T3S: Improving Multi-Task Reinforcement Learning with Task-Specific Feature Selector and Scheduler.
IJCNN(2023)
摘要
Multi-task reinforcement learning (MTRL) is a technique to train multiple tasks simultaneously, where previous works usually train a single model to solve different tasks by sharing parameters across various tasks. However, these methods are faced with inter-task interference since what parameters should be shared across tasks is not addressed, dramatically reducing learning efficiency. To solve these problems, we propose a novel MTRL framework called Task-Specific feature Selector and Scheduler (T3S), which consists of two components: a feature selector and a task scheduler. Specifically, the feature selectors employ hypernetworks to construct task-specific soft masks, which can be applied by globally shared representation to construct task-specific features. The task scheduler selects tasks for learning through two metrics, where the selection probability is inversely proportional to task progress (e.g., success rate) and task learning speed. Experimental results show that T3S consistently outperforms the state-of-the-art MTRL algorithms on various robotics manipulation tasks.
更多查看译文
关键词
reinforcement learning, multi-task learning, knowledge sharing, task scheduler
AI 理解论文
溯源树
样例
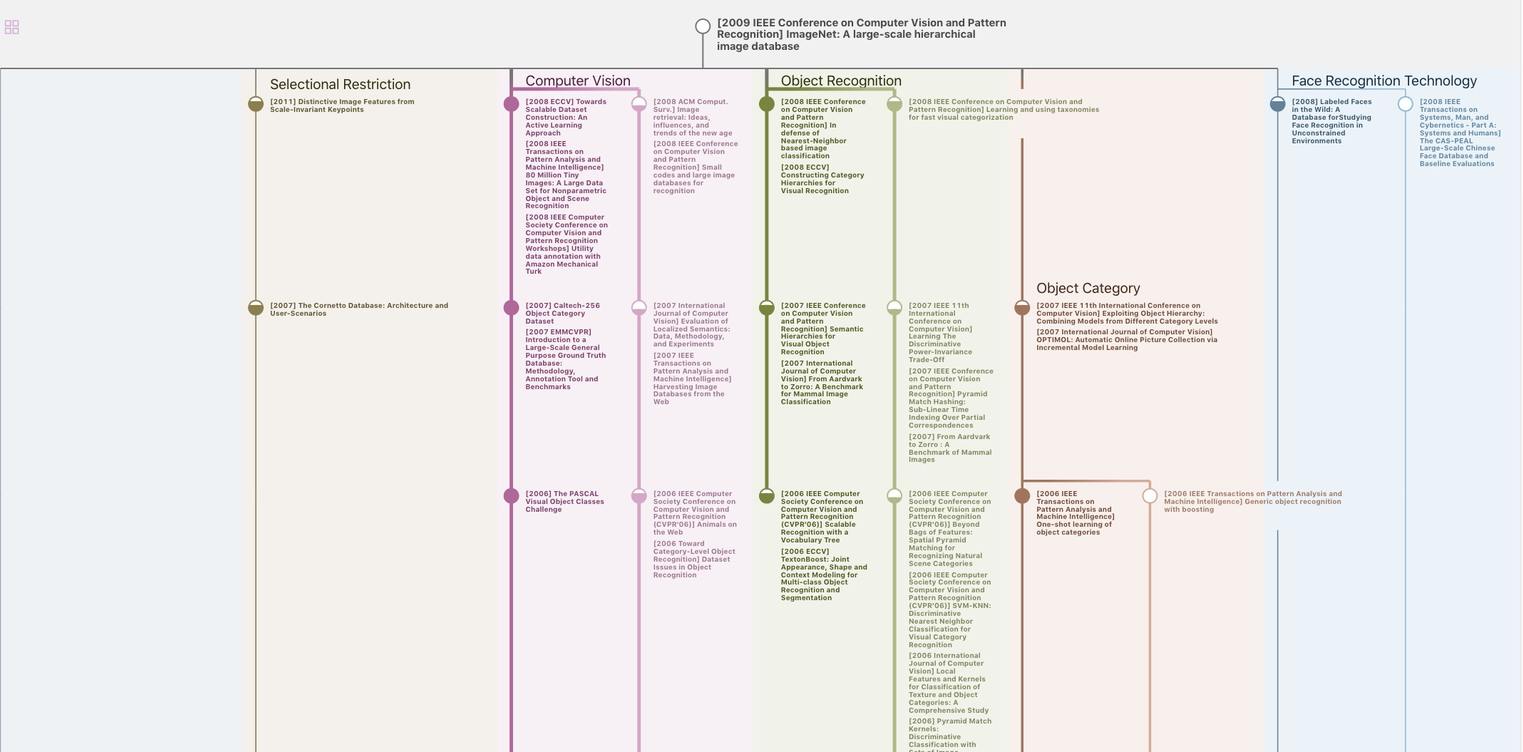
生成溯源树,研究论文发展脉络
Chat Paper
正在生成论文摘要