Data-Locked Knowledge Distillation with Ready-To-Use Substitutes.
IJCNN(2023)
摘要
Knowledge Distillation (KD) becomes a popular way of knowledge transfer from a pre-trained cumbersome teacher model to a compact student model by training the student to mimic the teacher's outputs. However, the original training data of the teacher model, which are supposed to be used for distilling, are often inaccessible during the student training phase due to privacy and intellectual property issues. Several studies reported encouraging results for such kind of data-locked KD problem by collecting substitute inputs via synthesization or seeking ready-to-use samples from publicly available data. This paper aims to take a step further with the more cost-efficient selection-based method. Specifically, we propose a new In-Distribution Detection (IDD) criterion to measure the quality of a candidate sample based on both model confidence and class-relationship consistency. Immediate evaluation of IDD performance with several benchmark image datasets verifies the effectiveness of the designed measurement compared to existing ones, showing its potential for substitute selection from open resources. Experimental results on data-locked KD demonstrate that our approach consistently improves the previous selection-based approach and even outperforms the latest synthesization-based ones given a proper candidate set.
更多查看译文
关键词
in-distribution detection, knowledge distillation, class relationship, pre-trained model, data-locked
AI 理解论文
溯源树
样例
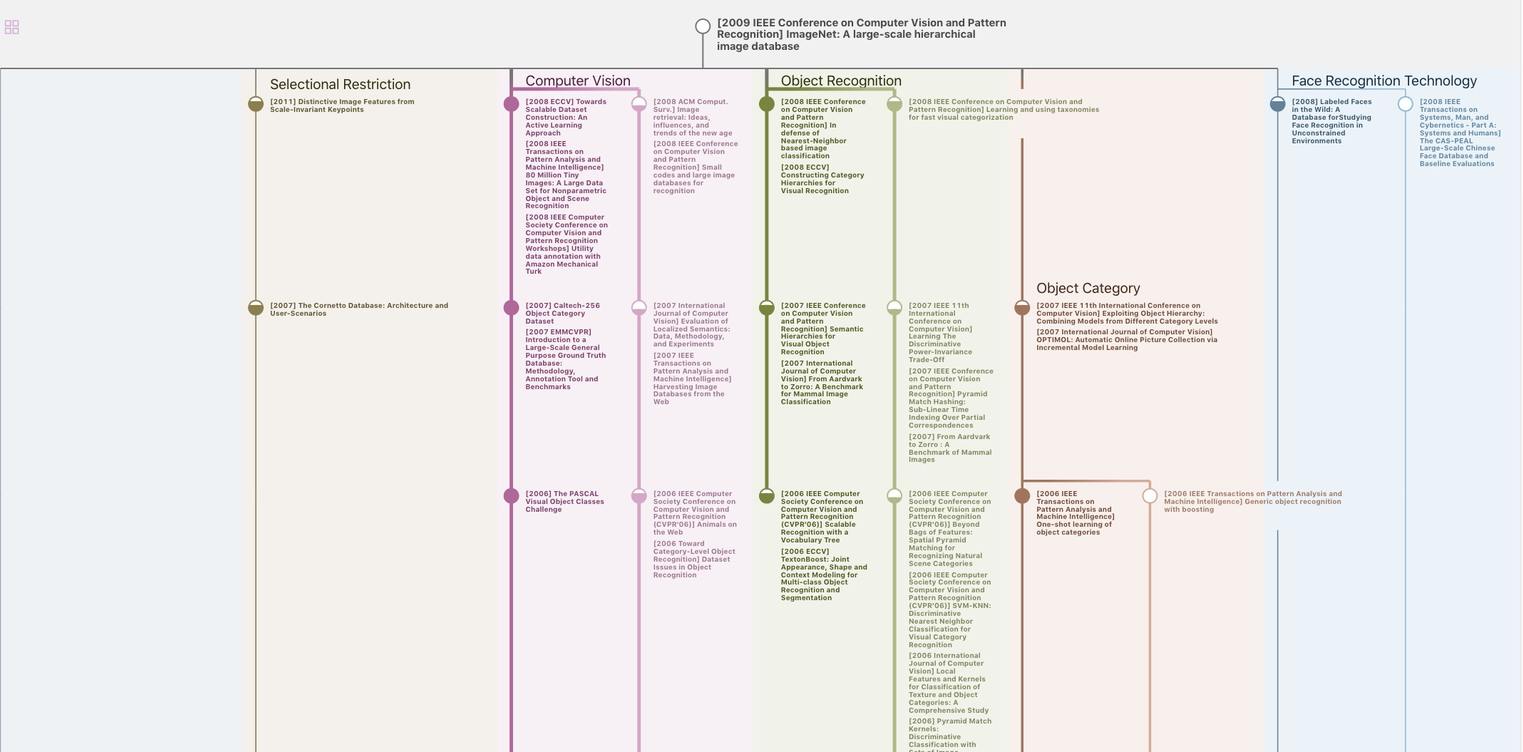
生成溯源树,研究论文发展脉络
Chat Paper
正在生成论文摘要