Interpretable and Flexible Target-Conditioned Neural Planners For Autonomous Vehicles.
ICRA(2023)
摘要
Learning-based approaches to autonomous vehicle planners have the potential to scale to many complicated real-world driving scenarios by leveraging huge amounts of driver demonstrations. However, prior work only learns to estimate a single planning trajectory, while there may be multiple acceptable plans in real-world scenarios. To solve the problem, we propose an interpretable neural planner to regress a heatmap, which effectively represents multiple potential goals in the bird's-eye view of an autonomous vehicle. The planner employs an adaptive Gaussian kernel and relaxed hourglass loss to better capture the uncertainty of planning problems. We also use a negative Gaussian kernel to add supervision to the heatmap regression, enabling the model to learn collision avoidance effectively. Our systematic evaluation on the Lyft Open Dataset across a diverse range of real-world driving scenarios shows that our model achieves a safer and more flexible driving performance than prior works.
更多查看译文
关键词
adaptive Gaussian kernel,autonomous vehicle planners,bird,driver demonstrations,heatmap regression,interpretable neural planner,more flexible driving performance,multiple acceptable plans,multiple potential goals,negative Gaussian kernel,planning problems,real-world driving scenarios,safer driving performance,single planning trajectory
AI 理解论文
溯源树
样例
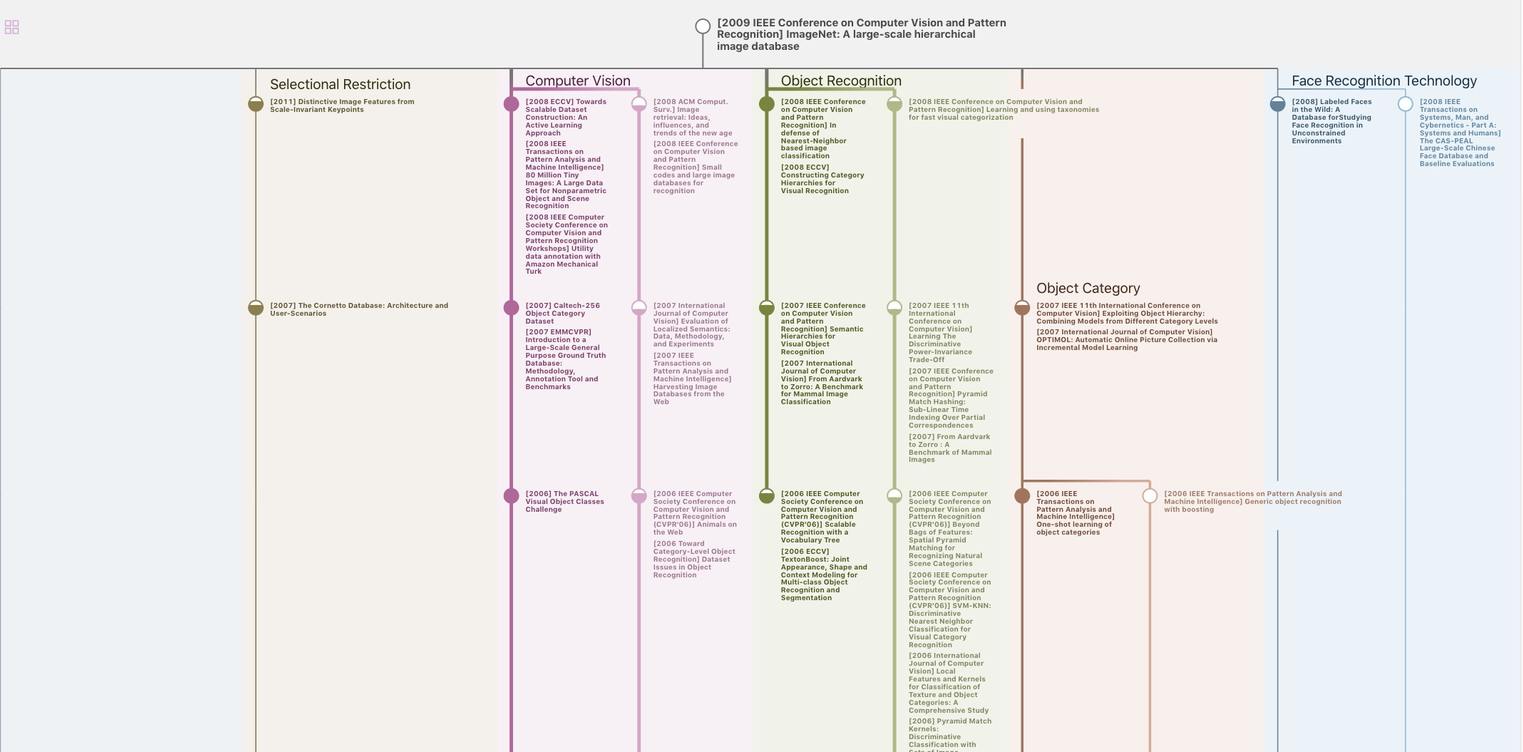
生成溯源树,研究论文发展脉络
Chat Paper
正在生成论文摘要