CDANER: Contrastive Learning with Cross-domain Attention for Few-shot Named Entity Recognition
2023 INTERNATIONAL JOINT CONFERENCE ON NEURAL NETWORKS, IJCNN(2023)
摘要
Few-shot Named Entity Recognition (NER) aims to recognize unseen name entities based on a tiny support set that consists of seen name entities and labels, which is obviously different from traditional supervised NER methods. Contrastive learning has become a popular solution for few-shot NER, which improves the robustness of NER to handle unlabeled entities by learning a similarity metric to measure the semantic similarity between test samples and entity labels. However, existing contrastive learning based NER methods individually learn the word embedding in source and target domains, ignoring connections between entities with the same label and limiting the effectiveness of contrast learning. In this paper, we propose a novel few-shot NER framework that jointly models different domain texts and optimizes a generalized objective of differentiating between words in all stages. The proposed model builds the cross-domain attention layer to enhance the feature representations of words and transfer the entity similarity information from the source domain to the target domain. This significantly reduces the divergence between entities with same label. Experimental results on the largest Few-shot NER dataset show that CDANER significantly outperforms all baseline methods, which verifies the effectiveness and robustness of the proposed model.
更多查看译文
AI 理解论文
溯源树
样例
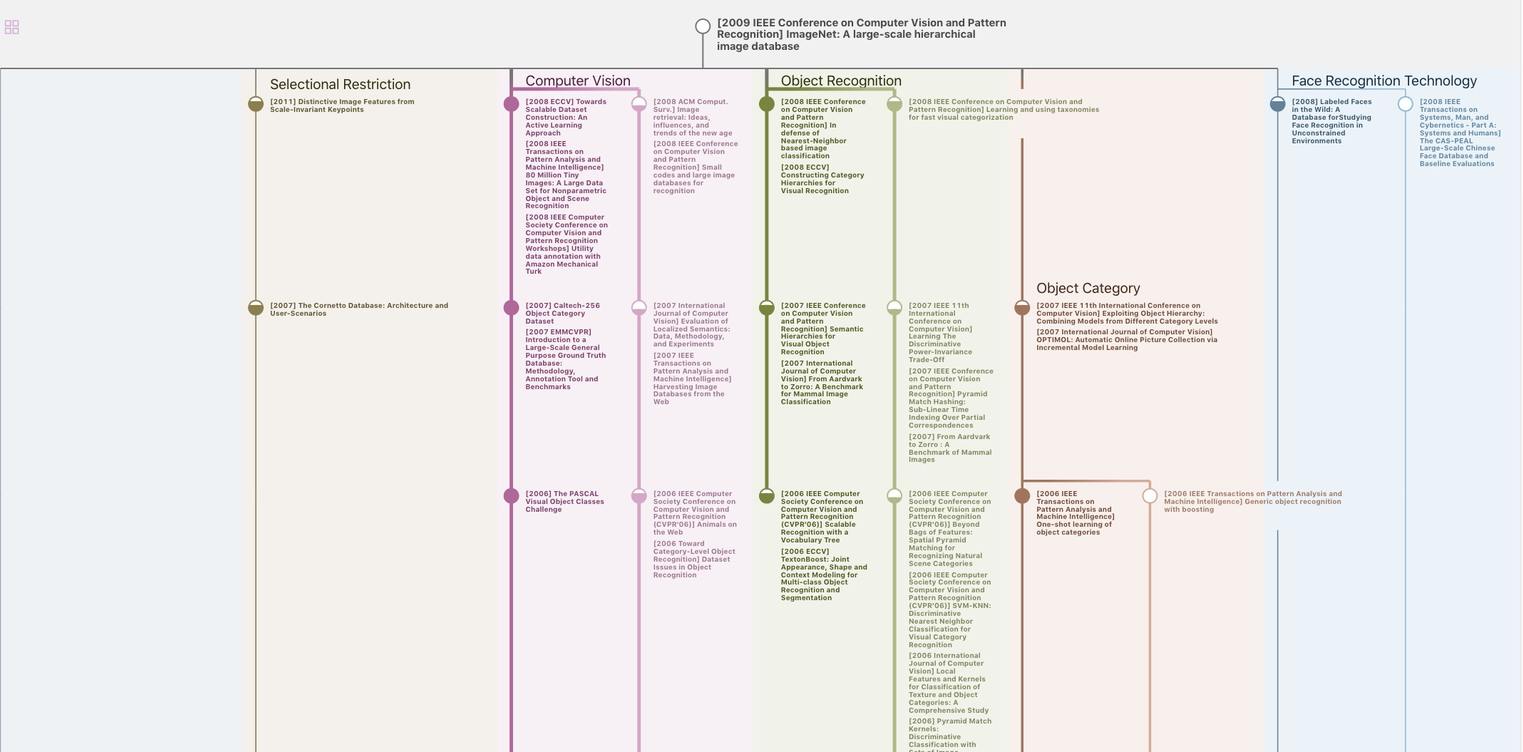
生成溯源树,研究论文发展脉络
Chat Paper
正在生成论文摘要