RAN: A Relation-aware Network for Relation Extraction.
IJCNN(2023)
摘要
Relation extraction is extracting relational facts between target entities from plain text. It has attracted much interest and achieved improvement from research communities recently. However, effectively capturing relational information while ignoring intra-sentence noise from the text remains a challenge. To this end, we propose a novel RAN (Relation-aware Network) model for relation extraction. The basic idea of RAN is to model relation features, providing more accurate guidance for directly capturing relational information in a sentence, and improving the performance of relation extraction. Specifically, we first propose a multi-channel relation representation learning module. This module studies dependencies between the target entity pair in multiple sub-channels to model relation vectors. Then a dual attention mechanism-based relational words selection module is designed to highlight the relational information in the sentence of different dimensions, including semantic- and syntactic-level features. We evaluate our model on two public datasets: SemEval-2010 Task 8 and TACRED. Experiments on two benchmark datasets demonstrate the inspiring performance improvement over state-of-the-art models.
更多查看译文
关键词
dual attention mechanism-based relational words selection module,model relation features,multichannel relation representation learning module,RAN,relation extraction,relation-aware network,relational information,semantic level features,SemEval-2010 Task 8 dataset,TACRED dataset
AI 理解论文
溯源树
样例
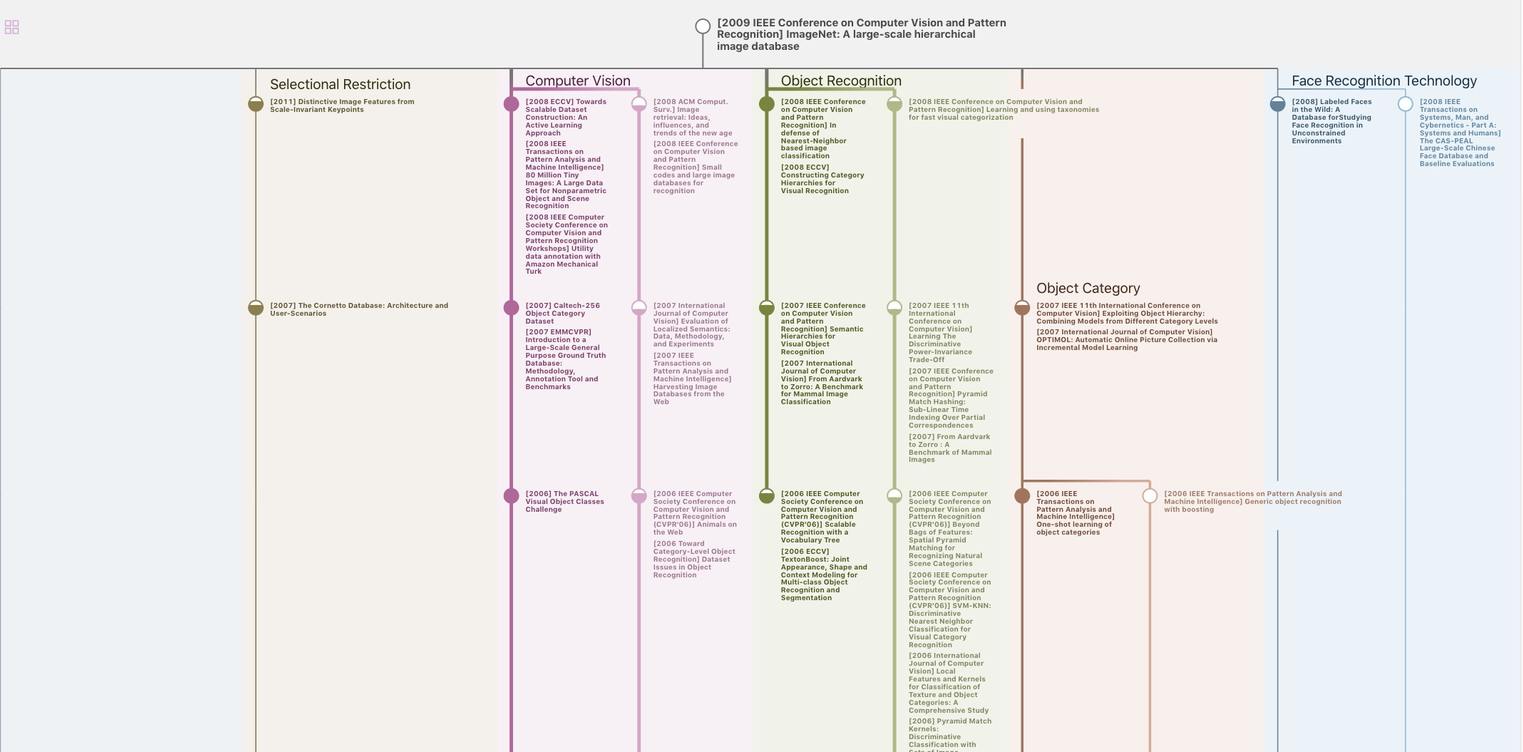
生成溯源树,研究论文发展脉络
Chat Paper
正在生成论文摘要