Combining datasets to improve model fitting.
IJCNN(2023)
摘要
For many use cases, combining information from different datasets can be of interest to improve a machine learning model's performance, especially when the number of samples from at least one of the datasets is small. An additional challenge in such cases is that the features from these datasets are not identical, even though there are some commonly shared features among the datasets. To tackle this, we propose a novel framework called Combine datasets based on Imputation (ComImp). In addition, we propose PCA-ComImp, a variant of ComImp that utilizes Principle Component Analysis (PCA), where dimension reduction is conducted before combining datasets. This is useful when the datasets have a large number of features that are not shared across them. Furthermore, our framework can also be utilized for data preprocessing by imputing missing data, i.e., filling in the missing entries while combining different datasets. To illustrate the performance and practicability of the proposed methods and their potential usages, we conduct experiments for various tasks (regression, classification) and for different data types (tabular data, time series data) when the datasets to be combined have missing data. We also investigate how the devised methods can be used with transfer learning to provide even further model training improvement. Our results indicate that can provide extra improvement when being used in combination with transfer learning.
更多查看译文
关键词
combining datasets,transfer learning,missing data,imputation
AI 理解论文
溯源树
样例
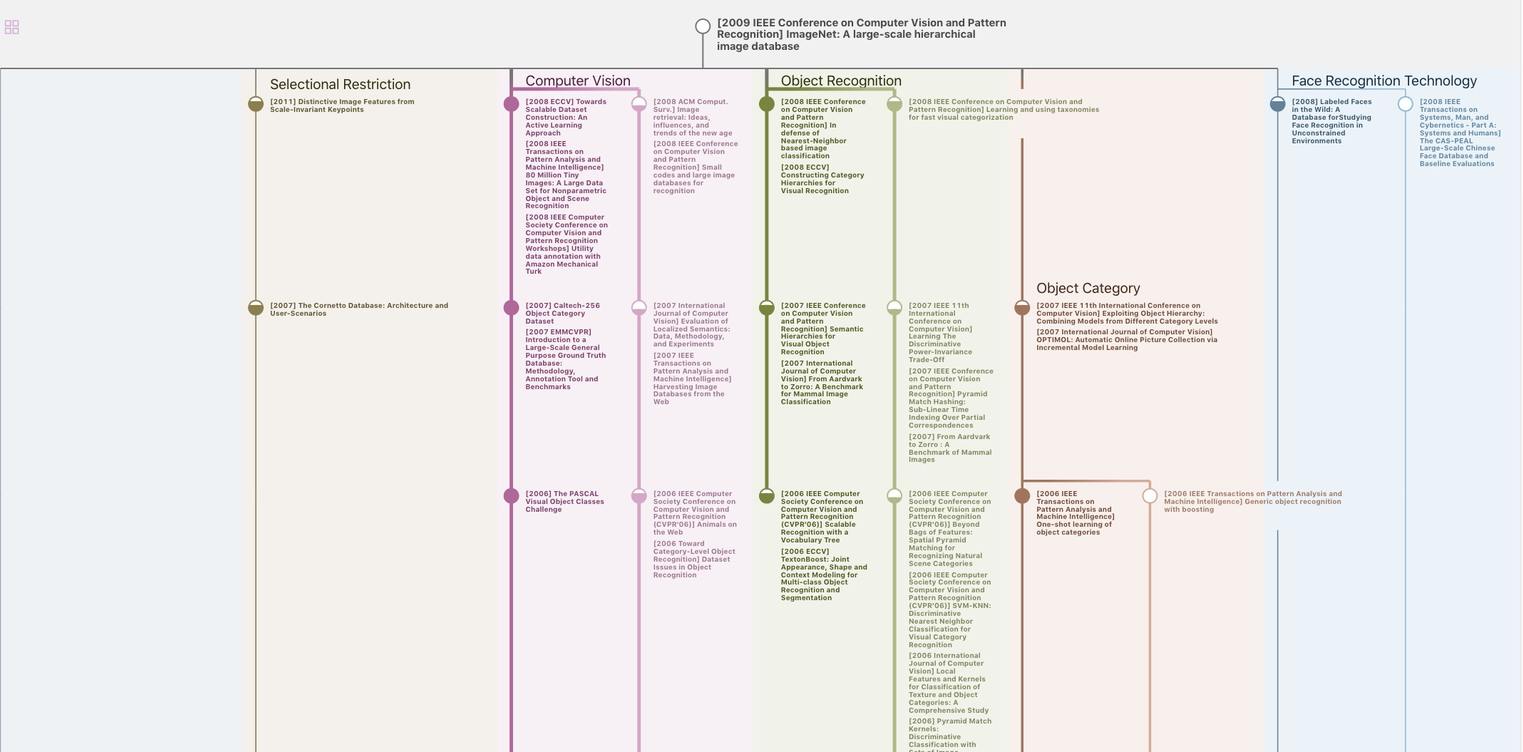
生成溯源树,研究论文发展脉络
Chat Paper
正在生成论文摘要