Dual-Path Reconstruction Guided Segmentation Network for Unsupervised Anomaly Detection and Localization.
IJCNN(2023)
摘要
Visual anomaly detection methods with localization are critically important for industrial manufacturing quality control. Because of the rarity of anomalies and the irregular variation of anomaly patterns, unsupervised methods have been widely explored. For the anomaly detection and localization tasks, the image reconstruction-based approaches have shown competitive performance. However, reconstruction results of those methods are coarse and visually blurred, which leads to a high rate of false detection and false pixel-level localization. To address those issues, we propose a framework called Dual-Path Reconstruction Guided Segmentation Network (DRGS-Net), which determines the abnormal regions by segmenting the anomaly image with its reconstructed result as the reference template. DRGS-Net mainly consists of a novel dual-path reconstruction sub-network and a specifically designed anomaly segmentation sub-network. They are jointly trained end-to-end, with the former fusing texture repair and image reconstruction information to obtain fine-grained reconstruction results and the latter learning a decision boundary between normal and anomalous regions based on reconstruction results. On the standard benchmark dataset MVTecAD and an additional dataset DAGM, DRGS-Net shows competitive performance in image-level detection and achieves outstanding improvement in pixel-level localization. Further experiments with the few samples setting demonstrate that DRGS-Net retains strong performance with only few anomaly-free training images.
更多查看译文
关键词
Unsupervised, Anomaly detection, Pixel-level localization, Dual-path reconstruction, MVTecAD
AI 理解论文
溯源树
样例
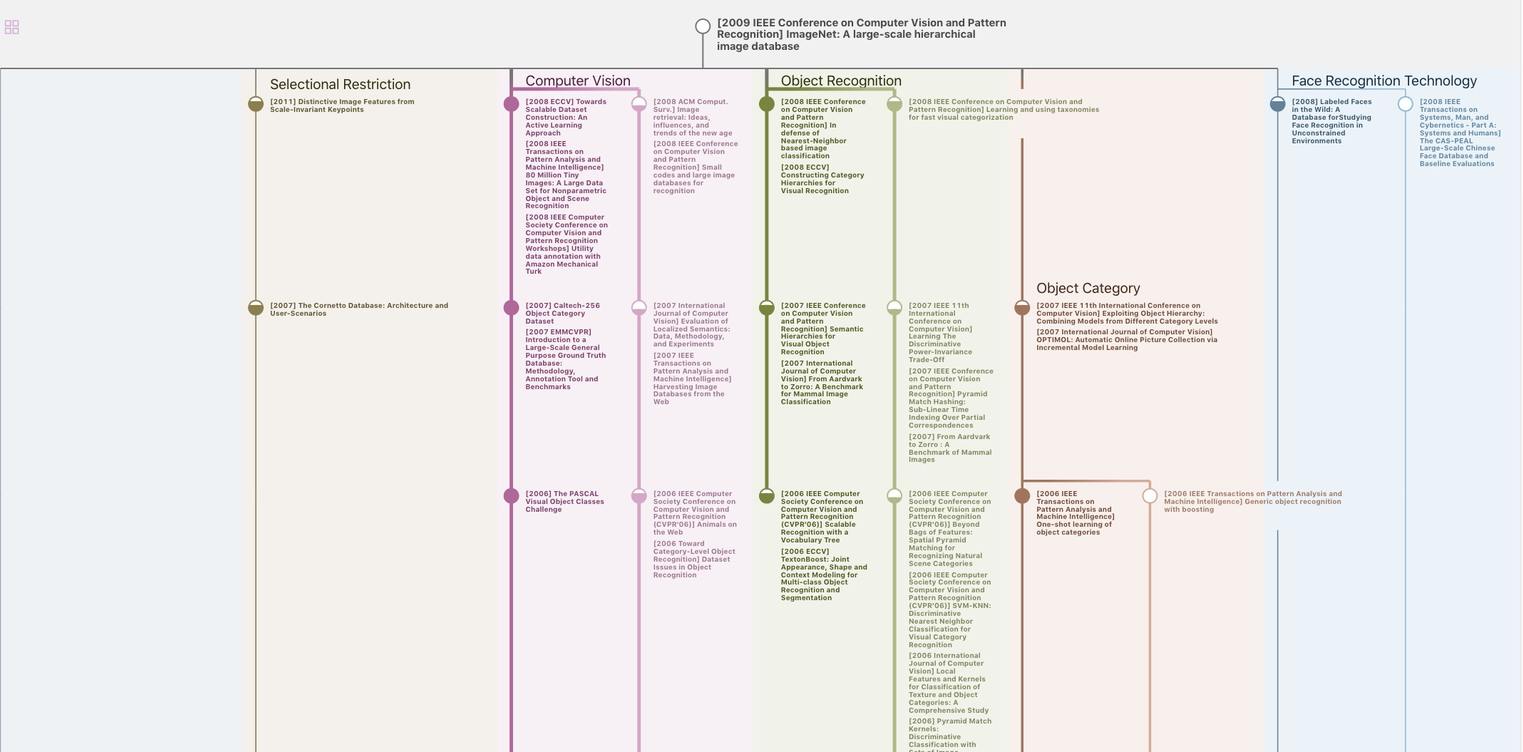
生成溯源树,研究论文发展脉络
Chat Paper
正在生成论文摘要