Improving Knowledge Tracing with Diverse Question Factors.
IJCNN(2023)
摘要
Knowledge Tracing (KT) aims at predicting future students' performance based on their responses to historical questions, which plays a pivotal role in intelligent education. Most of the existing efforts pay more attention to students' dynamically changing knowledge states while neglecting the individualization of questions and difficulty level that differs from question to question. Towards this end, in this paper, we propose a novel Diverse Question Factors-enhanced Knowledge Tracing (DQFKT) method, which sufficiently explores various question factors to generate better prediction. On one hand, two reliable and low-dimensional student-concept connection spaces are established through exploiting the student-question-concept relations and individualization of question, and then fine-grained response representations of questions are obtained according to students' responses. On the other hand, difficulty level with concrete concept for each question is introduced to simulate the complex interactions between students and questions for improving the prediction performance. Extensive experiments on three datasets have demonstrated that the DQFKT approach has more precise prediction of student performance and stronger interpretable power.
更多查看译文
关键词
Intelligent Education,Knowledge Tracing,Question Factors,Response Representation of Question,Difficulty Level of Question
AI 理解论文
溯源树
样例
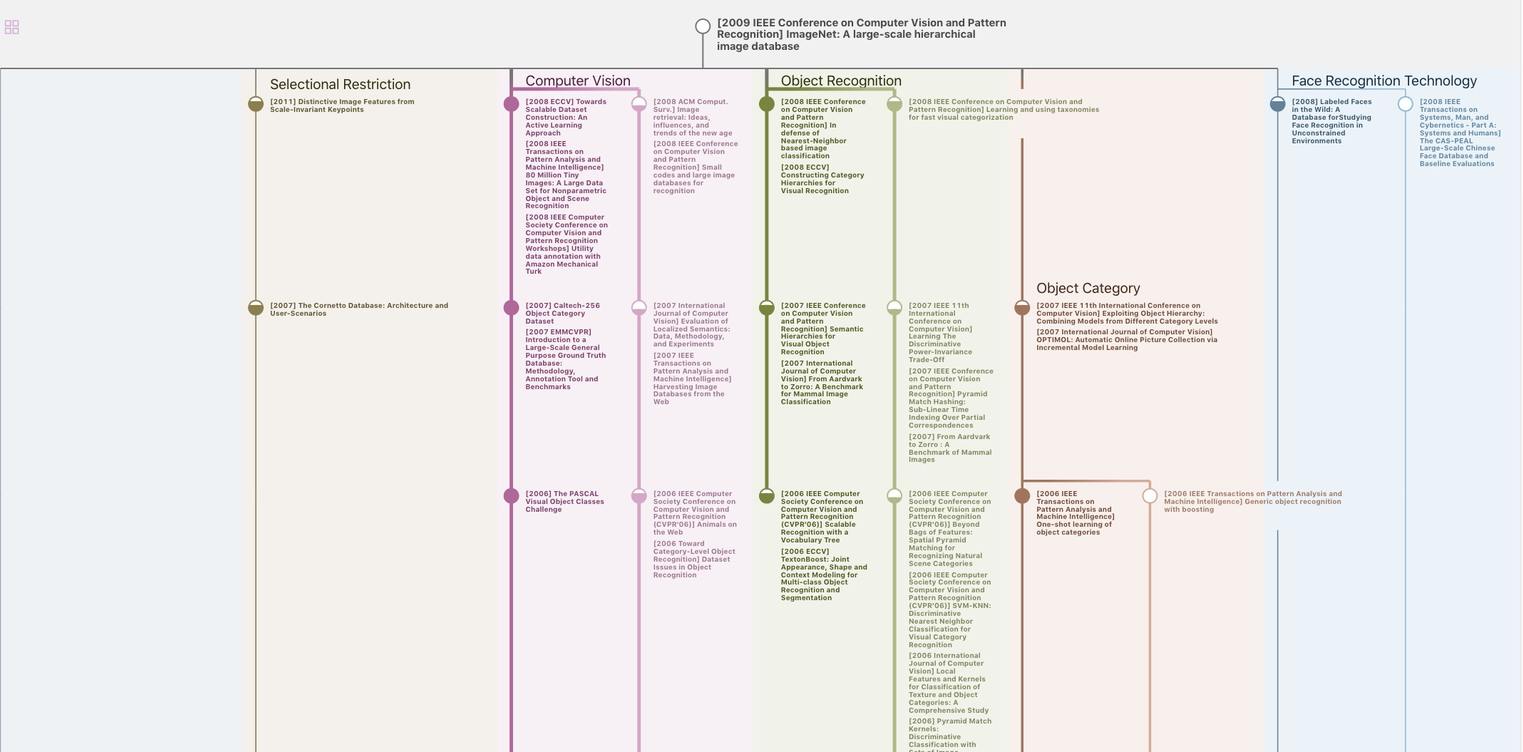
生成溯源树,研究论文发展脉络
Chat Paper
正在生成论文摘要