Iterative Fusion Method Based on Heterogeneous Graph Neural Network for Entity Alignment.
IJCNN(2023)
摘要
Entity alignment aims to link equivalent entities across different knowledge graphs. The knowledge graph contains multiple types of information, such as entities, relations, and attributes. Most existing studies have focused on entity information and ignored other information. Some of the latest approaches introduce information such as relations, but the interactivity could be stronger. Otherwise, it will lead to bias in the extracted features when the central entities have the same or similar neighbours. Thus, the correct entity alignment seed pair cannot be found. In this paper, by introducing heterogeneous graph neural networks to model entities and relations as nodes on the graph simultaneously, the relevant information of relations is fully considered. Furthermore, an iterative fusion method is proposed to enhance the interaction of these two types of semantic nodes to obtain a node representation more suitable for the entity alignment task. The model performs entity alignment after updating the node representations. The experimental results show that all Hits@1 values of this model are greater than 75%, all Hits@10 values are greater than 95%, and all MRR values are greater than 0.83 on the dataset DBP15K.
更多查看译文
关键词
Entity Alignment, Knowledge Graph, Heterogeneous Graph Neural Network, Iterative Fusion
AI 理解论文
溯源树
样例
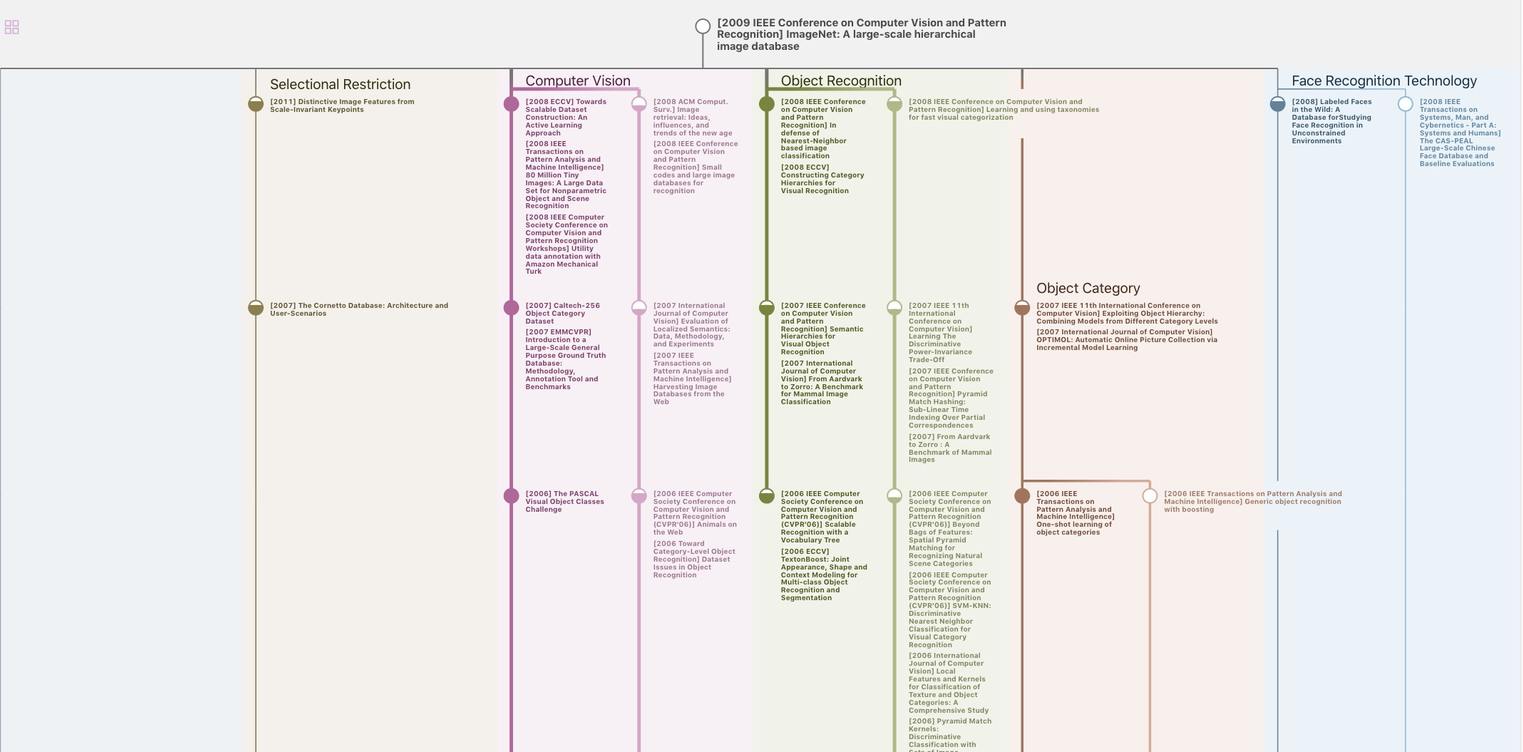
生成溯源树,研究论文发展脉络
Chat Paper
正在生成论文摘要