Learning Deposition Policies for Fused Multi-Material 3D Printing.
ICRA(2023)
摘要
3D printing based on continuous deposition of materials, such as filament-based 3D printing, has seen widespread adoption thanks to its versatility in working with a wide range of materials. An important shortcoming of this type of technology is its limited multi-material capabilities. While there are simple hardware designs that enable multi-material printing in principle, the required software is heavily underdeveloped. A typical hardware design fuses together individual materials fed into a single chamber from multiple inlets before they are deposited. This design, however, introduces a time delay between the intended material mixture and its actual deposition. In this work, inspired by diverse path planning research in robotics, we show that this mechanical challenge can be addressed via improved printer control. We propose to formulate the search for optimal multi-material printing policies in a reinforcement learning setup. We put forward a simple numerical deposition model that takes into account the non-linear material mixing and delayed material deposition. To validate our system we focus on color fabrication, a problem known for its strict requirements for varying material mixtures at a high spatial frequency. We demonstrate that our learned control policy outperforms state-of-the-art hand-crafted algorithms.
更多查看译文
关键词
actual deposition,delayed material deposition,deposition policies,diverse path planning research,filament-based 3D printing,fused multimaterial 3D,important shortcoming,individual materials,intended material mixture,learned control policy,material mixtures,multimaterial capabilities,nonlinear material mixing,optimal multimaterial printing policies,reinforcement learning setup,required software,simple hardware designs,simple numerical deposition model,typical hardware design fuses,widespread adoption thanks
AI 理解论文
溯源树
样例
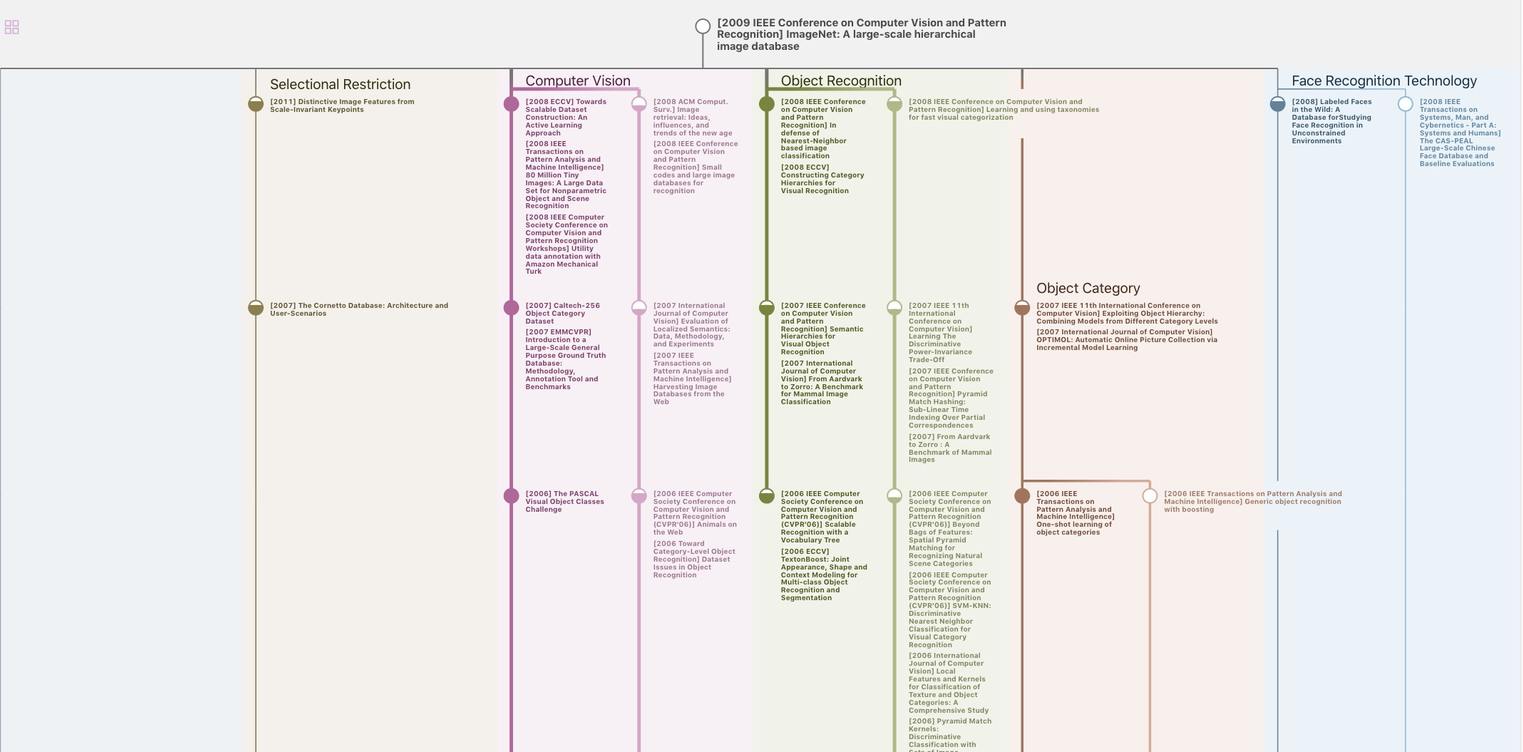
生成溯源树,研究论文发展脉络
Chat Paper
正在生成论文摘要