Entry-Specific Bounds for Low-Rank Matrix Completion under Highly Non-Uniform Sampling
ISIT(2024)
摘要
Low-rank matrix completion concerns the problem of estimating unobserved
entries in a matrix using a sparse set of observed entries. We consider the
non-uniform setting where the observed entries are sampled with highly varying
probabilities, potentially with different asymptotic scalings. We show that
under structured sampling probabilities, it is often better and sometimes
optimal to run estimation algorithms on a smaller submatrix rather than the
entire matrix. In particular, we prove error upper bounds customized to each
entry, which match the minimax lower bounds under certain conditions. Our
bounds characterize the hardness of estimating each entry as a function of the
localized sampling probabilities. We provide numerical experiments that confirm
our theoretical findings.
更多查看译文
关键词
asymptotic scalings,entry-specific bounds,error upper bounds,estimation algorithms,hardness,low-rank matrix completion,minimax lower bounds,sampling probabilities
AI 理解论文
溯源树
样例
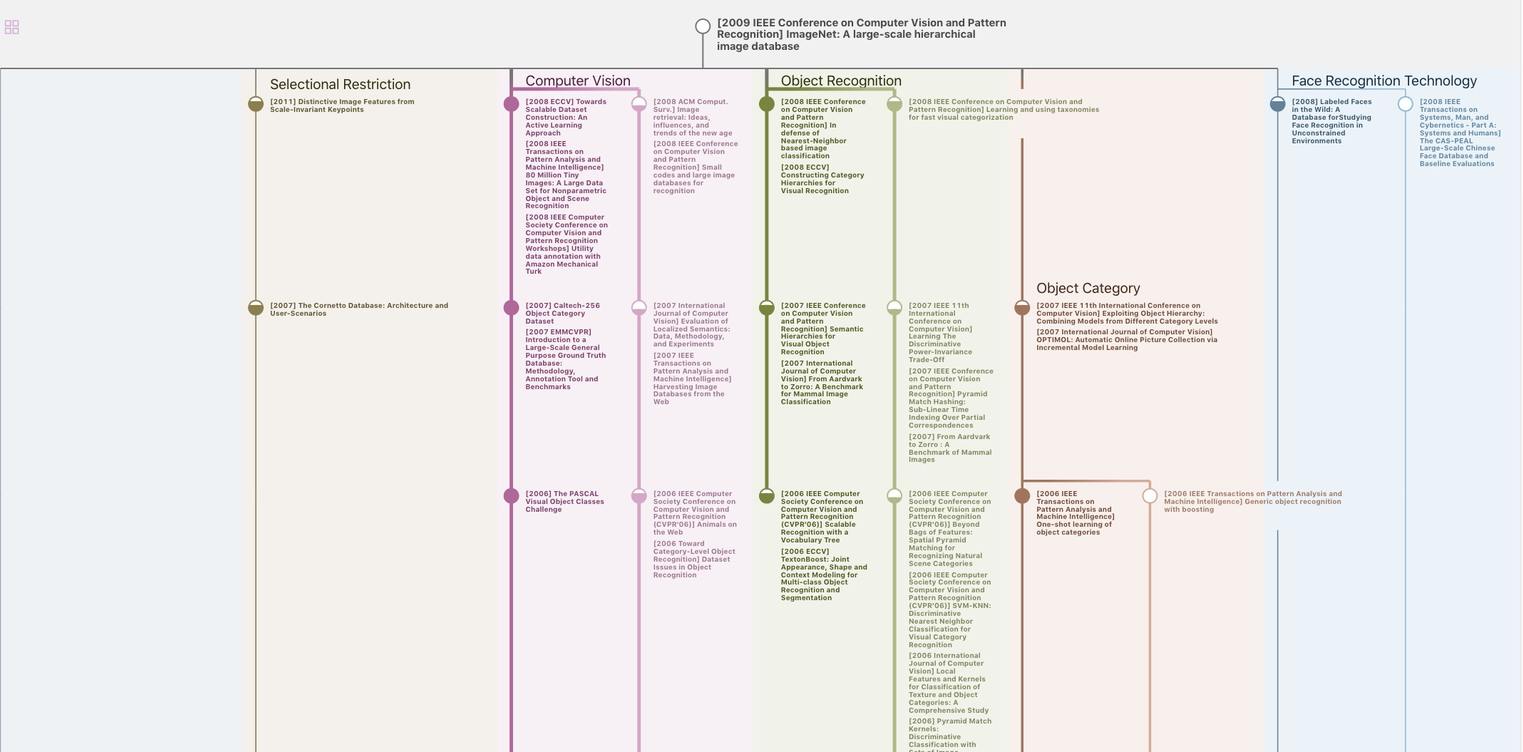
生成溯源树,研究论文发展脉络
Chat Paper
正在生成论文摘要