GraspAda: Deep Grasp Adaptation through Domain Transfer.
ICRA(2023)
摘要
Learning-based methods for robotic grasping have been shown to yield high performance. However, they rely on expensive-to-acquire and well-labeled datasets. In addition, how to generalize the learned grasping ability across different scenarios is still unsolved. In this paper, we present a novel grasp adaptation strategy to transfer the learned grasping ability to new domains based on visual data using a new grasp feature representation. We present a conditional generative model for visual data transformation. By leveraging the deep feature representational capacity from the well-trained grasp synthesis model, our approach utilizes feature-level contrastive representation learning and adopts adversarial learning on output space. This way we bridge the domain gap between the new domain and the training domain while keeping consistency during the adaptation process. Based on transformed input grasp data via the generator, our trained model can generalize to new domains without any fine-tuning. The proposed method is evaluated on benchmark datasets and based on real robot experiments. The results show that our approach leads to high performance in new scenarios.
更多查看译文
关键词
adaptation process,approach utilizes feature-level contrastive representation learning,conditional generative model,deep feature representational capacity,deep grasp adaptation,domain gap,domain transfer,grasp adaptation strategy,grasp feature representation,learned grasping ability,robotic grasping,trained model,training domain,visual data transformation
AI 理解论文
溯源树
样例
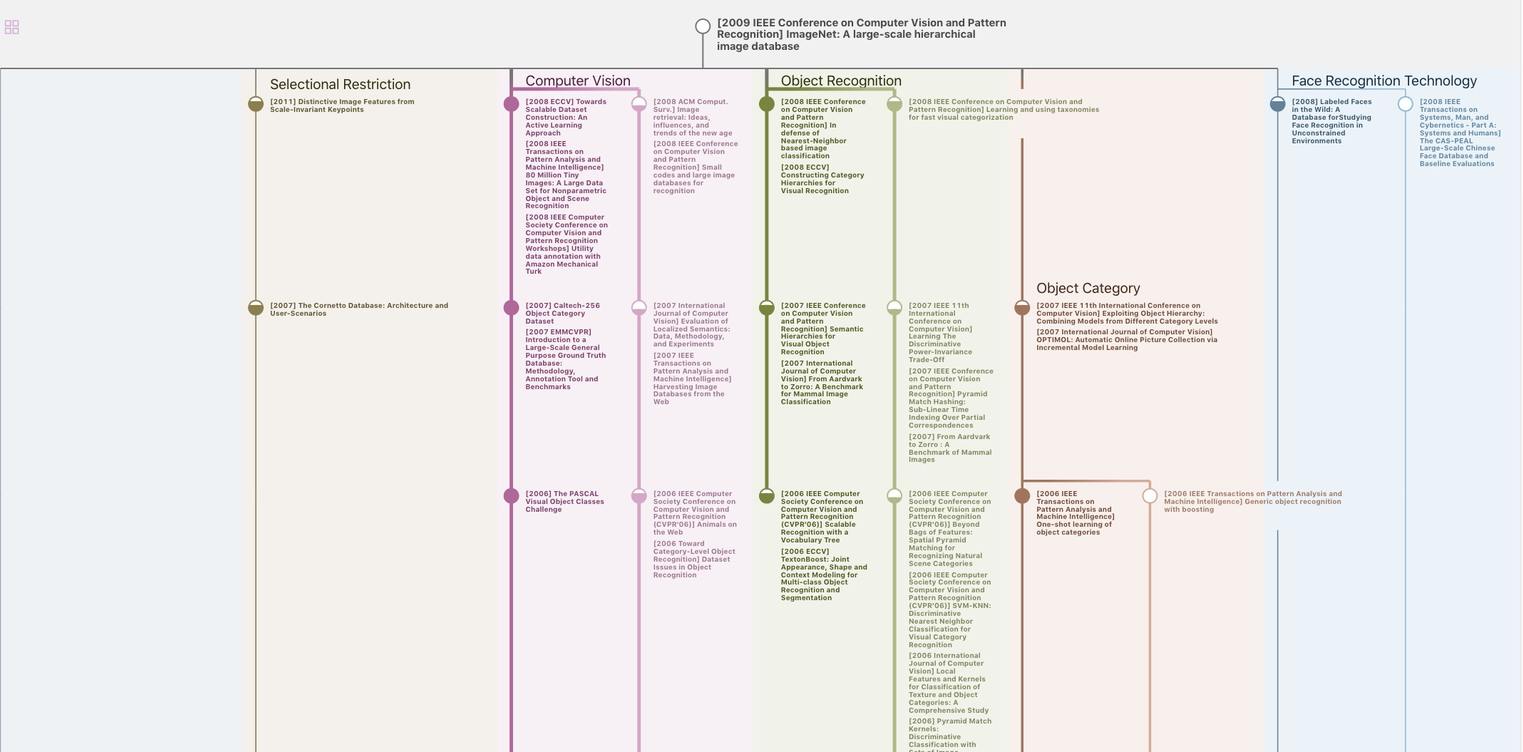
生成溯源树,研究论文发展脉络
Chat Paper
正在生成论文摘要