Evaluating and Comparing Heterogeneous Ensemble Methods for Unsupervised Anomaly Detection.
IJCNN(2023)
摘要
Ensembles are one of the most promising research directions for unsupervised anomaly detection. But combining many different models into such an ensemble requires good combination procedures that are able to combine the strengths of many different submodels. To find, evaluate and understand these procedures, we create the biggest experiment to date, including multiple orders of magnitude more ensembles than each of our competitors. Using this high number of comparisons, we also study the effect different normalization methods have on the combination procedure and extract conditional performances of individual models. We use this, to develop a simple set of best practices to create good and reliable anomaly detection ensembles.
更多查看译文
关键词
anomaly detection, ensembles, unsupervised machine learning
AI 理解论文
溯源树
样例
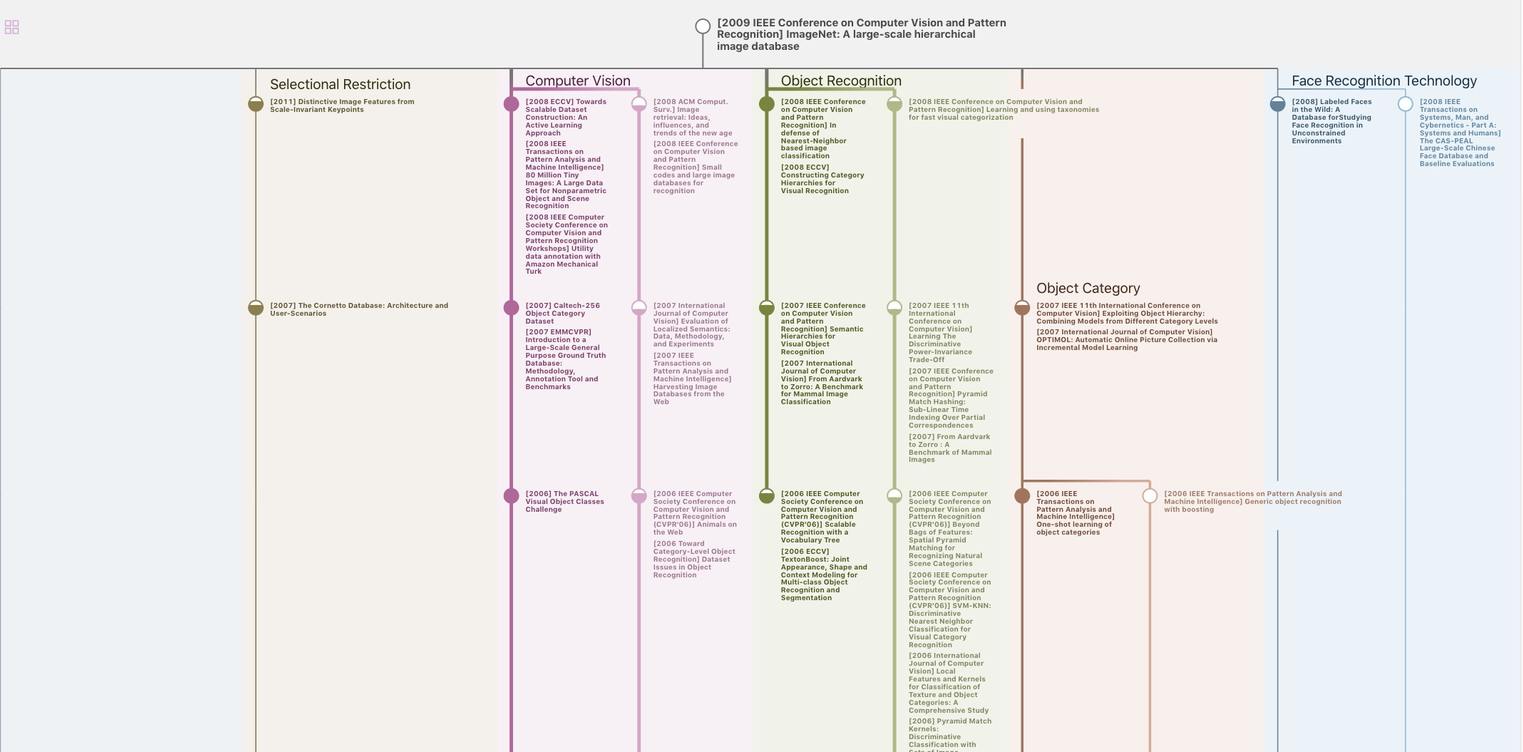
生成溯源树,研究论文发展脉络
Chat Paper
正在生成论文摘要