SNARGs for Monotone Policy Batch NP.
CRYPTO (2)(2023)
摘要
We construct a succinct non-interactive argument ( SNARG ) for the class of monotone policy batch NP languages, under the Learning with Errors ( LWE ) assumption. This class is a subclass of NP that is associated with a monotone function f : { 0 , 1 } k → { 0 , 1 } and an NP language L , and contains instances ( x 1 , … , x k ) such that f ( b 1 , … , b k ) = 1 where b j = 1 if and only if x j ∈ L . Our SNARG s are arguments of knowledge in the non-adaptive setting, and satisfy a new notion of somewhere extractability against adaptive adversaries. This is the first SNARG under standard hardness assumptions for a sub-class of NP that is not known to have a (computational) non-signaling PCP with parameters compatible with the standard framework for constructing SNARG s dating back to [Kalai-Raz-Rothblum, STOC ’13]. Indeed, our approach necessarily departs from this framework. Our construction combines existing quasi-arguments for NP (based on batch arguments for NP ) with a new type of cryptographic encoding of the instance and a new analysis going from local to global soundness. The main novel ingredient used in our encoding is a predicate-extractable hash ( PEHash ) family, which is a primitive that generalizes the notion of a somewhere extractable hash. Whereas a somewhere extractable hash allows to extract a single input coordinate, our PEHash extracts a global property of the input. We view this primitive to be of independent interest, and believe that it will find other applications.
更多查看译文
关键词
batch,snargs,policy
AI 理解论文
溯源树
样例
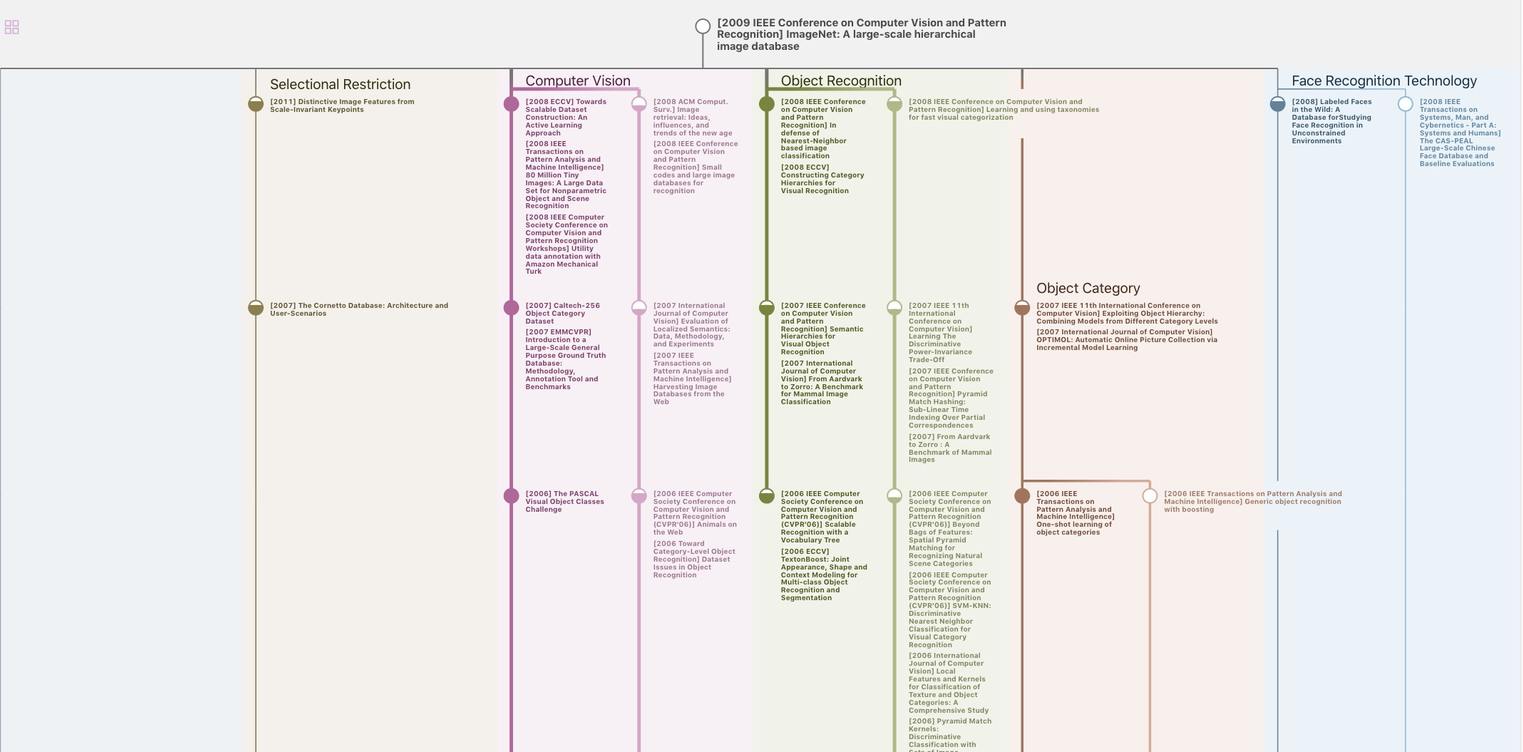
生成溯源树,研究论文发展脉络
Chat Paper
正在生成论文摘要