A Continuous Off-Policy Reinforcement Learning Scheme for Optimal Motion Planning in Simply-Connected Workspaces
ICRA(2023)
摘要
In this work, an Integral Reinforcement Learning (RL) framework is employed to provide provably safe, convergent and almost globally optimal policies in a novel Off-Policy Iterative method for simply-connected workspaces. This restriction stems from the impossibility of strictly global navigation in multiply connected manifolds, and is necessary for formulating continuous solutions. The current method generalizes and improves upon previous results, where parametrized controllers hindered the method in scope and results. Through enhancing the traditional reactive paradigm with RL, the proposed scheme is demonstrated to outperform both previous reactive methods as well as an RRT* method in path length, cost function values and execution times, indicating almost global optimality.
更多查看译文
关键词
current method generalizes,formulating continuous solutions,global optimality,Integral Reinforcement Learning framework,multiply connected manifolds,Off-Policy Iterative method,Off-Policy Reinforcement Learning scheme,optimal motion planning,optimal policies,previous reactive methods,RL,RRT* method,simply-connected workspaces,strictly global navigation
AI 理解论文
溯源树
样例
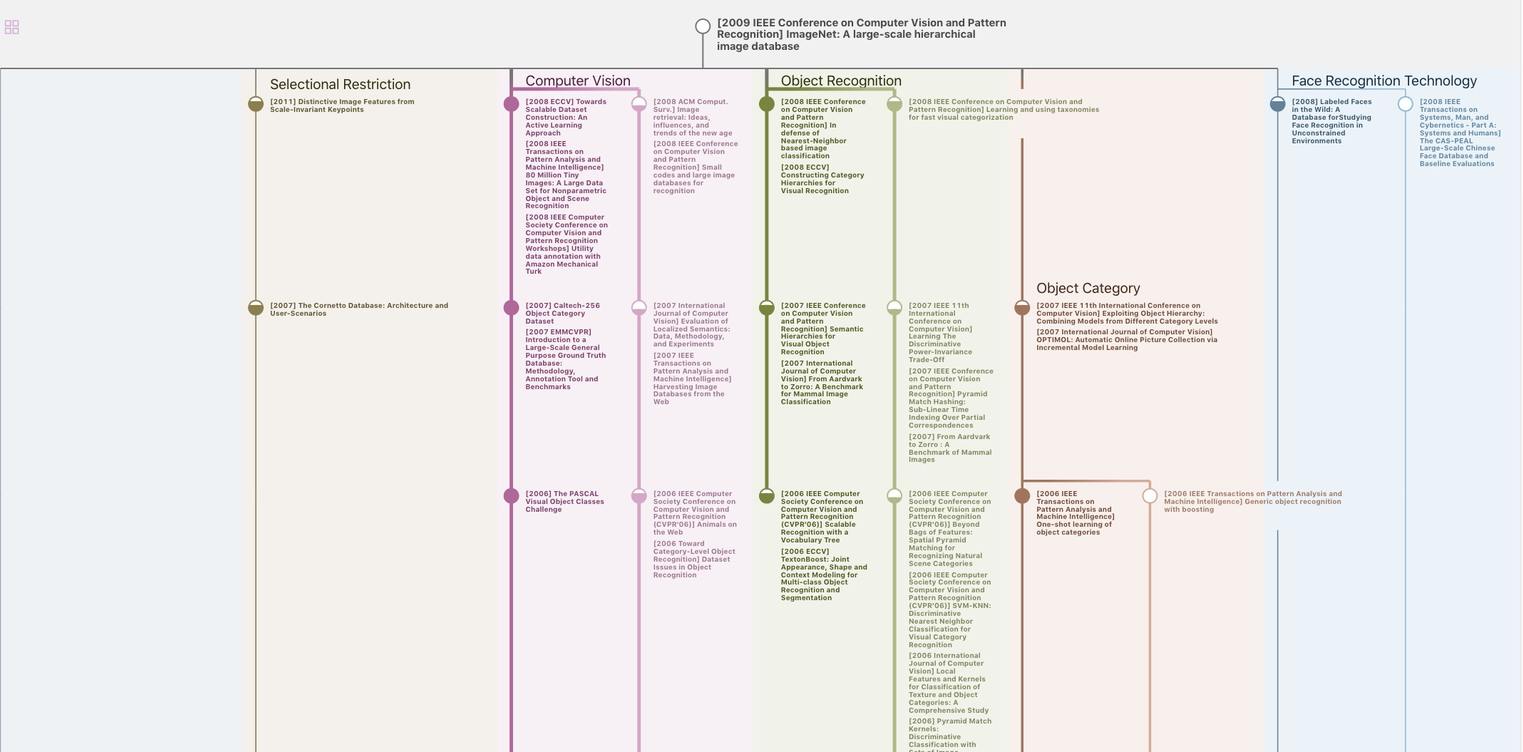
生成溯源树,研究论文发展脉络
Chat Paper
正在生成论文摘要