRLB) and construct the asymptotic efficient estimator that achieves CRLB. In addition, we provide the information geometric interpretation of the CRLB as projecting the score function onto the functional subspaces spanned by the distributed nodes. Finally, we present the neural estimator to compute the optimal statistics that the nodes shall transmit to each other for continuous variables.
更多查看译文
关键词
asymptotic efficient estimator,collaborative distributed parameter estimation,Cramer-Rao lower bound,CRLB,dimensionality constraints,federated learning,functional subspaces,information geometric interpretation,information theoretic approach,neural estimator,score function
AI 理解论文
溯源树
样例
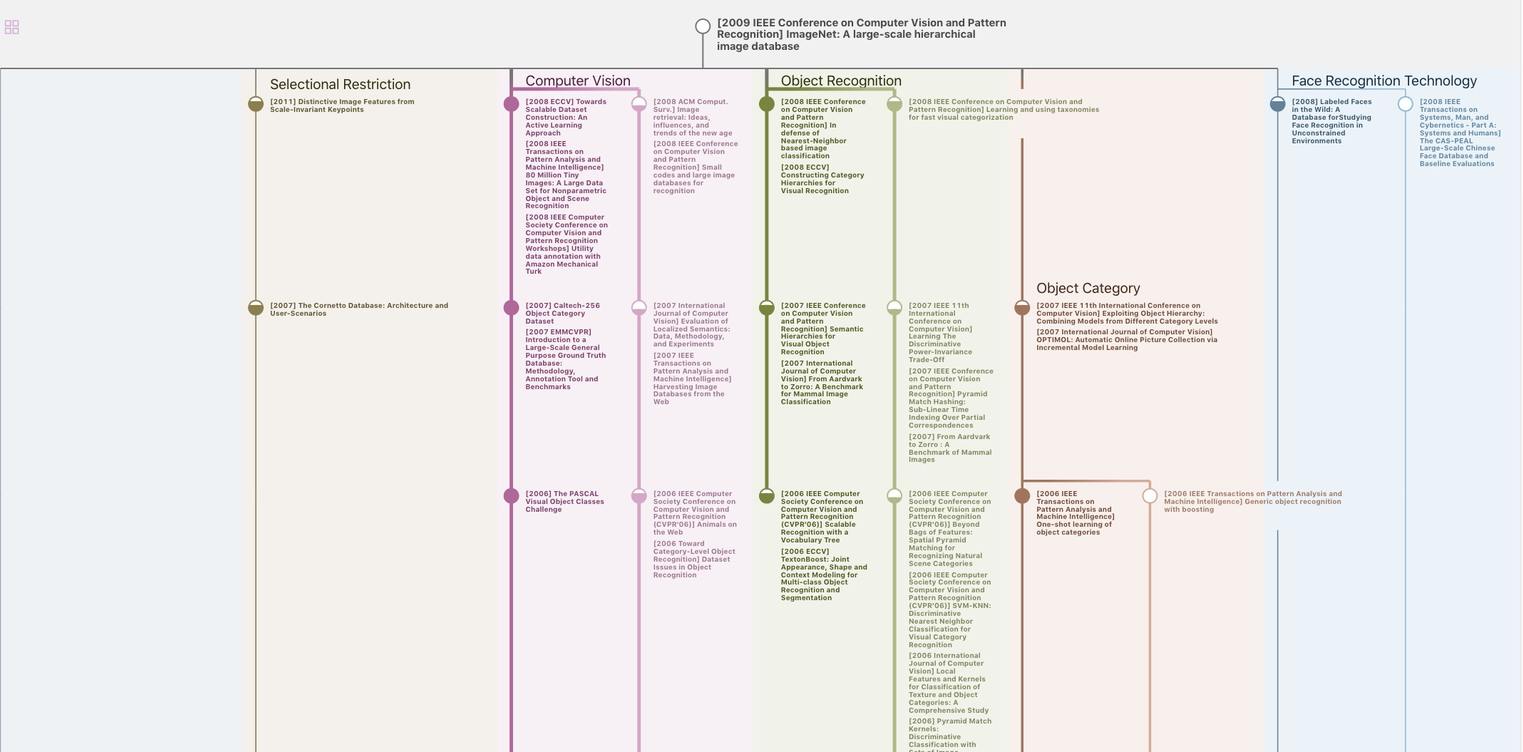
生成溯源树,研究论文发展脉络
Chat Paper
正在生成论文摘要