Evolution Strategies Enhanced Complex Multiagent Coordination.
IJCNN(2023)
摘要
Multi-agent coordination involves both the individual reward and team reward, where the former guides the agent to learn basic skills and the latter measures how well such a team cooperatively completes final tasks. However, in many complex scenarios, these two aspects can be contradictory, due to that one agent excessively pursuing its own profits may suppress the performance of other teammates and lead to the reduction of overall profits. Besides, such dual rewards are generally entangled which make the learning swing between optimizing either the former or latter, which further leads to the sub-optimal and unstable solutions. Moreover, the sparse reward problem commonly encountered in the multi-agent system would further exacerbate this contradiction. In the present work, we address these challenges by proposing CEMARL, a novel framework combining cross-entropy method (CEM) and off-policy multiagent reinforcement learning (MARL). CEM is gradient-free and learns from the whole episode, whereas MARL is gradient-based and learns from the experiences of agents. The core idea behind CEMARL is that it explicitly decomposes the individual reward and team reward, and deals with them through gradient-based learning and gradient-free evolution, respectively. By means of this, it can simultaneously maximize the individual and team reward, and reconciles the contradiction between individual and team as well as the sparse reward problem. CEMARL shows both conciseness in framework and stability in training, and achieves significantly better performances than state-of-the-art baselines on a range of complex tasks.
更多查看译文
关键词
Multi-agent coordination,Reinforcement Learning,Evolution Strategies
AI 理解论文
溯源树
样例
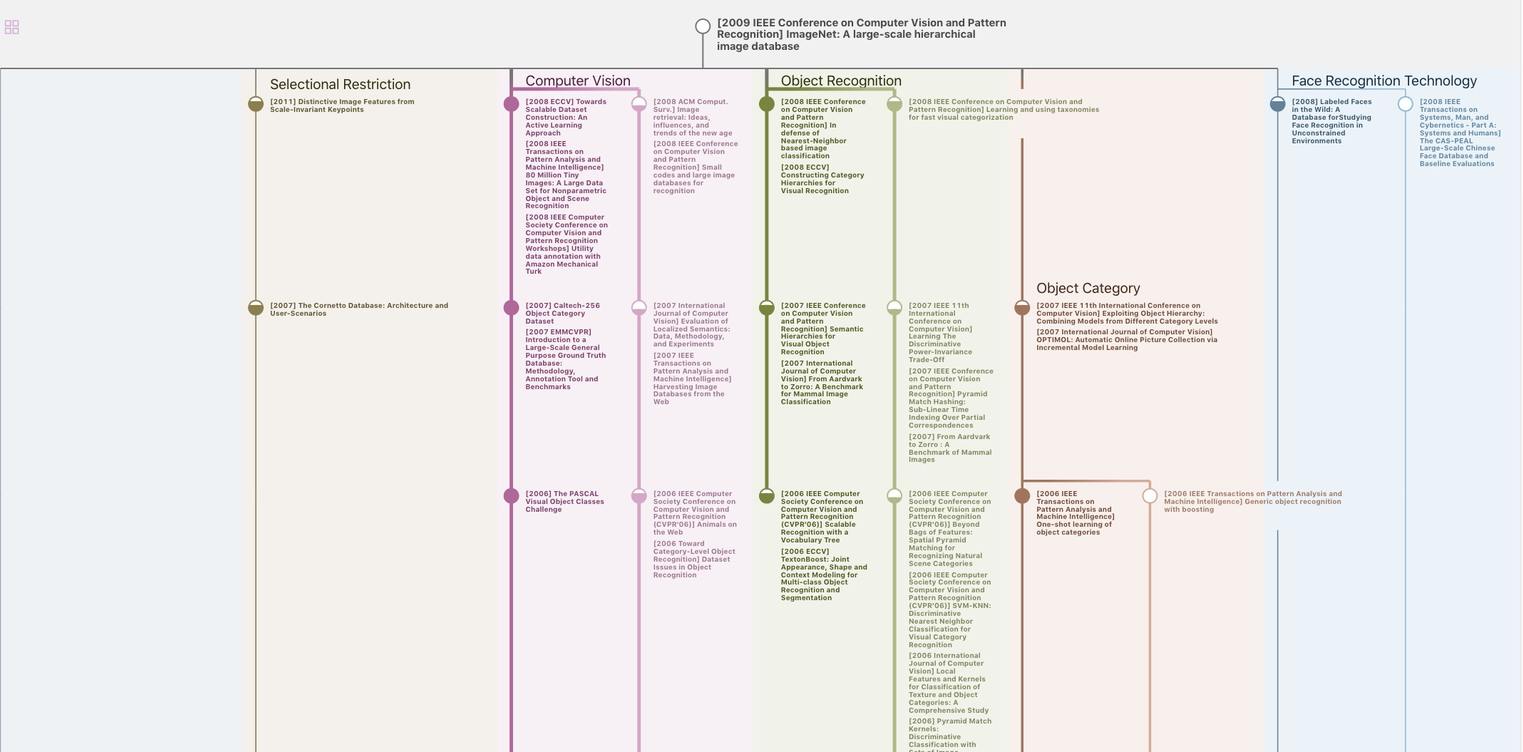
生成溯源树,研究论文发展脉络
Chat Paper
正在生成论文摘要