HSimCSE: Improving Contrastive Learning of Unsupervised Sentence Representation with Adversarial Hard Positives and Dual Hard Negatives.
IJCNN(2023)
摘要
Recently, contrastive learning (CL) has emerged as the fundamental framework for learning better sentence representations. In the unsupervised sentence representation task, due to the lack of labeled data, current CL-based approaches generally use various methods to generate or select positive and negative samples for the given sentence. Despite their success, existing CL-based unsupervised sentence representation methods underestimate hard positive samples and hard negative samples, which do not fully exploit the power of contrastive learning. In this paper, we argue that we need to focus more on hard positive and hard negative samples. To this end, we propose a novel contrastive learning model, HSimCSE, that extends SimCSE by considering both the hard positive and hard negative samples. Specifically, we first propose a novel adversarial positive sample generation module to generate an adversarial hard positive sample, then we propose a dual negative sample selection module to select hard negative samples from the in-batch samples and the entire training corpus. Finally, we propose a quadruplet loss to minimize the distance between the anchor sample and the adversarial hard positive sample and maximize the distance between the anchor sample and the two hard negative samples. Experiments conducted on seven semantic text similarity tasks demonstrate the effectiveness of our method. The source code can be found at https://github.com/xubodhu/HSimCSE.
更多查看译文
关键词
adversarial hard positive sample,adversarial positive sample generation module,anchor sample,CL-based unsupervised sentence representation methods,contrastive learning model,dual hard negatives,dual negative sample selection module,hard negative samples,HSimCSE,in-batch samples,quadruplet loss,semantic text similarity tasks
AI 理解论文
溯源树
样例
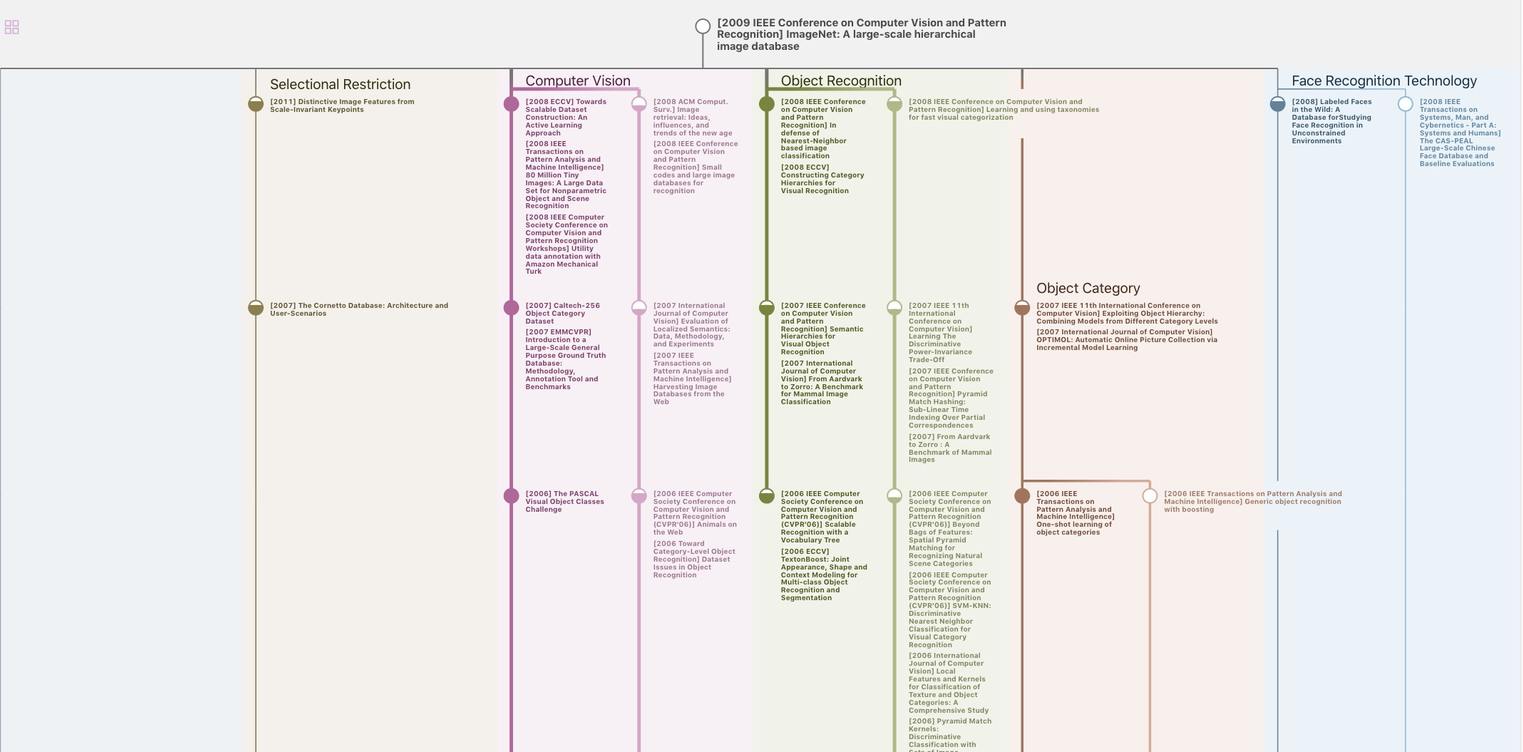
生成溯源树,研究论文发展脉络
Chat Paper
正在生成论文摘要