Stability and Limit Cycles of Fuzzy Inferences in a Recurrent Petri-like Neural Network.
IJCNN(2023)
摘要
This paper proposes a generic architecture of a recurrent fuzzy inferential neural network realized with Petri Nets. The proposed recurrent topology allows revision or updates of fuzzy singleton memberships of inferences, which may lead to limit cycles (sustained periodic oscillations) or stability of fuzzy inferences. Determining stability in such recurrent fuzzy neural network requires adaptation of fuzzy memberships for all propositions mapped at places of the Petri Net in parallel. The network is said to have attained stability, if after k updates of memberships of the propositions, the steady-state values are reached for all the propositions of the network, where k denotes the number of transitions in the Petri net. If fuzzy memberships of the propositions do not converge after k updates, then the network yields sustained oscillations in memberships (called Limit Cycles). Detection of stability or limit cycles in such network requires users to wait for k steps of fuzzy membership updates at all places of the network. To avoid the computational overhead for k updating of memberships in the entire network, this paper makes an attempt to determine the condition of stability or limit cycles using Lyapunov stability theorem before the network is invoked for fuzzy membership updating and inference generation. The results of the analysis envisage that the condition of stability depends on the topological architecture and initial assignment of memberships at the places. The condition derived can be checked to test possible stability in the network. In case the condition for stability is not attainable, the network is expected to have limit cycles, which too can be detected without updating of memberships.
更多查看译文
关键词
Fuzzy membership updating, recurrent topology, Lyapunov stability, Fuzzy inference
AI 理解论文
溯源树
样例
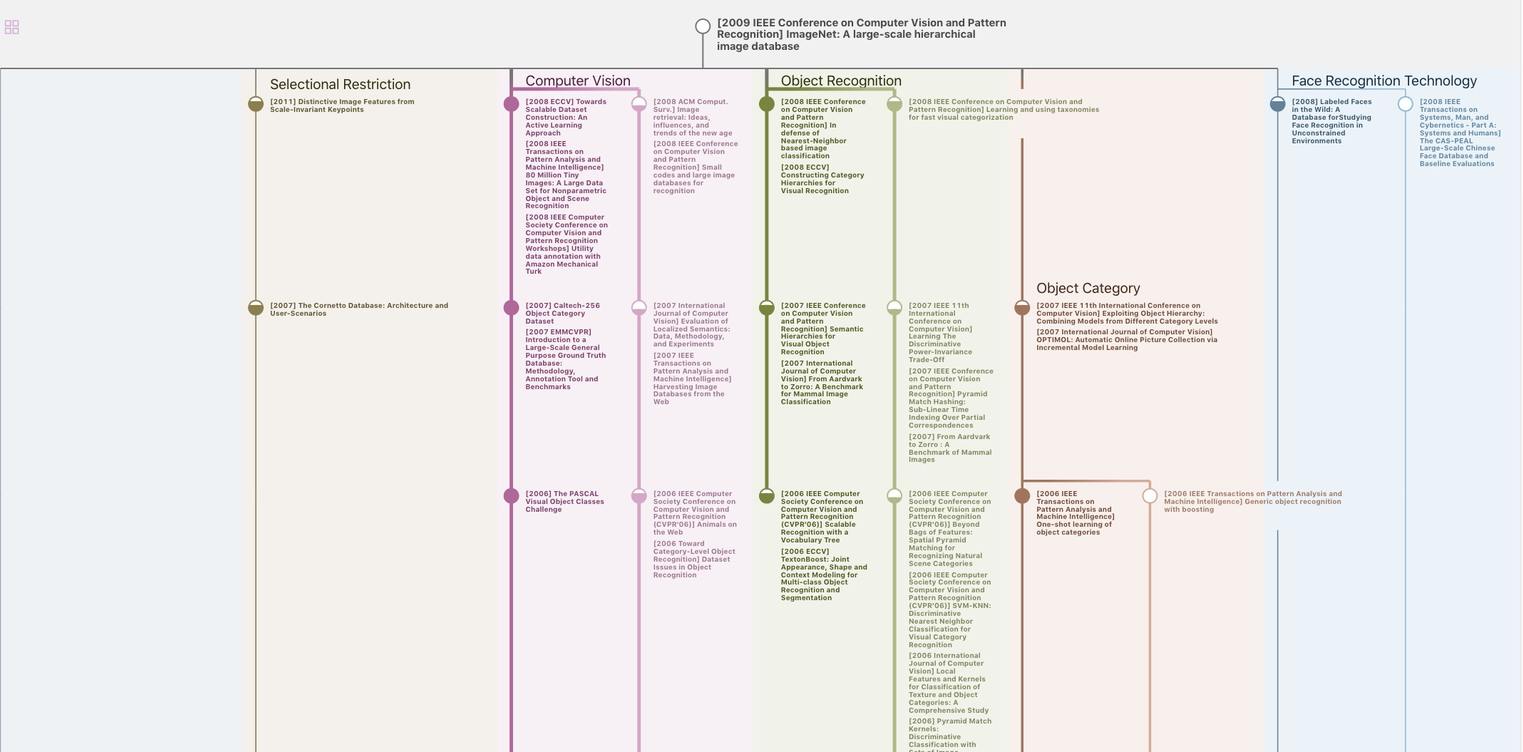
生成溯源树,研究论文发展脉络
Chat Paper
正在生成论文摘要