Test-time Domain Adaptation for Monocular Depth Estimation
2023 IEEE INTERNATIONAL CONFERENCE ON ROBOTICS AND AUTOMATION, ICRA(2023)
摘要
Test-time domain adaptation, i.e. adapting source-pretrained models to the test data on-the-fly in a source-free, unsupervised manner, is a highly practical yet very challenging task. Due to the domain gap between source and target data, inference quality on the target domain can drop drastically especially in terms of absolute scale of depth. In addition, unsupervised adaptation can degrade the model performance due to inaccurate pseudo labels. Furthermore, the model can suffer from catastrophic forgetting when errors are accumulated over time. We propose a test-time domain adaptation framework for monocular depth estimation which achieves both stability and adaptation performance by benefiting from both self-training of the supervised branch and pseudo labels from self-supervised branch, and is able to tackle the above problems: our scale alignment scheme aligns the input features between source and target data, correcting the absolute scale inference on the target domain; with pseudo label consistency check, we select confident pixels thus improve pseudo label quality; regularisation and self-training schemes are applied to help avoid catastrophic forgetting. Without requirement of further supervisions on the target domain, our method adapts the source-trained models to the test data with significant improvements over the direct inference results, providing scale-aware depth map outputs that outperform the state-of-the-arts. Code is available at https://github.com/Malefikus/ada-depth.
更多查看译文
AI 理解论文
溯源树
样例
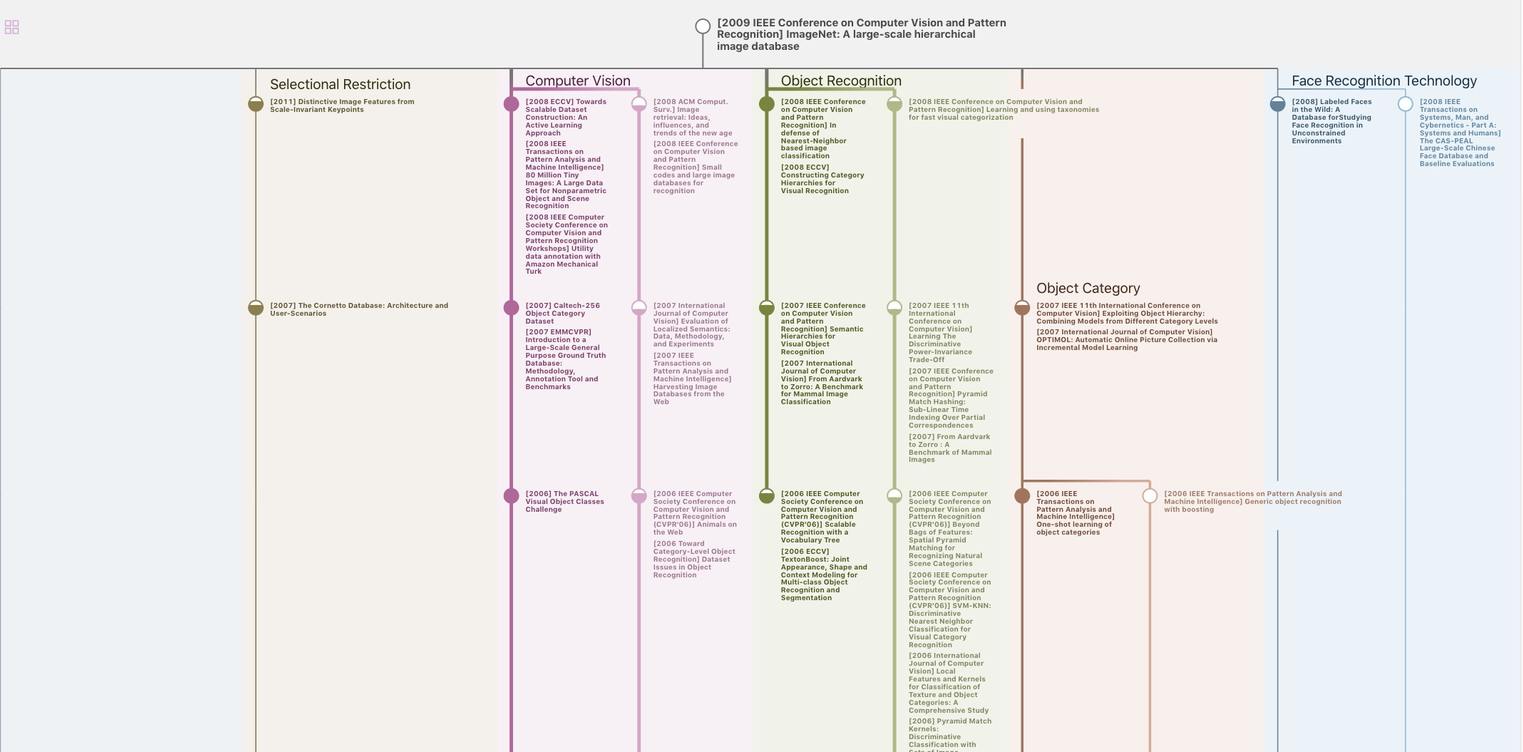
生成溯源树,研究论文发展脉络
Chat Paper
正在生成论文摘要