Boosting External-Reference Image Quality Assessment by Content-Constrain Loss and Attention-based Adaptive Feature Fusion.
IJCNN(2023)
摘要
With the development of deep learning, image quality assessment (IQA) methods have made significant progress, but full-reference (FR) methods are limited by the reference image, and the performance of no-reference (NR) methods is relatively poor. Therefore, some researchers have introduced a new scheme, external-reference (ER) IQA, which uses an arbitrary highquality image as the reference image (external reference image). The key to ER-IQA is how to extract useful reference information from external reference images and use it effectively. Since the content of the external reference image is independent of the distorted image, we think that the content information of the external reference image is harmful to the algorithm. Therefore, a content-constrain loss is designed for training the network to suppress the content information of external reference images. To utilize the external reference information more effectively, we design an attention-based adaptive feature fusion (AAFF) module. Experimental results demonstrate the effectiveness of the designed loss and feature fusion module.
更多查看译文
关键词
image quality assessment, external-reference, content-constrain loss, attention-based adaptive feature fusion
AI 理解论文
溯源树
样例
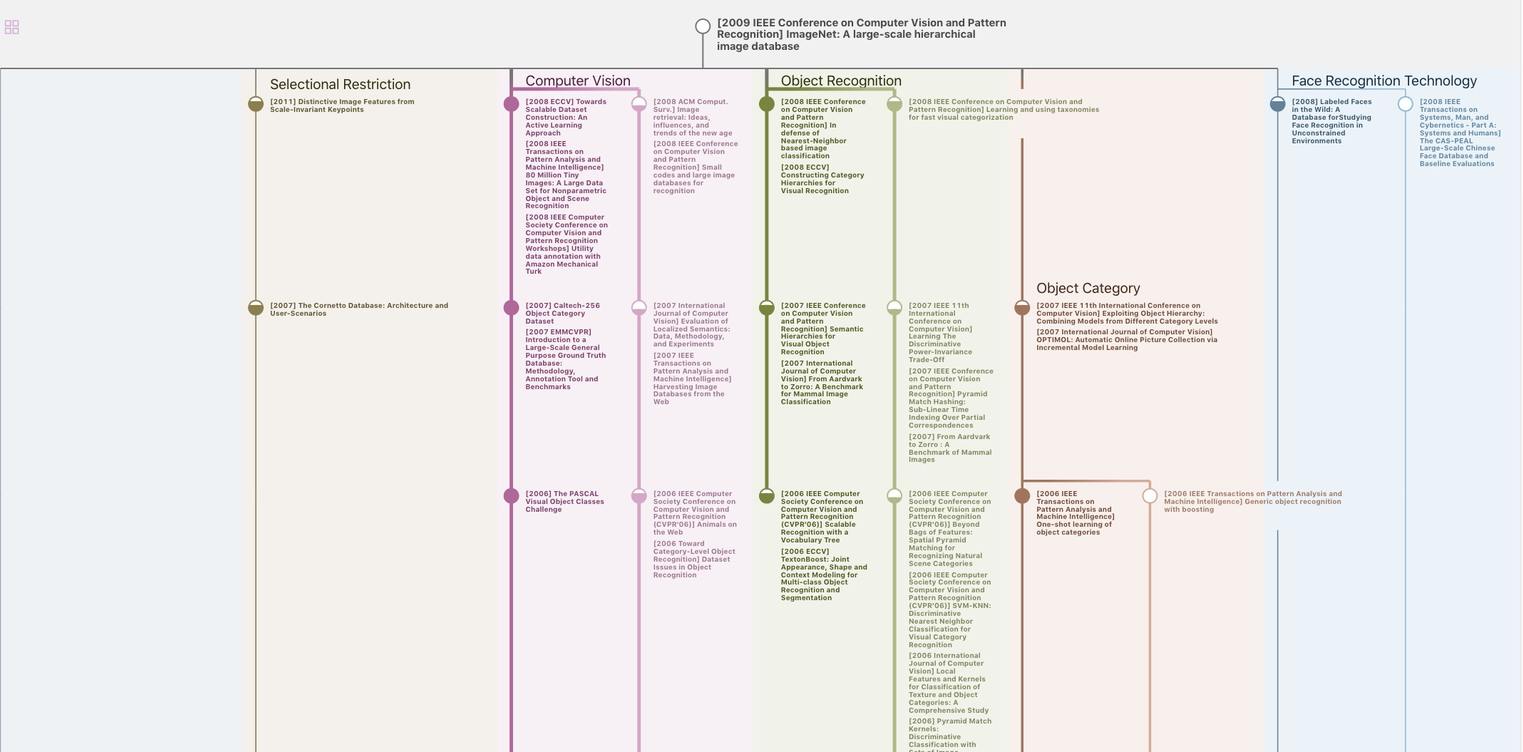
生成溯源树,研究论文发展脉络
Chat Paper
正在生成论文摘要