Memory-based Exploration-value Evaluation Model for Visual Navigation
2023 IEEE INTERNATIONAL CONFERENCE ON ROBOTICS AND AUTOMATION, ICRA(2023)
摘要
We propose a hierarchical visual navigation solution, called Memory-based Exploration-value Evaluation Model (MEEM), to improve the agent's navigation performance. MEEM employs a hierarchical policy to tackle the challenge of sparse rewards, holds an episodic memory to store the historical information of the agent, and applies an Exploration-value Evaluation Model to calculate an exploration-value for action planning at each location in the observable area. We experimentally verify MEEM by navigation performance comparison on two datasets including the grid-map dataset and the 3D scenes Gibson dataset, where our approach achieves state-of-the-art performance on both. Specifically, the overall success rate of MEEM is 95% on the grid-map dataset while the best competitor reaches 68% only. As for the Gibson dataset, the success rate of ours and the best competitor SemExp are 69.8% and 54.4%, respectively. Ablation analysis on the tile-map dataset indicates that all three components of MEEM have positive effects.
更多查看译文
AI 理解论文
溯源树
样例
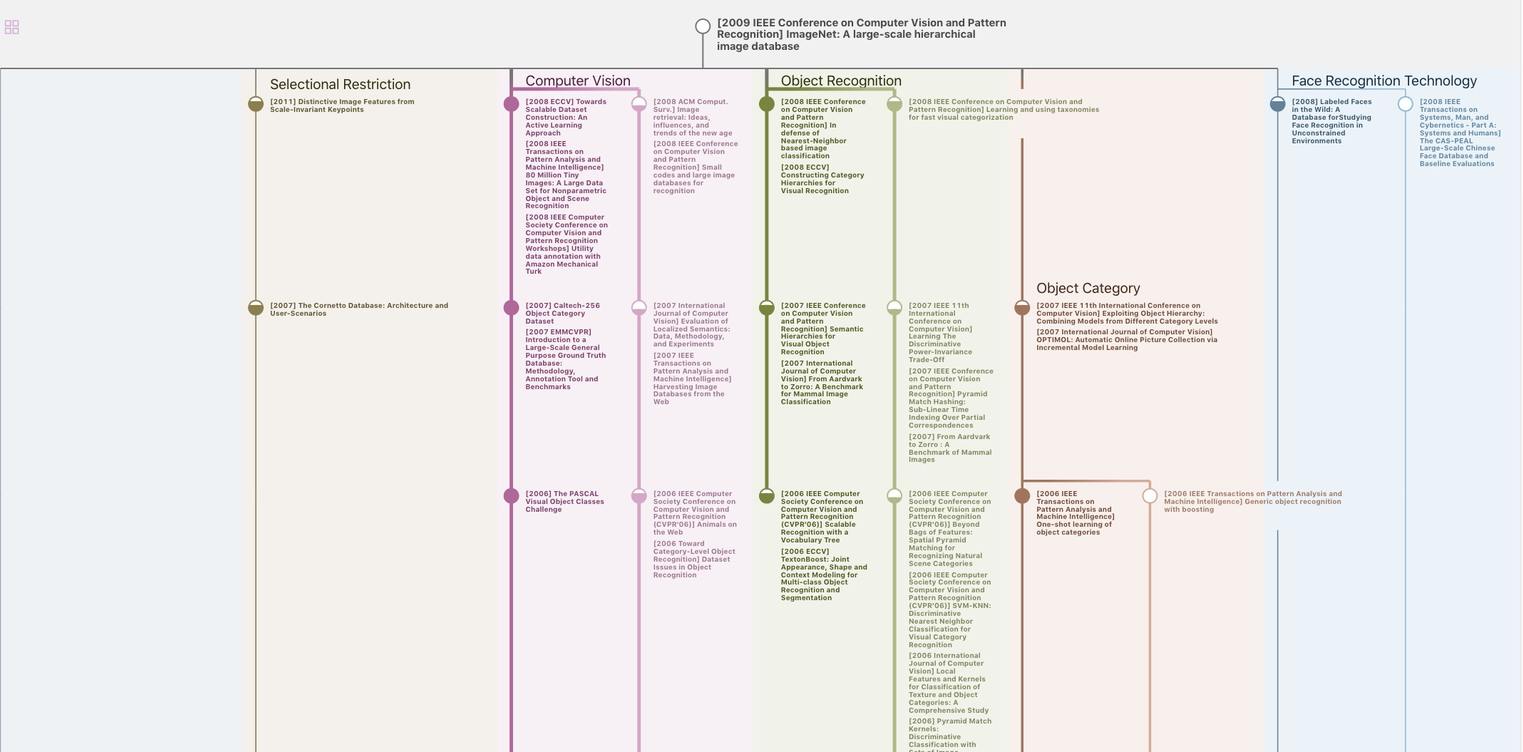
生成溯源树,研究论文发展脉络
Chat Paper
正在生成论文摘要