Global and Reactive Motion Generation with Geometric Fabric Command Sequences
2023 IEEE INTERNATIONAL CONFERENCE ON ROBOTICS AND AUTOMATION, ICRA(2023)
摘要
Motion generation seeks to produce safe and feasible robot motion from start to goal. Various tools at different levels of granularity have been developed. On one extreme, sampling-based motion planners focus on completeness - a solution, if it exists, would eventually be found. However, produced paths are often of low quality, and contain superfluous motion. On the other, reactive methods optimise the immediate cost to obtain the next controls, producing smooth and legible motion that can quickly adapt to perturbations, uncertainties, and changes in the environment. However, reactive methods are highly local, and often produce motion that become trapped in non-convex regions of the environment. This paper contributes, Geometric Fabric Command Sequences, a method that lies in the middle ground. It can produce globally optimal motion that is smooth and intuitive, while being also reactive. We model motion via a reactive Geometric Fabric policy that ingests a sequence of attractor states, or commands, and then apply global optimisation over the space of commands. We postulate that solutions for different problems and scenes are highly transferable when conditioned on environmental features. Therefore, an implicit generative model is trained on solutions from optimisation and environment features in a self-supervised manner. That is, faced with multiple motion generation problems, the learning and optimisation are contained within the same loop: the optimisation generates labels for learning, while the learning improves the optimisation for the next problem, which in turn provides higher quality labels. We empirically validate our method in both simulation and on a real-world 6-DOF JACO arm.
更多查看译文
AI 理解论文
溯源树
样例
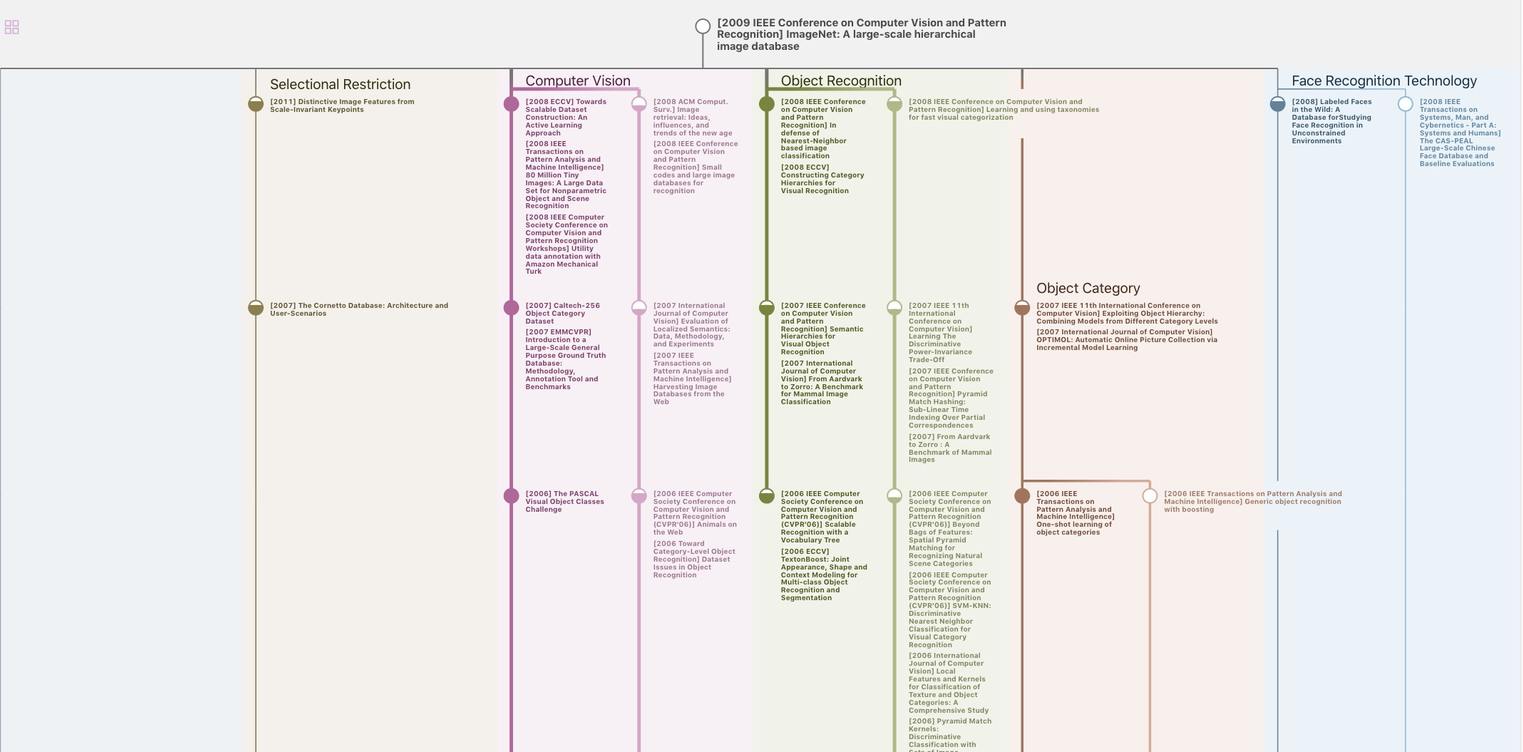
生成溯源树,研究论文发展脉络
Chat Paper
正在生成论文摘要