Efficient Early Warning System in Identifying Enset Bacterial Wilt Disease using Transfer Learning.
IJCNN(2023)
摘要
Enset (Ensete ventricosum (Welw.) Cheesman) is an indigenous Ethiopian crop that sustains the livelihood of more than 20 million people. Enset is used as a crop for human food security, animal feed, and a source of fiber for farmers. Since the past few decades, the production of enset has been severely curbed by bacterial wilt disease. The early detection and prevention of this disease are crucial for enhancing production. Deep learning in plant disease management is becoming an effective way to improve agro-productivity. However, standard convolutional neural network (CNN) models require a large number of parameters and higher computational costs. Efficient CNN models allow users to benefit without having to submit their data to a server for analysis, which is especially useful in parts of the world where internet access is fragile or even inaccessible. In this paper, we proposed a MobileNetV3-Small model which we jointly trained newly added classifier layers with selected final layers of the base model. The proposed model achieved 99.93% of accuracy rate on a test set. The model is trained using a dataset that includes 99 popular enset clones we collected from five different locations in Ethiopia with varying altitudes, climates, and weather conditions.
更多查看译文
关键词
Plant Disease Detection, Enset Bacterial Wilt, Convolutional Neural Network, MobileNetV3, Image Classification
AI 理解论文
溯源树
样例
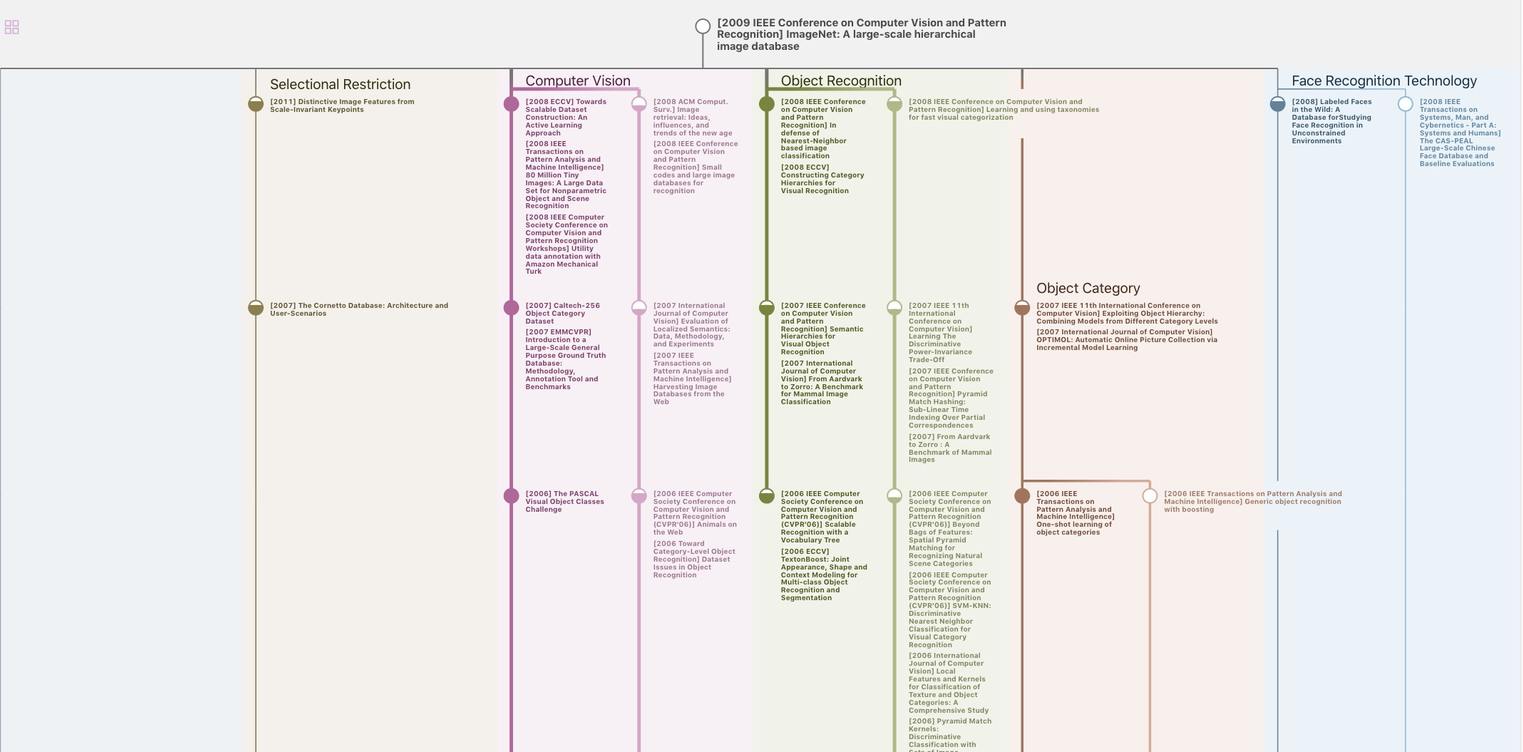
生成溯源树,研究论文发展脉络
Chat Paper
正在生成论文摘要