Semantic-Reconstructed Graph Transformer Network for Event Detection.
IJCNN(2023)
摘要
Event detection (ED) is a key subtask of information extraction to extract key events, such as stock rise and fall and social public opinion, from news or social media. Although current GCN-based event detection methods achieve remarkable success via building graphs with dependency trees, they typically suffer from two challenges: 1) They use sequence models to learn contextual information of sentences, ignoring the long-term dependencies problem of sequence models might learn ineffective information and make it propagate in GCN layers. 2) Most methods do not exploit global dependency label information and grammatical structure information that convey rich linguistic knowledge directly, and only consider local dependency label information. To cope with these challenges, we propose a novel event detection model via semantic-reconstructed graph transformer networks (SRGTNED), which incorporates semantic reconstruction and path information collection methods. Using the semantic reconstruction method, we assign a pruned sequence to each word based on the path information to capture contextual information consistent with sentence semantics. Moreover, to better utilize global dependency label information and grammatical structure information, a Graph Transformer Network (GTN)-based heterogeneous graph embedding framework is introduced to automatically learn path information between important words by converting sentences as heterogeneous graphs. We conduct experiments on the ACE2005 dataset and the Commodity News dataset, and the experimental results demonstrate that our method significantly outperforms 11 state-of-the-art baselines in terms of the F1-score.
更多查看译文
关键词
event detection, semantic reconstruction, path information, heterogeneous graph
AI 理解论文
溯源树
样例
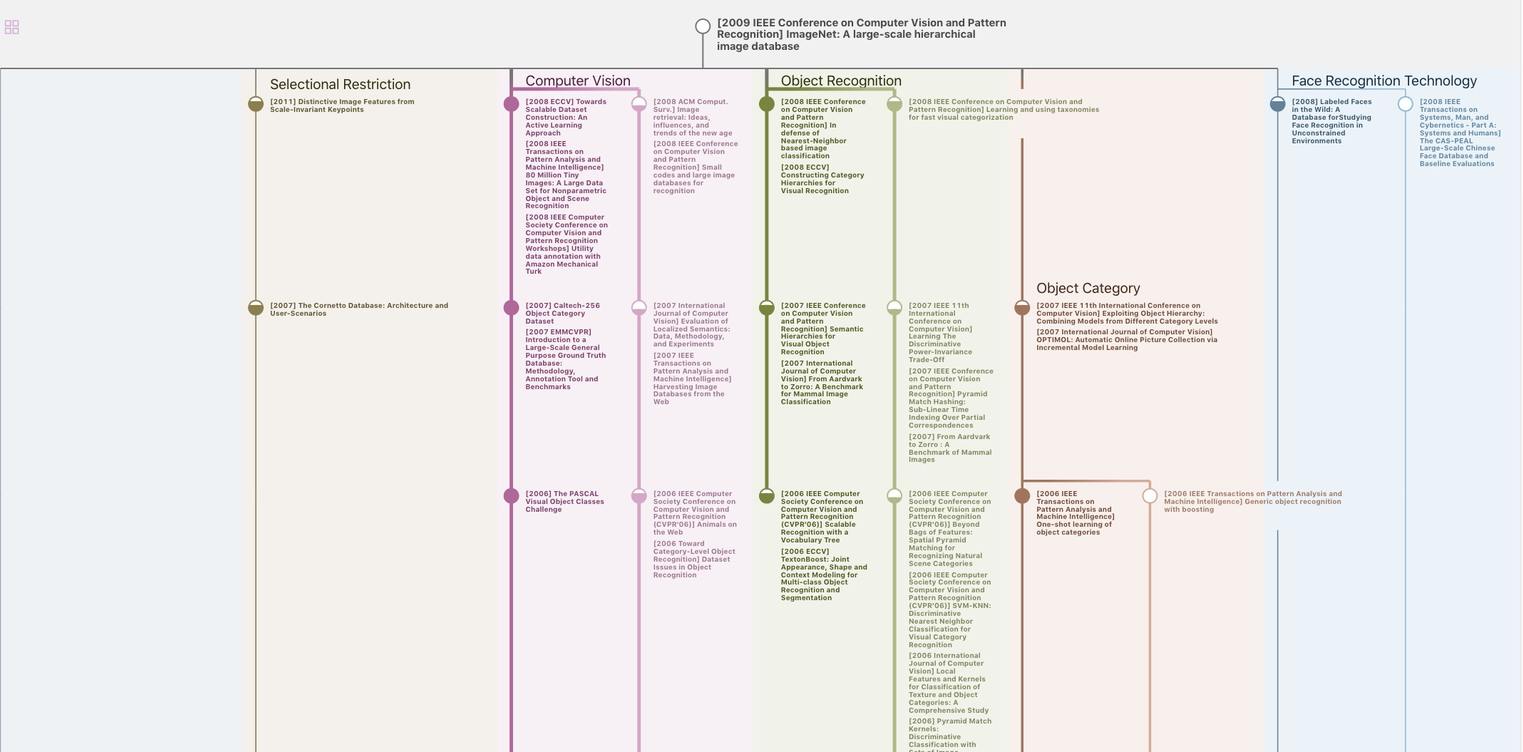
生成溯源树,研究论文发展脉络
Chat Paper
正在生成论文摘要