Pairwise and Hyper-correlations Based Spatiotemporal Neural Networks for Traffic Speed Predictions.
MDM(2023)
摘要
The problem of traffic speed predictions is still very challenging due to the complex and dynamic urban traffic conditions. Many existing works have implied the importance of integrating spatial correlations into models to explore nonlinear spatio-temporal dependencies and make traffic predictions in near future. However, some of the works only consider pairwise correlations and cannot model the hidden information among multiple nodes well, and the others only consider hyper-correlations (that can be shared by more than two nodes) and discount the role of the pairwise ones for propagating spatial dependencies. Therefore, we propose a Spatio-Temporal neural nEtwork based on both Pairwise and Hyper-correlations (STEPH) for traffic speed predictions. It is distinguished primarily by incorporating both types of spatial correlations into temporal information and designing new hybrid spatio-temporal blocks in neural networks to effectively overcome the challenge. Experiments on two real-world traffic datasets demonstrate the effectiveness of the proposed model, and show its superiority performance compared to other state-of-the-art baselines.
更多查看译文
关键词
traffic predictions,graph convolutions,hypergraph convolutions,hybrid spatio-temporal blocks
AI 理解论文
溯源树
样例
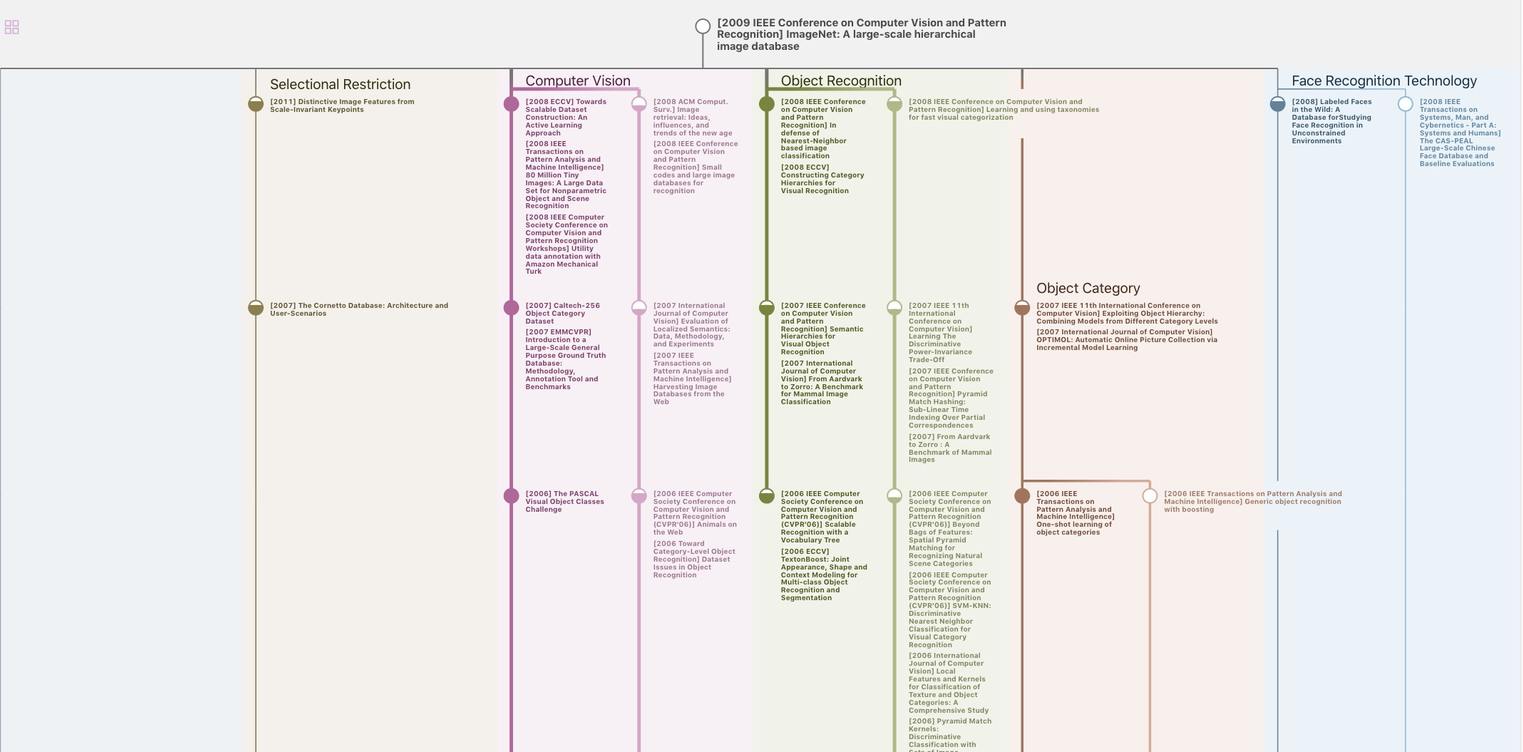
生成溯源树,研究论文发展脉络
Chat Paper
正在生成论文摘要