Asynchronous State Estimation of Simultaneous Ego-motion Estimation and Multiple Object Tracking for LiDAR-Inertial Odometry
ICRA(2023)
摘要
We propose LiDAR-Inertial Odometry via Simultaneous EGo-motion estimation and Multiple Object Tracking (LIO-SEGMOT), an optimization-based odometry approach targeted for dynamic environments. LIO-SEGMOT is formulated as a state estimation approach with asynchronous state update of the odometry and the object tracking. That is, LIO-SEGMOT can provide continuous object tracking results while preserving the keyframe selection mechanism in the odometry system. Meanwhile, a hierarchical criterion is designed to properly couple odometry and object tracking, preventing system instability due to poor detections. We compare LIO-SEGMOT against the baseline model LIO-SAM, a state-of-the-art LIO approach, under dynamic environments of the KITTI raw dataset and the self-collected Hsinchu dataset. The former experiment shows that LIO-SEGMOT obtains an average improvement 1.61% and 5.41% of odometry accuracy in terms of absolute translational and rotational trajectory errors. The latter experiment also indicates that LIO-SEGMOT obtains an average improvement 6.97% and 4.21% of odometry accuracy.
更多查看译文
关键词
Autonomous driving,SLAM,odometry,multiple object tracking
AI 理解论文
溯源树
样例
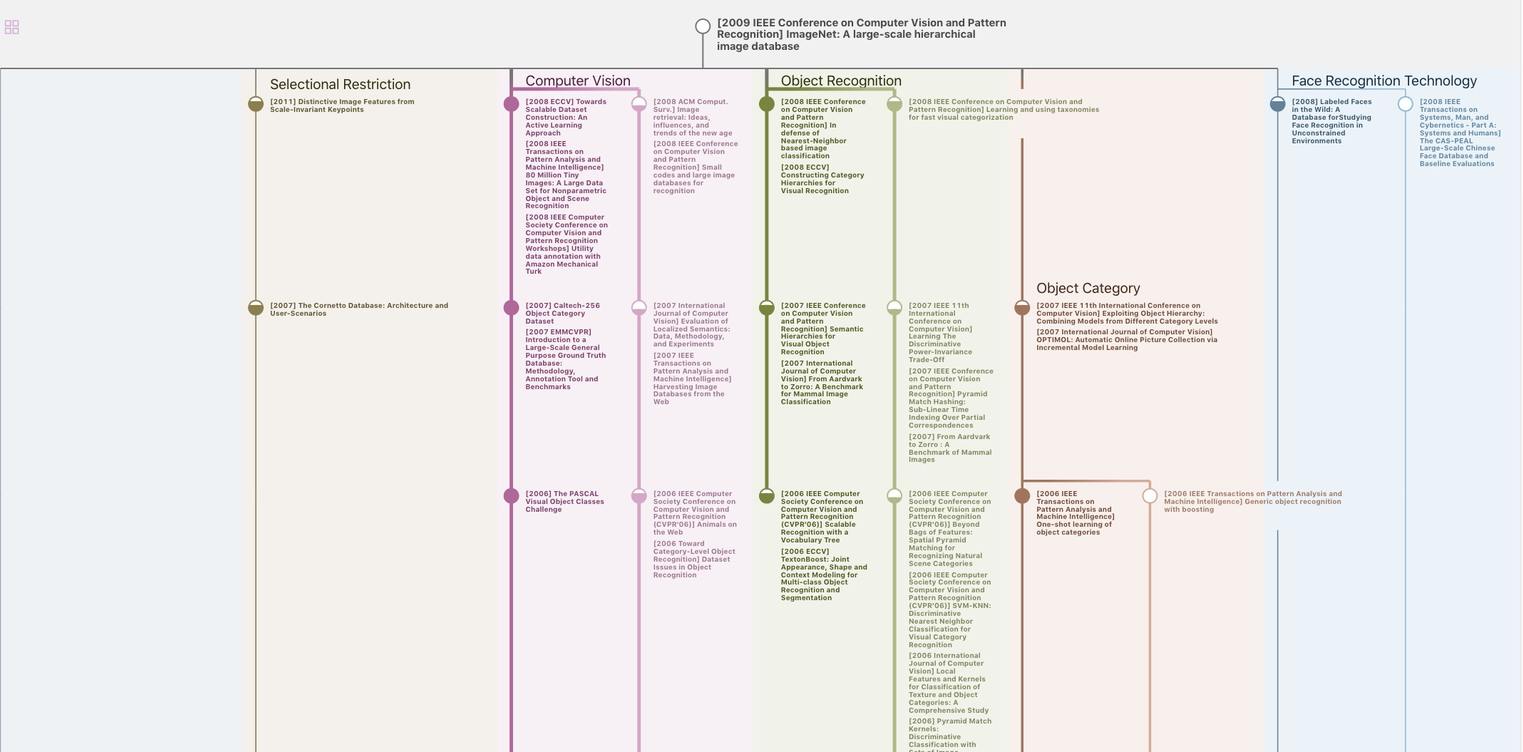
生成溯源树,研究论文发展脉络
Chat Paper
正在生成论文摘要