Time-multiplexed Reservoir Computing with Percolating Networks of Nanoparticles.
IJCNN(2023)
摘要
Percolating Networks of Nanoparticles (PNNs) are under investigation as candidates for physical implementations of reservoir computing (RC). Several promising features have been identified in PNNs, such as brain-like critical dynamics, long-range spatiotemporal correlations and nonlinear I-V characteristics. However, the information processing capability of PNNs remains to be demonstrated. Here we present detailed modelling of PNNs operating in the tunneling regime as delayed-dynamical reservoirs (DDRs). The computational capacity of these reservoirs is successfully demonstrated by their performance in two benchmark tasks: waveform discrimination and tenth-order nonlinear auto-regressive moving average (NARMA10) time series prediction. Furthermore, the interplay between the PNN response time and the delayed feedback is elucidated, providing valuable insight for future DDR design.
更多查看译文
关键词
brain-like critical dynamics,computational capacity,DDR,delayed-dynamical reservoirs,information processing capability,long-range spatiotemporal correlations,NARMA10,percolating networks of nanoparticles,PNN response time,tenth-order nonlinear auto-regressive moving average time series prediction,time-multiplexed reservoir computing,tunneling regime,waveform discrimination
AI 理解论文
溯源树
样例
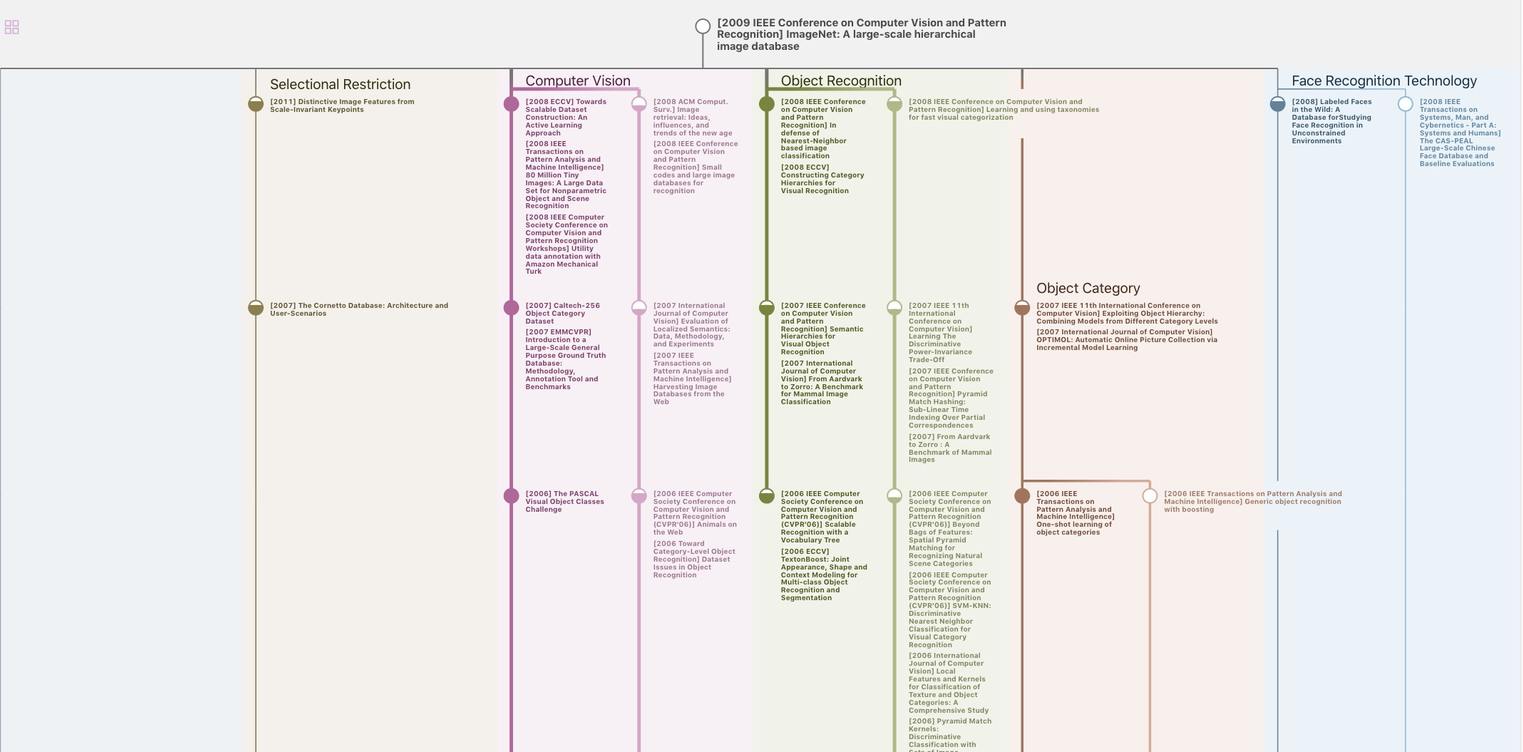
生成溯源树,研究论文发展脉络
Chat Paper
正在生成论文摘要