Joint Node Representation Learning and Clustering for Attributed Graph via Graph Diffusion Convolution.
IJCNN(2023)
摘要
In recent years, the representation learning method based on graph convolution network has made the latest achievements in attributed graph clustering. However, these methods only deal with clustering as a downstream task, and better performance can be achieved if clustering is combined with the node learning representation process. This paper proposes a novel method of graph clustering based on graph diffusion convolution network, which jointly conducts node representation learning and clustering. The graph diffusion strategy is applied on the node attributes to assign the near-by nodes with high weights in the step of feature propagation. The output nodes after diffusion were reconstructed by a linear encoder-decoder, and also could be represented by the clusters. The joint learning is achieved by minimizing both errors of reconstruction and representation. Experiments conducted on three public datasets and three real datasets from Zhengzhou Commodity Exchange demonstrate the effectiveness of the proposed method in the task of node clustering.
更多查看译文
关键词
Graph Diffusion Convolution,Attributed Graph,Clustering
AI 理解论文
溯源树
样例
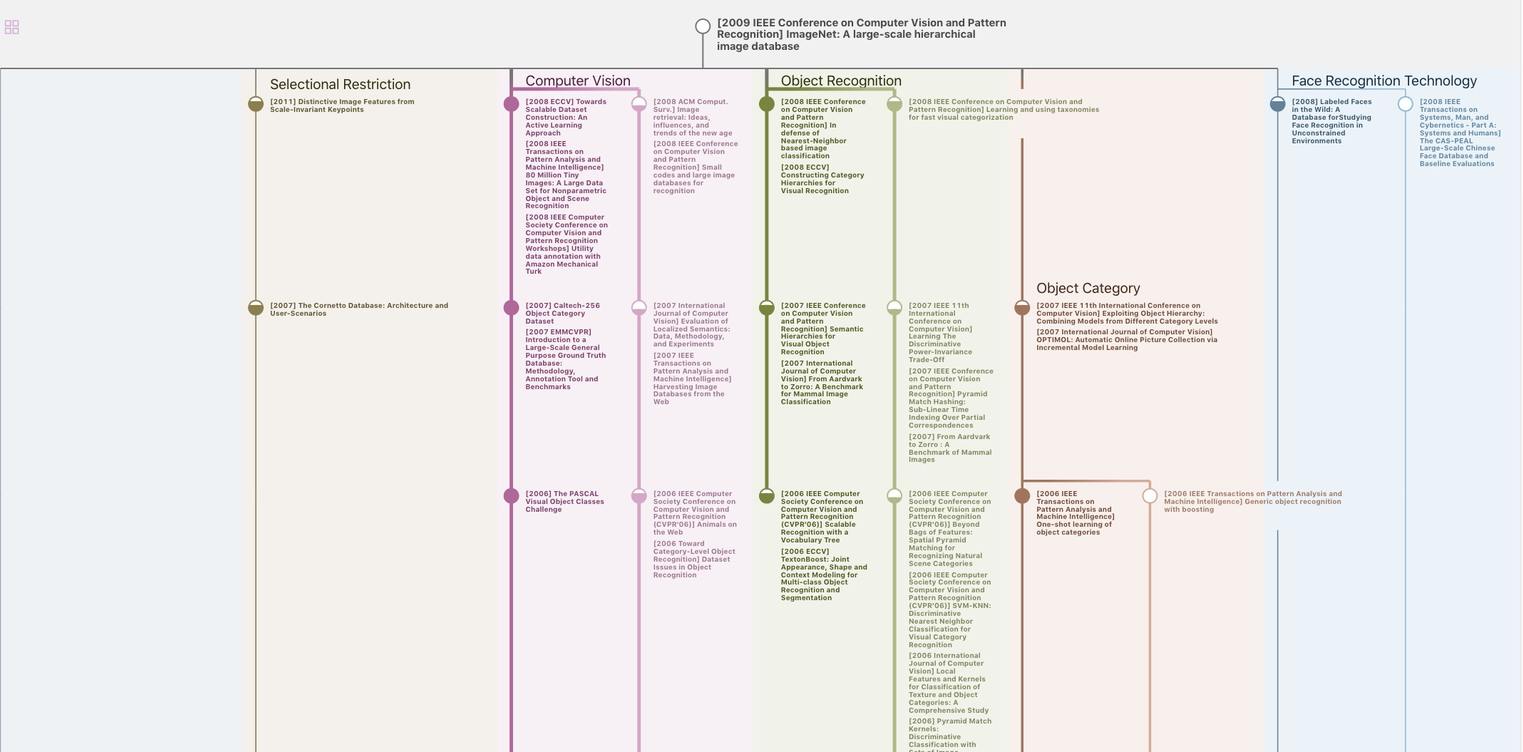
生成溯源树,研究论文发展脉络
Chat Paper
正在生成论文摘要