Improving The Local Stability of Deep Model With Margin Losses.
IJCNN(2023)
摘要
One of the serious problems encountered by deep models is their high sensitivity to small perturbations on the input samples. It is mainly manifested in two aspects, one is the problem of adversarial samples and the other is that the gradient interpretation results of similar samples are quite different, which seriously affects the reliability and dependence of the model. In this paper, we first obtain a new loss by deriving an upper bound of the expectation of the CE loss on the local Gaussian sampled data, which is called Margin Losses. Further, under the inspiration of this margin loss and in order to improve the computational efficiency, we designed another Margin Losses based on the loss gradient. The idea of these two Margin Losses is to force samples away from the decision-making boundary to ensure local stability. Empirical results on MNIST, CIFAR-10, and CIFAR-100 demonstrate that our losses can indeed improve the model's resistance to adversarial noise, and at the same time can promote the local consistency of the model gradient.
更多查看译文
关键词
adversarial samples, reliability, margin loss, stability
AI 理解论文
溯源树
样例
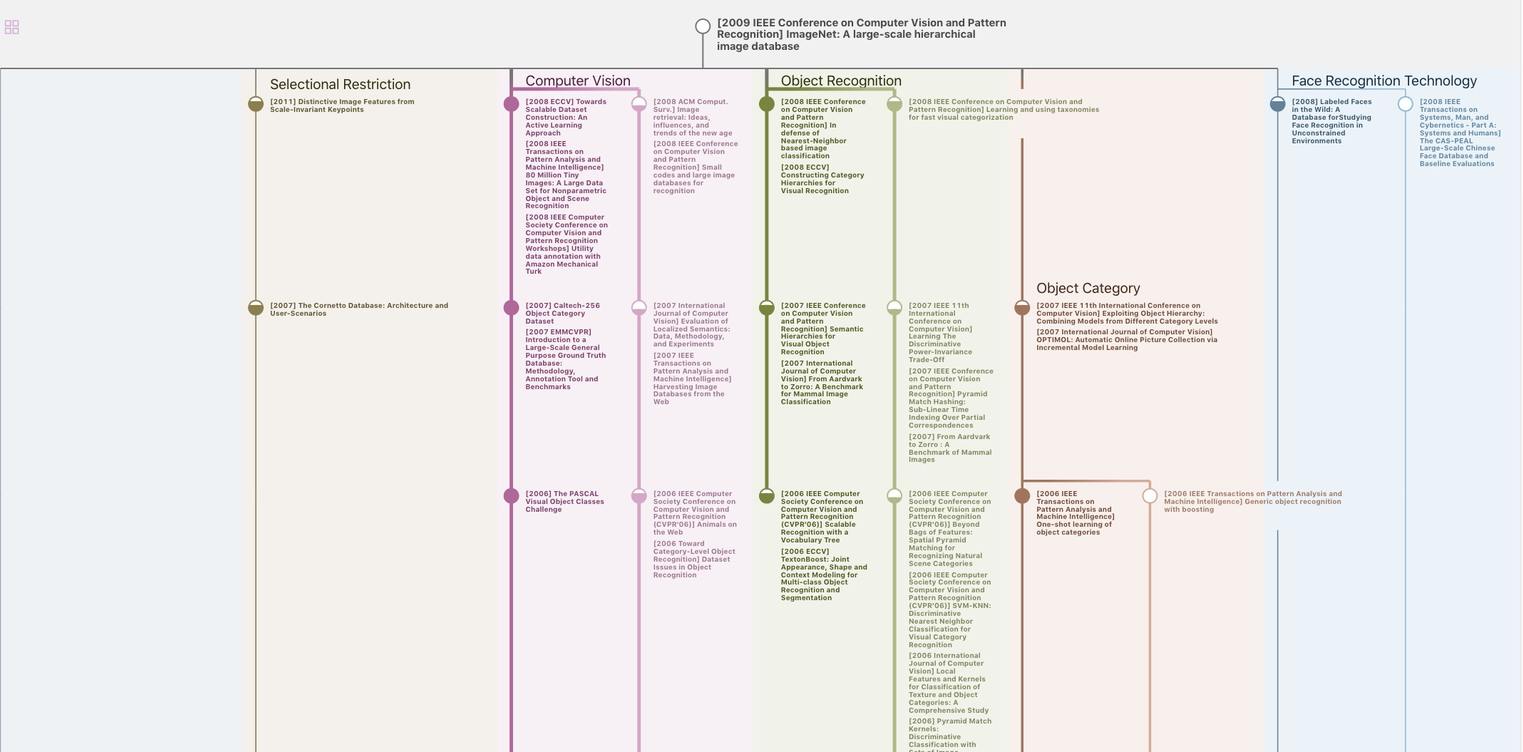
生成溯源树,研究论文发展脉络
Chat Paper
正在生成论文摘要