A Stochastic Rate-Distortion Approach to Supervised Learning Systems.
ISIT(2023)
摘要
Machine learning applications have exploded in recent years due to the availability of huge data sets as well as advances in computational and storage capabilities. Although successful methods have been proposed to reduce learning system complexity while maintaining required accuracy levels, theoretical understanding of the underlying trade-offs remains elusive. In this paper, the classical supervised learning problem is reformulated within a rate-distortion framework. It provides insights into crucial accuracy-complexity trade-offs, by considering the overall learning system as consisting of two components. The first is tasked with extracting (learning) from the source the minimal number of information bits necessary to ultimately achieve the prescribed output accuracy. The learned bits are then used to retrieve the desired output from the second component, an appropriately designed codebook. The premise here is that an optimal system is characterized by having to learn the minimum amount of information from the source, just sufficient to yield the system output at the desired precision, which implies efficiency in terms of system complexity, generalization and training data requirements. The design and training of such a reformulated system is detailed in this paper, and asymptotically optimal performance achieving the rate-distortion bound is established.
更多查看译文
关键词
accuracy levels,classical supervised learning problem,computational storage capabilities,crucial accuracy-complexity trade-offs,huge data sets,information bits,learning system complexity,machine learning applications,optimal system,prescribed output accuracy,rate-distortion framework,reformulated system,stochastic rate-distortion approach,supervised learning systems,system output,training data requirements
AI 理解论文
溯源树
样例
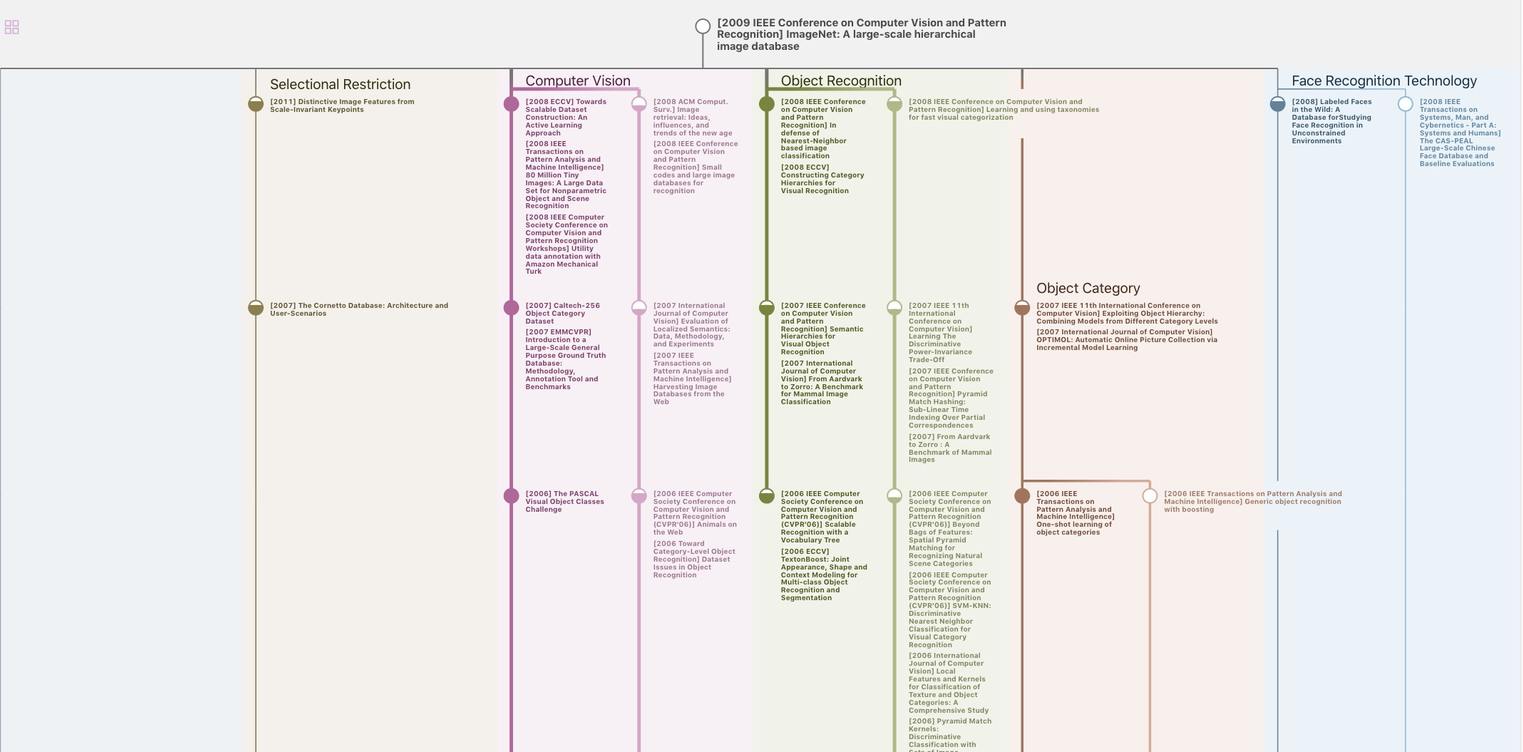
生成溯源树,研究论文发展脉络
Chat Paper
正在生成论文摘要