NeRFing it: Offline Object Segmentation Through Implicit Modeling.
ICRA(2023)
摘要
Most recently proposed methods for robotic perception are based on deep learning, which require very large datasets to perform well. The accuracy of a learned model is mainly dependent on the data distribution it was trained on. Thus for deploying such models, it is crucial to use training data belonging to the robot's environment. However, collecting and labeling data is a significant bottleneck, necessitating efficient data collection and labeling pipelines. This paper presents a method to compute high-quality object segmentation maps for RGB-D video sequences using minimal human labeling effort. We leverage the density learned by a Neural Radiance Field (NeRF) to infer the geometry of the scene, which we use to compute dense segmentation maps using a single 3D bounding box provided by a user. We study the accuracy of the computed segmentation maps and present a way to generate additional synthetic training examples observing the scene from novel viewpoints using the learned radiance fields. Our results show that our method is able to compute accurate segmentation maps, outperforming baseline and state-of-the-art methods. We also show that using the synthetic training examples improves performance on a downstream object detection task.
更多查看译文
关键词
accurate segmentation maps,additional synthetic training examples,computed segmentation maps,data distribution,deep learning,dense segmentation maps,downstream object detection task,efficient data collection,high-quality object segmentation maps,implicit modeling,labeling pipelines,learned radiance fields,minimal human labeling effort,NeRF,Neural Radiance Field,offline object segmentation,per-ception,RGB-D video sequences,robot,significant bottleneck,single 3D bounding box,training data
AI 理解论文
溯源树
样例
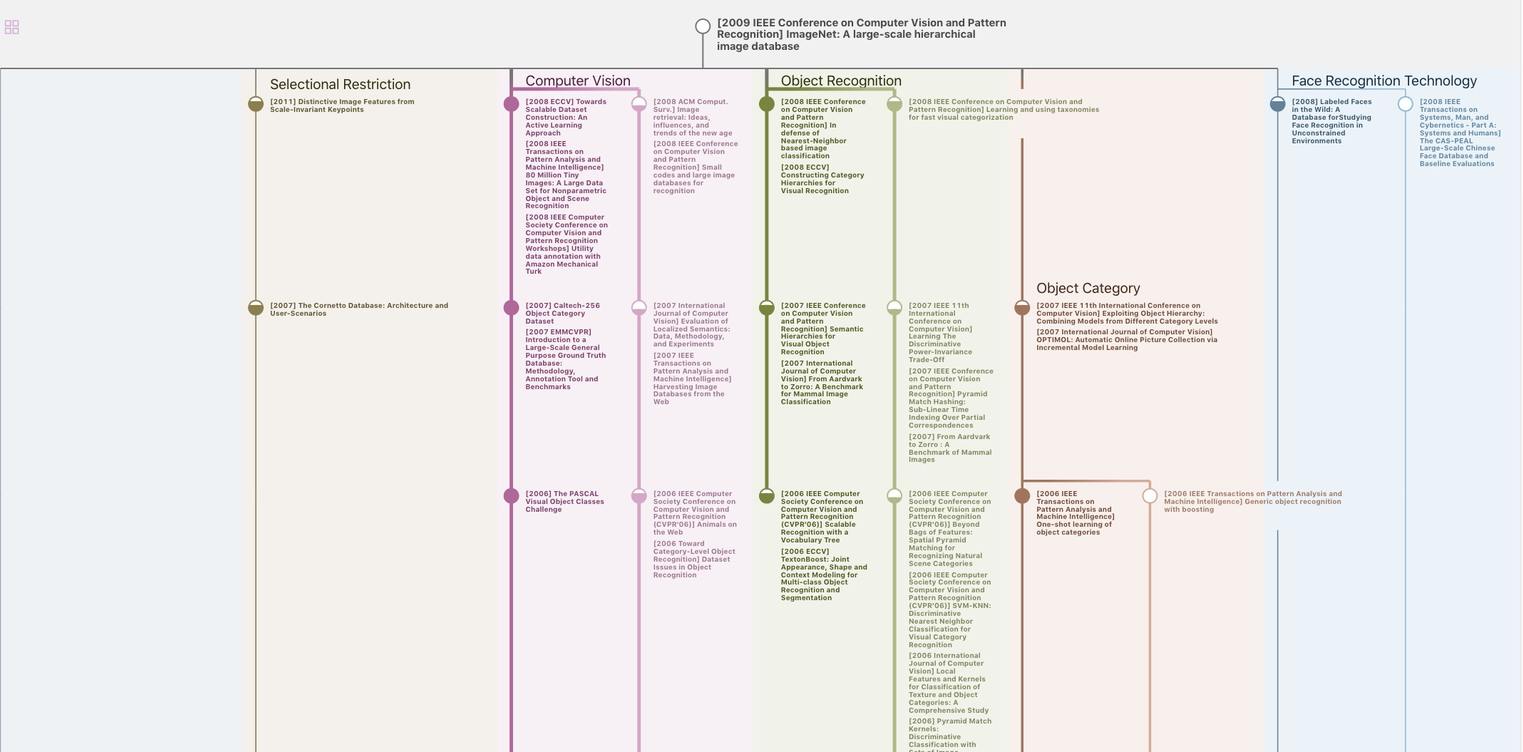
生成溯源树,研究论文发展脉络
Chat Paper
正在生成论文摘要