Real-Time Generative Grasping with Spatio-temporal Sparse Convolution.
ICRA(2023)
摘要
Robots performing mobile manipulation in unstructured environments must identify grasp affordances quickly and with robustness to perception noise. Yet in domains such as underwater manipulation, where perception noise is severe, computation is constrained, and the environment is dynamic, existing techniques fail. They are too computationally demanding, or too sensitive to noise to allow for closed loop grasping or dynamic replanning, or do not consider 6-DOF grasps. We present a novel grasp synthesis network, TSGrasp, that uses spatio-temporal sparse convolution to process a streaming point cloud in real time. The network generates 6-DOF grasps at greater speed and with less memory than Contact GraspNet, a state-of-the-art algorithm based on Point-Net++. By considering information from multiple successive frames of depth video, TSGrasp boosts robustness to noise or temporary self-occlusion and allows more grasps to be rapidly identified. Our grasp synthesis system was successfully demonstrated in an underwater environment with a Blueprint Labs Bravo robotic arm.
更多查看译文
关键词
6-DOF grasps,Blueprint Labs Bravo robotic arm,closed loop grasping,Contact GraspNet,depth video,dynamic replanning,grasp affordances,grasp synthesis system,mobile manipulation,perception noise,Point-Net++,real time generative grasping,spatio-temporal sparse convolution,streaming point cloud,temporary self-occlusion,TSGrasp,underwater environment,underwater manipulation,unstructured environments
AI 理解论文
溯源树
样例
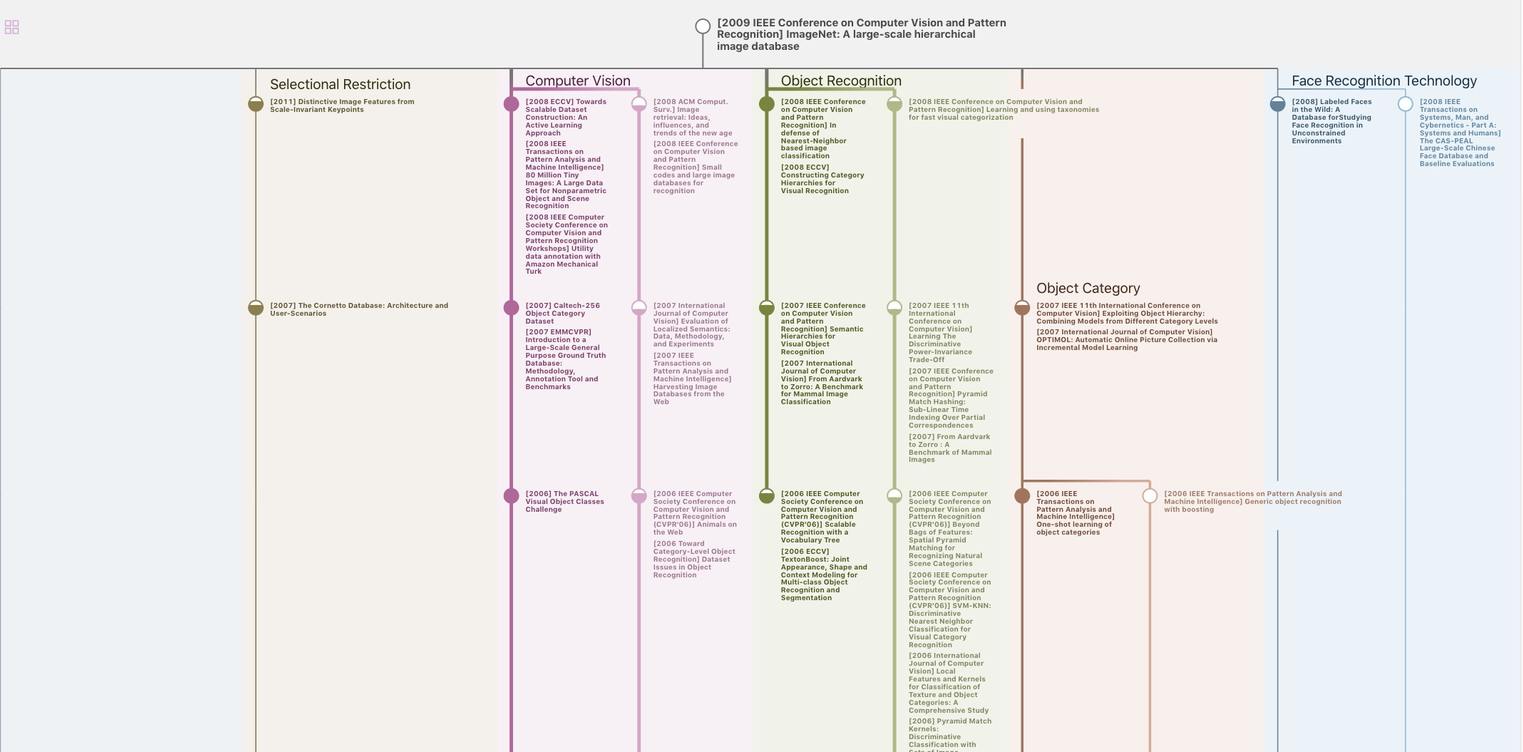
生成溯源树,研究论文发展脉络
Chat Paper
正在生成论文摘要