Learning Food Picking without Food: Fracture Anticipation by Breaking Reusable Fragile Objects
2023 IEEE INTERNATIONAL CONFERENCE ON ROBOTICS AND AUTOMATION, ICRA(2023)
摘要
Food picking is trivial for humans but not for robots, as foods are fragile. Presetting foods' physical properties does not help robots much due to the objects' inter- and intra-category diversity. A recent study proved that learning-based fracture anticipation with tactile sensors could overcome this problem; however, the method trains the model for each food to deal with intra-category differences, and tuning robots for each food leads to an undesirable amount of food consumption. This study proposes a novel framework for learning food-picking tasks without consuming foods. The key idea is to leverage the object-breaking experiences of several reusable fragile objects instead of consuming real foods while making the picking ability object-invariant with domain generalization (DG). In real-robot experiments, we trained a model with reusable objects (toy blocks, ping-pong balls, and jellies), selected based on the three common fracture types (crack, rupture, and crush). We then tested the model with four real food objects (tofu, bananas, potato chips, and tomatoes). The results showed that the proposed combination of reusable objects' breaking experiences and DG is effective for the food-picking task.
更多查看译文
AI 理解论文
溯源树
样例
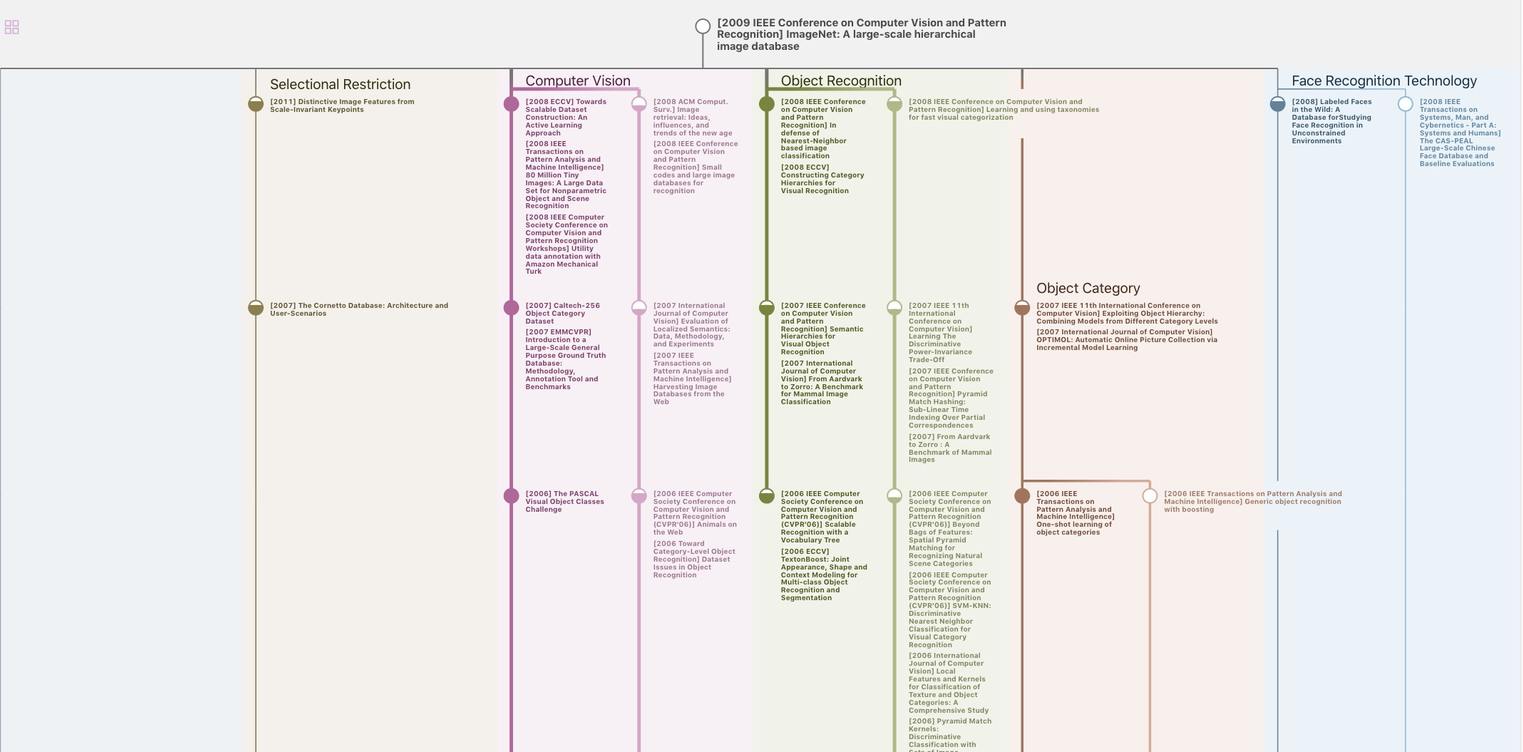
生成溯源树,研究论文发展脉络
Chat Paper
正在生成论文摘要