Collaborative Scheduling with Adaptation to Failure for Heterogeneous Robot Teams
2023 IEEE INTERNATIONAL CONFERENCE ON ROBOTICS AND AUTOMATION, ICRA(2023)
摘要
Collaborative scheduling is an essential ability for a team of heterogeneous robots to collaboratively complete complex tasks, e.g., in a multi-robot assembly application. To enable collaborative scheduling, two key problems should be addressed, including allocating tasks to heterogeneous robots and adapting to robot failures in order to guarantee the completion of all tasks. In this paper, we introduce a novel approach that integrates deep bipartite graph matching and imitation learning for heterogeneous robots to complete complex tasks as a team. Specifically, we use a graph attention network to represent attributes and relationships of the tasks. Then, we formulate collaborative scheduling with failure adaptation as a new deep learning-based bipartite graph matching problem, which learns a policy by imitation to determine task scheduling based on the reward of potential task schedules. During normal execution, our approach generates robot-task pairs as potential allocations. When a robot fails, our approach identifies not only individual robots but also subteams to replace the failed robot. We conduct extensive experiments to evaluate our approach in the scenarios of collaborative scheduling with robot failures. Experimental results show that our approach achieves promising, generalizable and scalable results on collaborative scheduling with robot failure adaptation.
更多查看译文
AI 理解论文
溯源树
样例
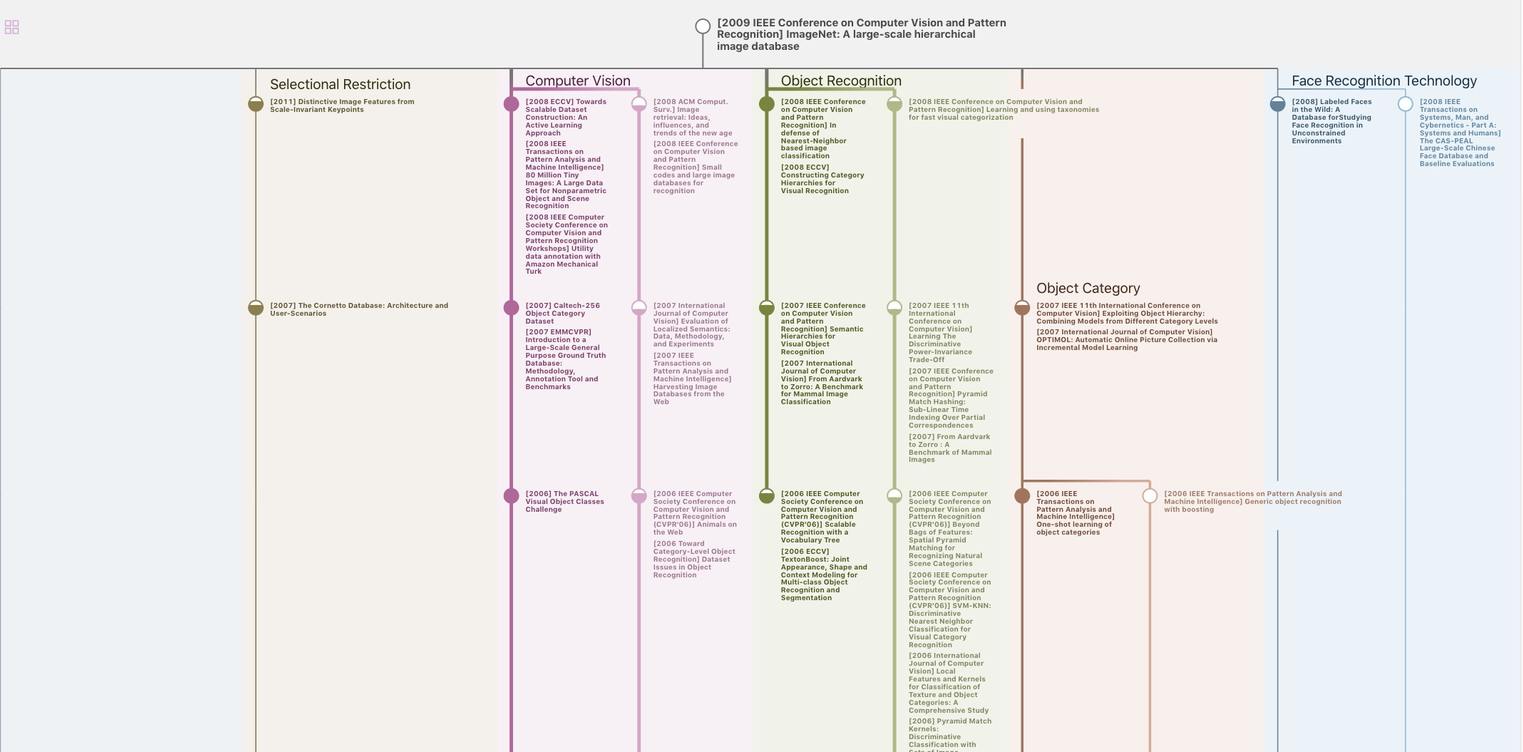
生成溯源树,研究论文发展脉络
Chat Paper
正在生成论文摘要