On Improving Boundary Quality of Instance Segmentation in Cluttered and Chaotic Scenarios.
ICRA(2023)
摘要
Instance segmentation is a long-standing task for supporting robotic bin picking. However, objects of diverse classes can be closely packed with occlusions in cluttered and chaotic scenes, hence, even recent methods could have difficulty in locating clear and precise boundaries to distinguish nearby objects. In this work, we aim to improve the boundary quality of the instance masks for robust and precise instance segmentation in these challenging scenarios. Technical-wise, we first formulate an IoU-based Boundary-aware Mask head (IBM head) for predicting the instance-level mask, boundary, and their corresponding IoU scores. With this core module, we then follow the coarse-to-fine strategy and design our pipeline with two stages: an IoUNet to learn localization-based objectness cue and a hierarchical mask refiner to produce sharper and cleaner boundaries. We deploy the IBM head throughout the framework. Extensive experimental results on three grasping benchmarks manifest that our method attains the best instance segmentation performance, compared with the state-of-the-art approaches. Practically, we conduct real-world picking tests to show that with the objectness and boundary IoU scores as guidance, we are able to filter invalid (occluded) instances and select high-fidelity (exposed) instances for grasping.
更多查看译文
关键词
chaotic scenarios,cluttered scenarios,coarse-to-fine strategy,diverse classes,IBM,IoU-based boundary-aware mask head,localization-based objectness,precise instance segmentation,robotic bin picking,robust instance segmentation
AI 理解论文
溯源树
样例
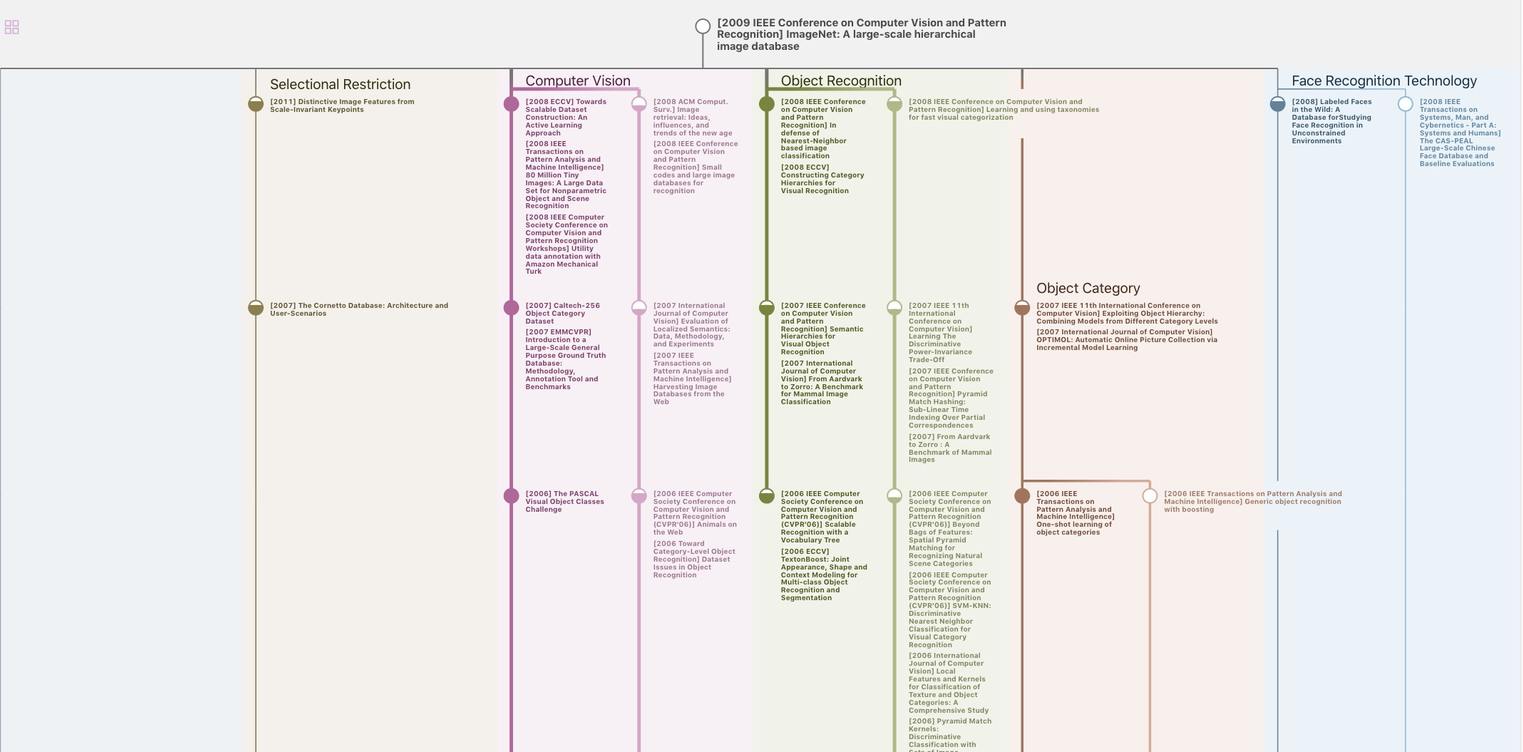
生成溯源树,研究论文发展脉络
Chat Paper
正在生成论文摘要