Dense Attention: A Densely Connected Attention Mechanism for Vision Transformer.
IJCNN(2023)
摘要
Recently, Vision Transformer has demonstrated its impressive capability in image understanding. The multi-head self-attention mechanism is fundamental to its formidable performance. However, self-attention has the drawback of high computational effort, which makes the training of the model require powerful computational resources or more time. This paper designs a novel and efficient attention mechanism Dense Attention to overcome the above problem. Dense attention aims to focus on features from multiple views through a dense connection paradigm. Benefiting from the attention of comprehensive features, dense attention can i) remarkably strengthen the image representation of the model, and ii) partially replace the multi-head self-attention mechanism to allow model slimming. To verify the effectiveness of dense attention, we implement it in the prevalent Vision Transformer models, including non-pyramid architecture DeiT and pyramid architecture Swin Transformer. The experimental results on ImageNet classification show that dense attention indeed contributes to performance improvement, +1.8/1.3% for DeiT-T/S and +0.7/+1.2% for Swin-T/S, respectively. Dense attention also demonstrates its transferability on CIFAR10 and CIFAR100 recognition benchmarks with classification accuracy of 98.9% and 89.6% respectively. Furthermore, dense attention can weaken the performance sacrifice caused by the pruning in the number of heads. Code and pre-trained models will be available(1).
更多查看译文
关键词
Dense connection,vision transformer,model slimming,image classification,pyramid
AI 理解论文
溯源树
样例
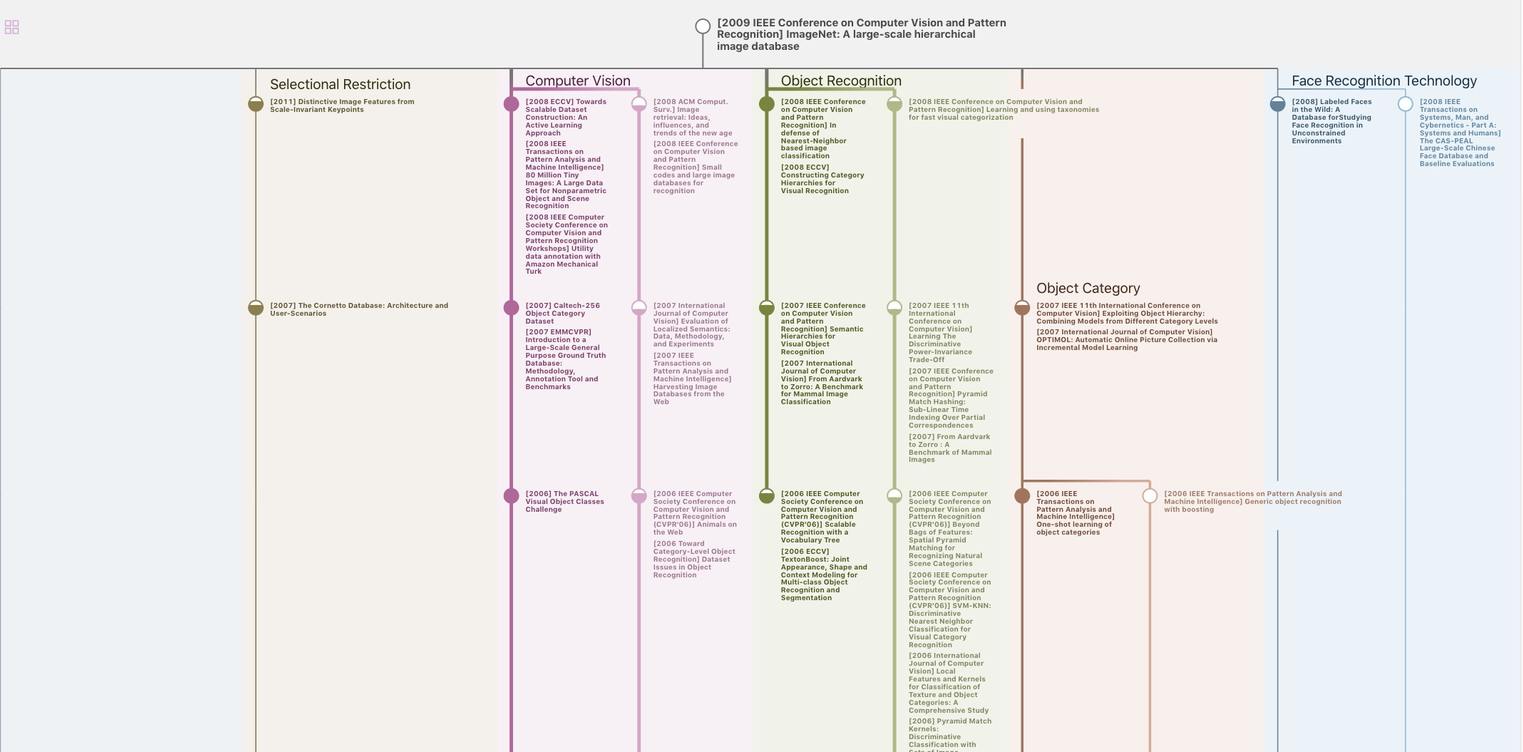
生成溯源树,研究论文发展脉络
Chat Paper
正在生成论文摘要