Incorporating Spatial Sparsity Constraint into Complex IVA of Multi-subject Complex-Valued fMRI Data
2023 INTERNATIONAL JOINT CONFERENCE ON NEURAL NETWORKS, IJCNN(2023)
摘要
Independence and sparsity are proved to be two basic features for spatial activations of functional magnetic resonance imaging (fMRI) data, and have shown efficiency in analysis of magnitude-only fMRI data. Since complex-valued fMRI data contains additional brain activity information beyond magnitude-only fMRI data, we propose to incorporate sparsity constraint into complex independent vector analysis (IVA) to take advantages of the two features in analyzing multi-subject complexvalued fMRI data. Specifically, we propose to improve a complexvalued IVA algorithm named AFIVA (adaptive fixed-point IVA) to add a phase sparsity constraint on spatial maps. Based on the cost function of AFIVA, we further implement the phase sparsity constraint using smoothed L0 norm, and utilize noncircularity of spatial maps as well in the second update of phase sparsity to extract meaningful activations. The results from experimental complex-valued fMRI datasets show that the proposed method yields higher accuracy than AFIVA in terms of true positive rates, confirming the advantage of sparsity in de-noising the independent spatial maps.
更多查看译文
关键词
Independent vector analysis,phase sparsity constraint,complex-valued fMRI data,noncircularity
AI 理解论文
溯源树
样例
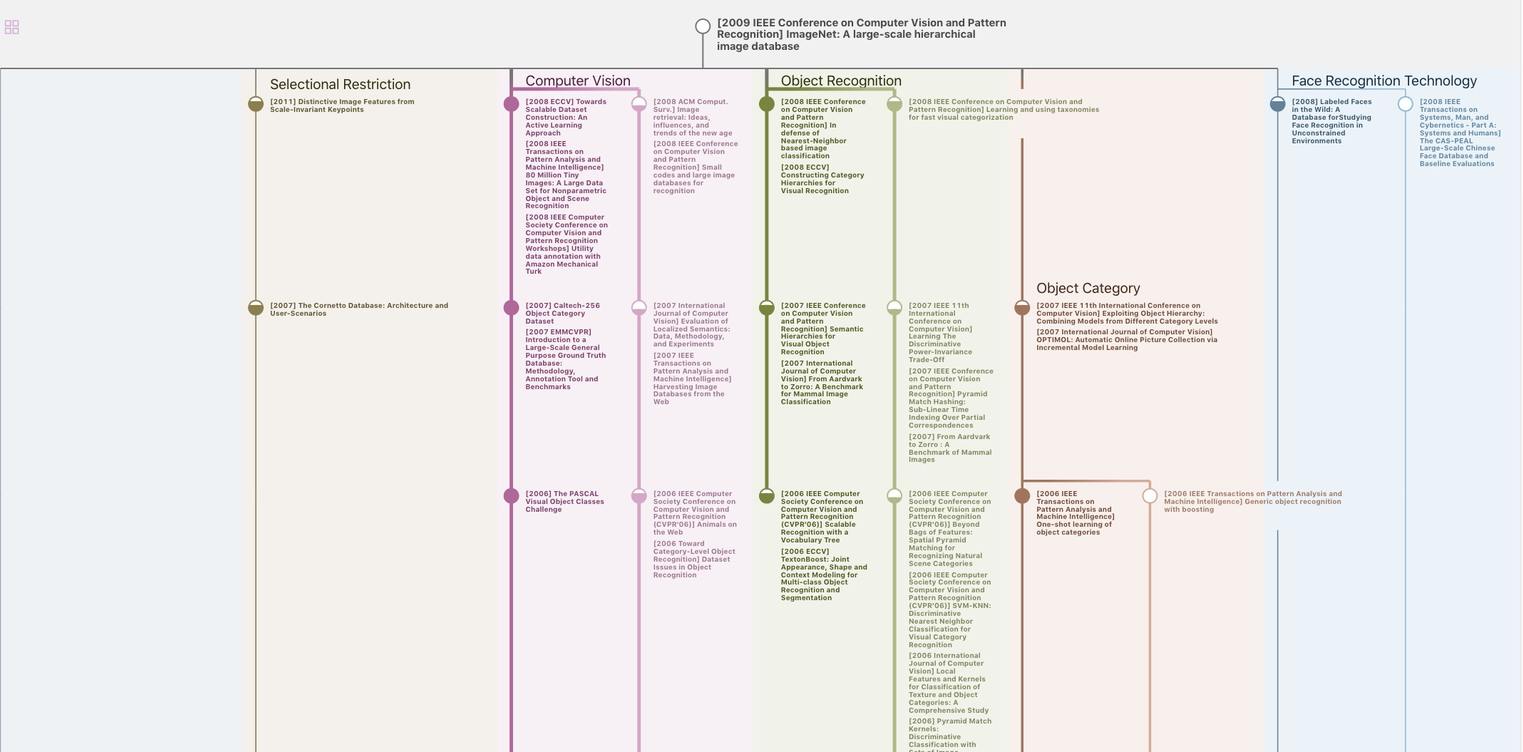
生成溯源树,研究论文发展脉络
Chat Paper
正在生成论文摘要