Uncertainty-Guided Active Reinforcement Learning with Bayesian Neural Networks
2023 IEEE INTERNATIONAL CONFERENCE ON ROBOTICS AND AUTOMATION, ICRA(2023)
摘要
Recent advances in Reinforcement Learning (RL) have made significant contributions in past years by offering intelligent solutions to solve robotic tasks. However, most RL algorithms, especially the model-free RL, are plagued by low learning efficiency and safety problems. In this paper, we propose using the Bayesian Neural Networks (BNNs) to guide the agent exploring actively to enhance the learning efficiency in RL and investigate the potential of recognizing safety risks in working environments with uncertainty information. We compare two types of uncertainty quantification methods in both action and state spaces. To validate our method, we visualize the quantified uncertainty in robot environments with or without safety hazards. Moreover, we evaluate the learning efficiency and safety performance of the RL agents learned with BNNs on different robotic tasks.
更多查看译文
AI 理解论文
溯源树
样例
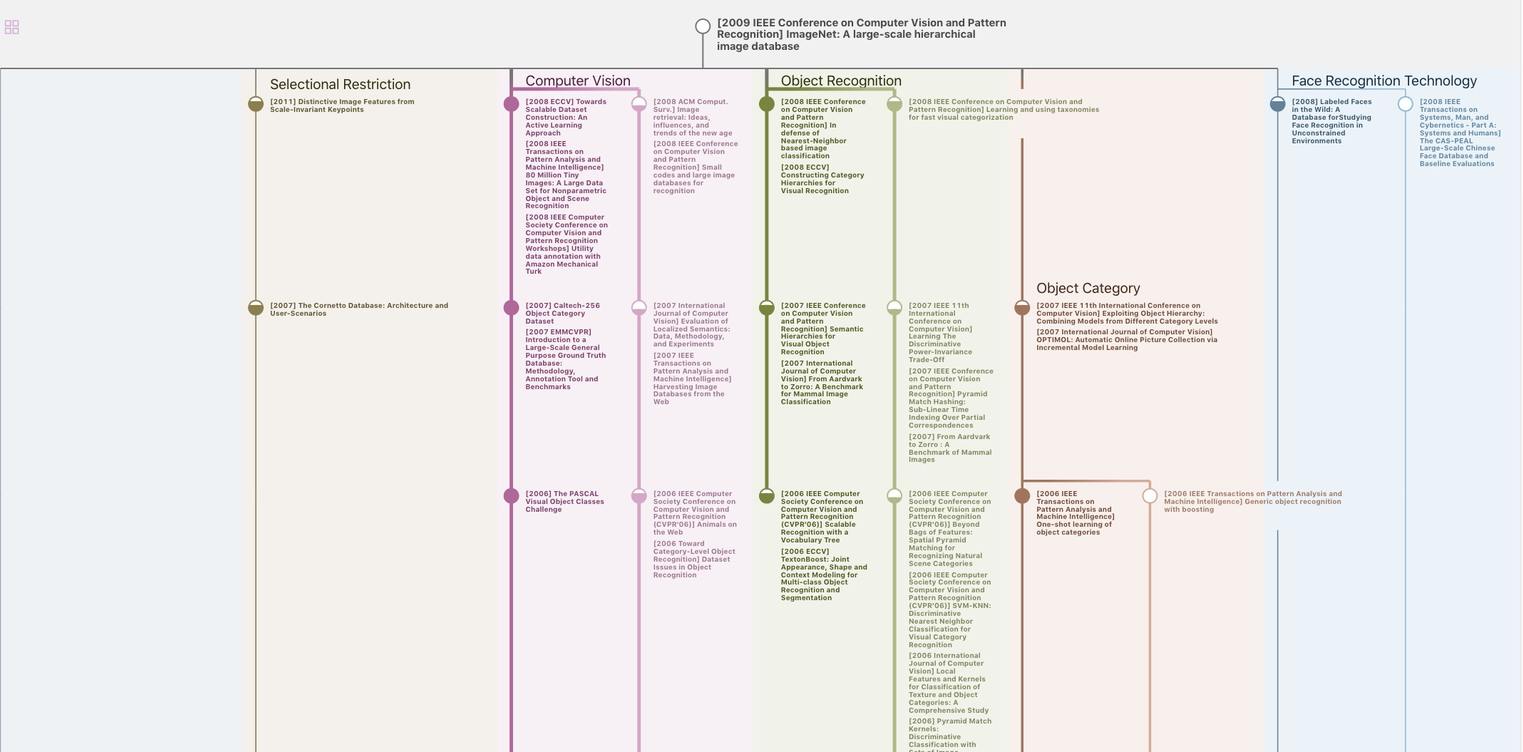
生成溯源树,研究论文发展脉络
Chat Paper
正在生成论文摘要